MACNet: Multi-Attention and Context Network for Polyp Segmentation.
APWeb/WAIM (2)(2022)
摘要
Colorectal cancer (CRC) is the third most common cancer worldwide. Colonoscopy is an effective technique for detecting colorectal polyps, which are closely associated with colorectal cancer. In clinical practice, segmenting polyps from colonoscopy images is of great significance, as it provides valuable information for diagnosis and surgery. Many networks have demonstrated better segmentation results. However, achieving accurate polyp segmentation remains a challenge due to the diverse in size, shape, texture and color of polyps. This paper proposes a Multi-Attention and Context Network (MACNet), which simulates the process of determining the segmentation region by clinical experts, incorporating the Balancing Attention Module (BAM), Non-local Information Statistical Attention module (Non-local), Position Rectify Module (PRM) and Focus Module (FM). BAM and PRM learn to adjust the distribution of attention in the feature map from six different perspectives: polyp region, surrounding mucosa, boundaries, channel axial, horizontal axial and vertical axial of the feature map. Non-local captures the connections between any two pixels in the feature map to supplement long-distance global dependence. FM uses context information of different scales to reason and refine the ambiguous regions in segmentation results and then achieves more accurate polyp segmentation. We evaluate the effectiveness of our network with six evaluation metrics on five polyp datasets, and it can be seen from results that our MACNet can achieve more accurate segmentation in general.
更多查看译文
关键词
Polyp segmentation, Semantic segmentation, Attention, Deep learning, Coloscopy
AI 理解论文
溯源树
样例
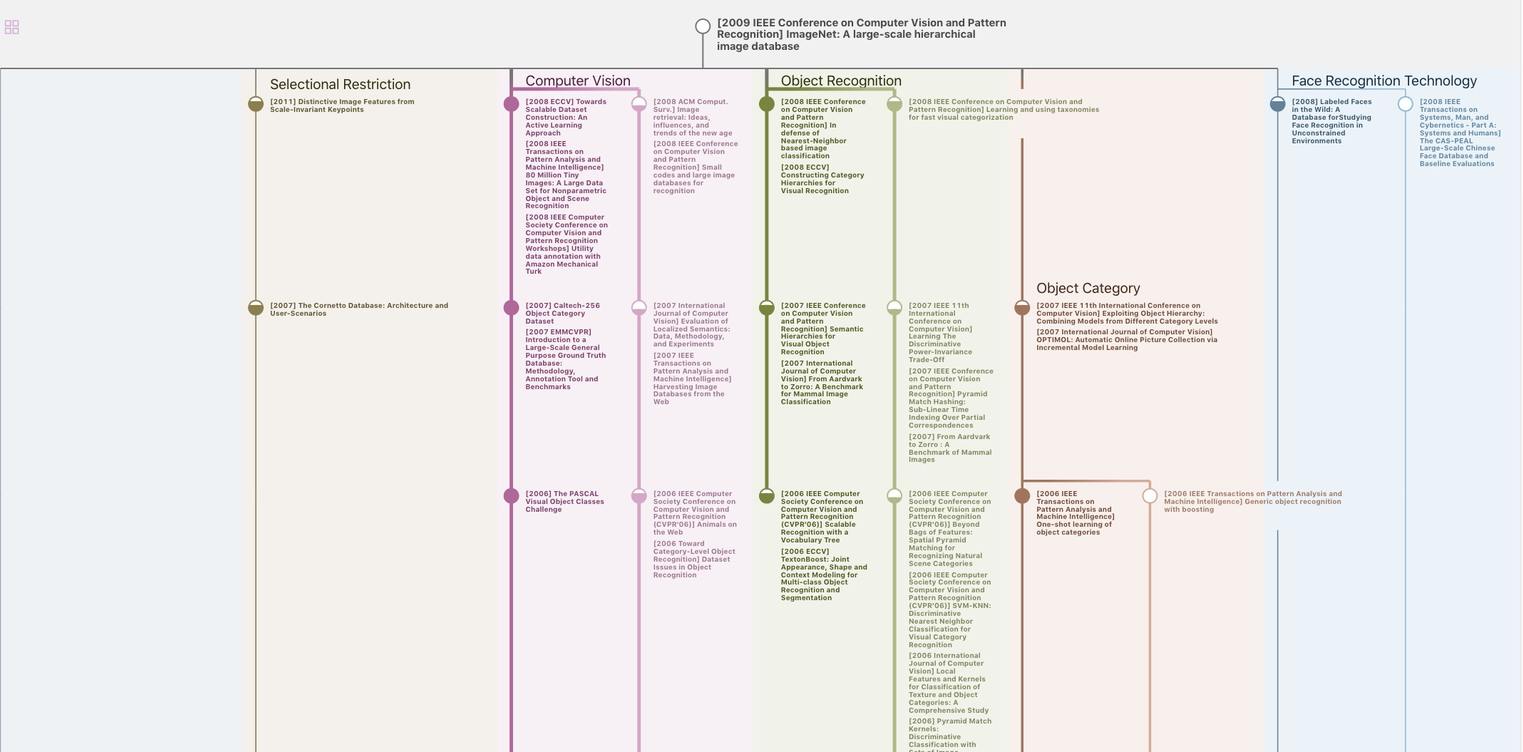
生成溯源树,研究论文发展脉络
Chat Paper
正在生成论文摘要