Insights from Deep Learning in Feature Extraction for Non-supervised Multi-species Identification in Soundscapes.
IBERAMIA(2022)
摘要
Biodiversity monitoring has taken a relevant role in conservation management plans, where several methodologies have been proposed to assess biological information of landscapes. Recently, soundscape studies have allowed biodiversity monitoring by compiling all the acoustic activity present in landscapes in audio recordings. Automatic species detection methods have shown to be a practical tool for biodiversity monitoring, providing insight into the acoustic behavior of species. Generally, the proposed methodologies for species identification have four main stages: signal pre-processing, segmentation, feature extraction, and classification. Most proposals use supervised methods for species identification and only perform for a single taxon. In species identification applications, performance depends on extracting representative species features. We present a feature extraction analysis for multi-species identification in soundscapes using unsupervised learning methods. Linear frequency cepstral coefficients (LFCC), variational autoencoders (VAE), and the KiwiNet architecture, which is a convolutional neural network (CNN) based on VGG19, were evaluated as feature extractors. LFCC is a frequency-based method, while VAE and KiwiNet belong to the deep learning area. In ecoacoustic applications, frequency-based methods are the most widely used. Finally, features were tested by a clustering algorithm that allows species recognition from different taxa. The unsupervised approaches performed multi-species identification between 78%-95%.
更多查看译文
关键词
deep learning,feature extraction,non-supervised,multi-species
AI 理解论文
溯源树
样例
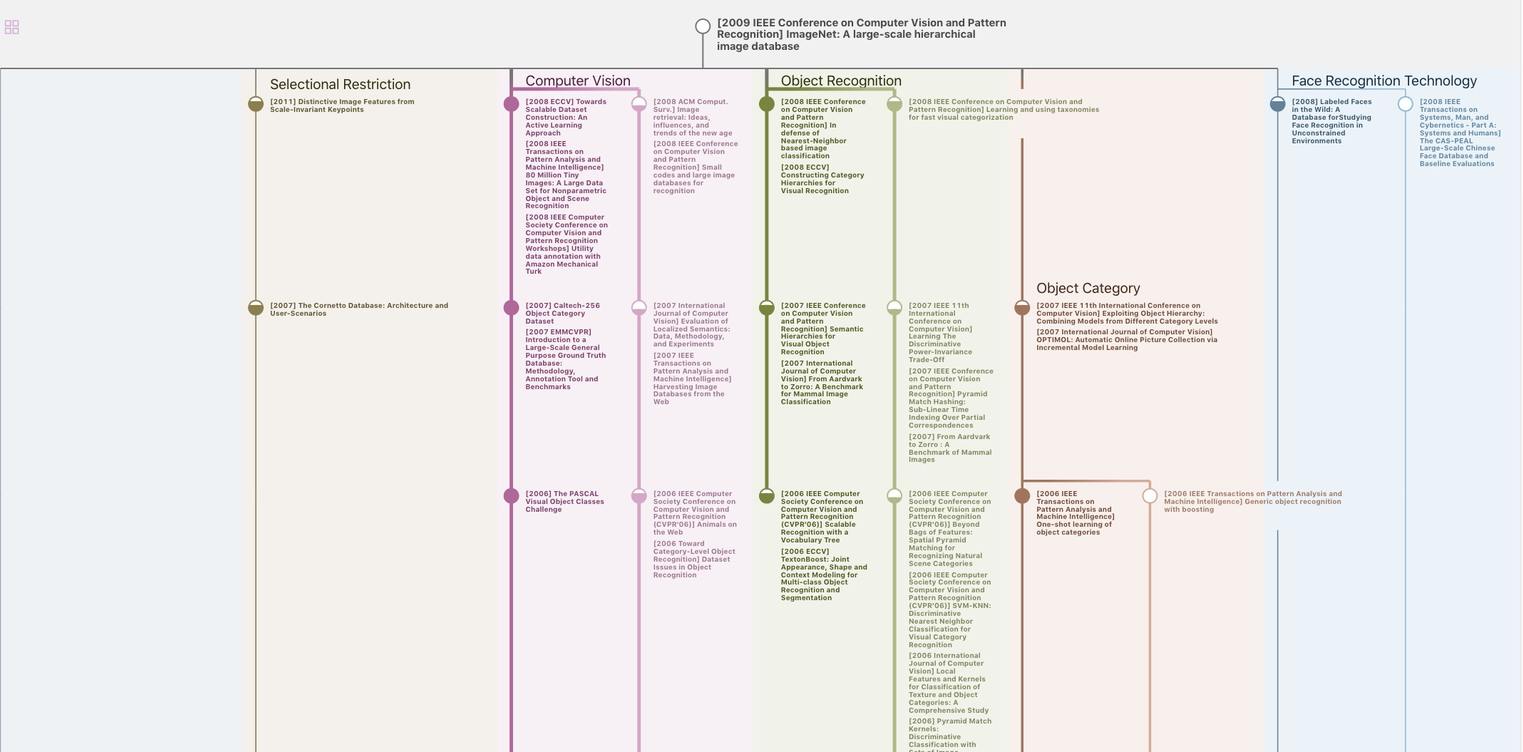
生成溯源树,研究论文发展脉络
Chat Paper
正在生成论文摘要