A fault-tolerant workflow scheduling method on deep reinforcement learning-based in edge environment.
ICNSC(2022)
摘要
Edge computing emerges recently and shows promising solutions to support and boost applications for the Internet of Things (IoT). Nevertheless, by considering a distributed edge server system consisting of multi-users with time-variability and mobility, which may cause applications to be stopped due to resource faults or link failures. We present here a method that directly aims to make the execution of workflows more reliable and efficient. The proposed and designed a fault-tolerant method first analyzed the allocation of a dependency-based task, then tolerates task failure on edge nodes using the primary-backup (PB) strategy, and finally applies state-of-the-art Deep Q Network (DQN) algorithm to obtain the optimise task scheduling scheme. To the end, we conduct a simulative case study on real datasets and compare ours with other fault-tolerant methods. The result shows that our approach improves the reliability of workflow execution in edge environment.
更多查看译文
关键词
Edge Computing,Fault Tolerance,Deep Reinforcement Learning,Workflow Scheduling
AI 理解论文
溯源树
样例
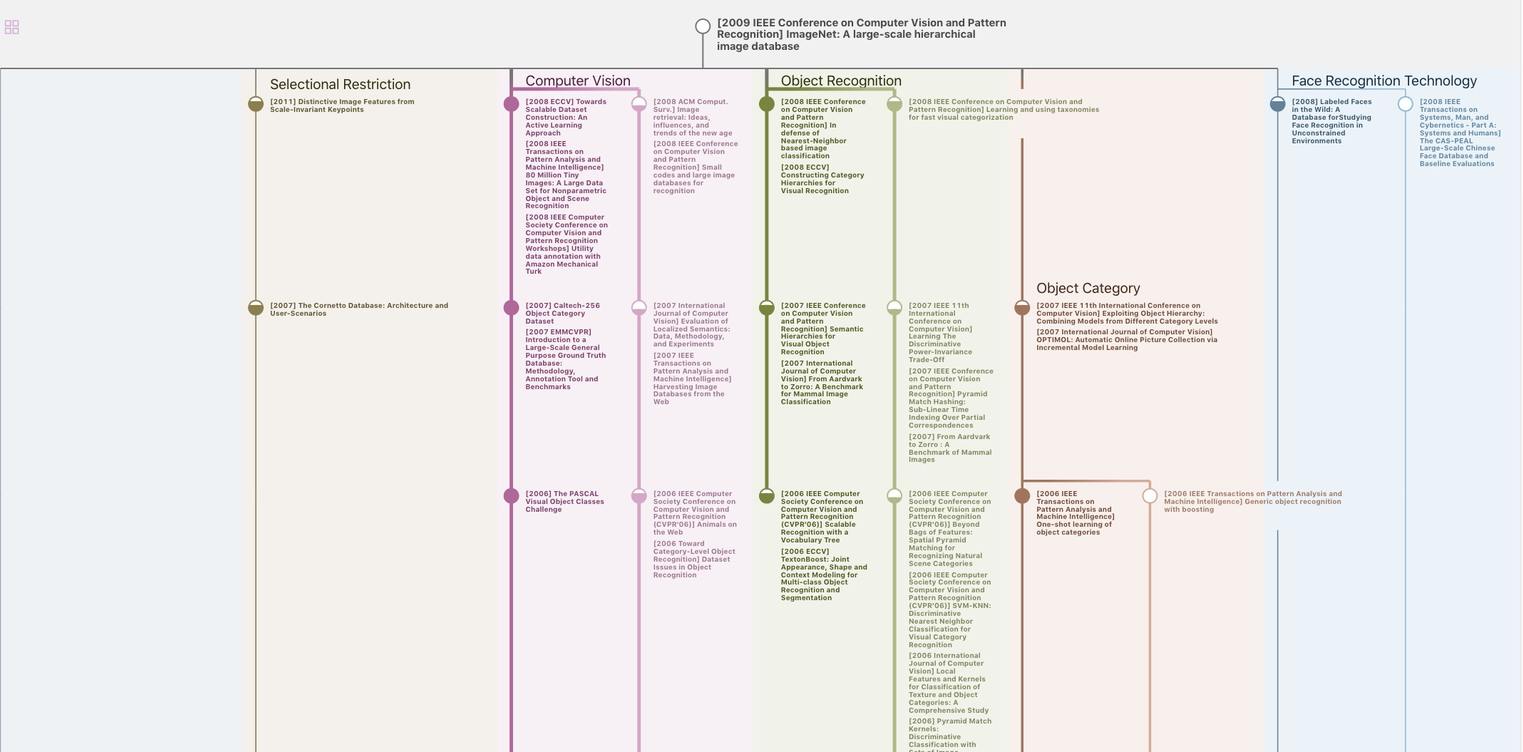
生成溯源树,研究论文发展脉络
Chat Paper
正在生成论文摘要