Adaptive Synapse Adjustment for Multivariate Cortical Learning Algorithm.
SCIS/ISIS(2022)
摘要
This paper proposes an adaptive synapse adjustment method in the cortical learning algorithm (CLA), which simultaneously predicts multiple time-series data. The cortical learning algorithm is a promising time-series data prediction algorithm. A way to predict multiple time-series data simultaneously is to prepare multiple CLA predictors. Although one CLA predictor handles one time-series data, CLA predictors are associated with each other by using synapses. However, the synapse relation between multiple CLA predictors harms the multivariate prediction when the multiple time-series data are lowly related. The proposed method evaluates a partial prediction accuracy for each synapse segment. The proposed method adaptively adds and deletes synapses based on the calculated partial prediction accuracy. This suppresses the synapses crossing multiple CLA predictors, which harms the simultaneous predictions of multiple time-series data. Experiments using artificial and real-world meteorological data show that the proposed method achieves higher prediction accuracy than the conventional method by suppressing the harmful effects caused by synapses crossing multiple predictors.
更多查看译文
关键词
cortical learning algorithm, multivariate time-series prediction, adaptive synapse adjustment
AI 理解论文
溯源树
样例
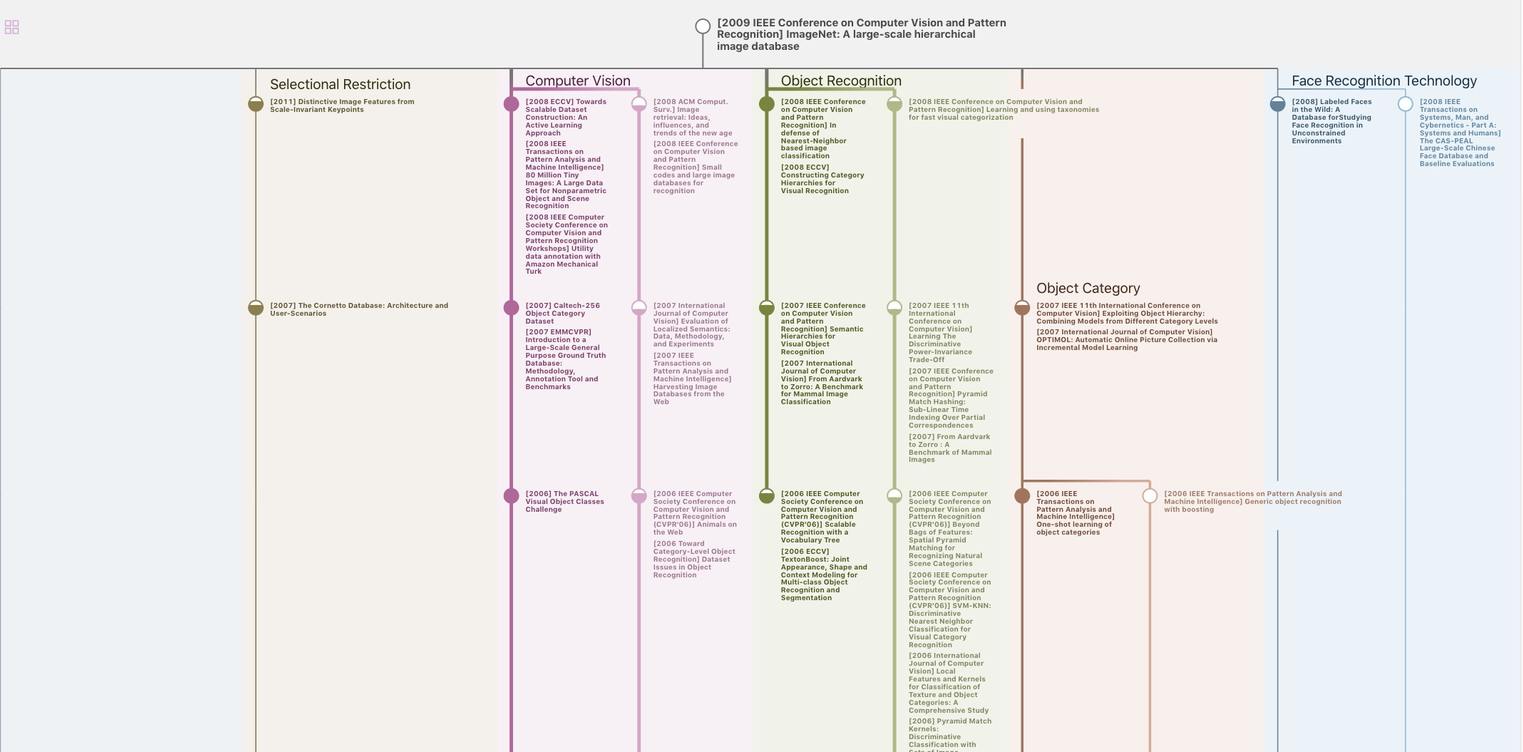
生成溯源树,研究论文发展脉络
Chat Paper
正在生成论文摘要