HideSeeker: Uncover the Hidden Gems in Obfuscated Images
SenSys(2022)
摘要
Obfuscation technologies have been well established for on-device image privacy protection, including pixelization, blurring, scribbling, sticker-covering, and inpainting. Despite their remarkable resistance to human observation, recent studies find that some of them are vulnerable to attacks by neural network-based recognition methods. In this work, we reveal the risk of privacy re-disclosure post image protection. Given an obfuscation-protected image, the privacy information includes 1) where the obfuscated region is and 2) what the hidden privacy-related objects are. Thus we focus on uncovering categories of privacy-related objects to evaluate the effectiveness of obfuscation technologies. Under severe obfuscation, unfortunately, even powerful object recognition models can hardly infer hidden privacy information. Inspired by the human observation process, we carefully craft a scheme HideSeeker, composed of feature-extraction, relation-graph-learning, and object-inference, to explore the contextual information of obfuscated regions and their relationships. HideSeeker can efficiently and effectively uncover the categories of hidden private objects in obfuscated images. We conduct comprehensive evaluations over two datasets containing 14206 images in total: laboratory-generated and publicly available obfuscated images. Our results demonstrate that HideSeeker successfully uncovers privacy-related objects with inference accuracy of up to 82.17%, and 77.72% on average no matter which obfuscation was applied, while the SOTA method can achieve an average accuracy of 42.3%. For images protected by inpainting, accuracy is improved from 24.67% to 78.16%.
更多查看译文
关键词
On-device privacy evaluation, privacy object inference, image obfuscation, relation graph
AI 理解论文
溯源树
样例
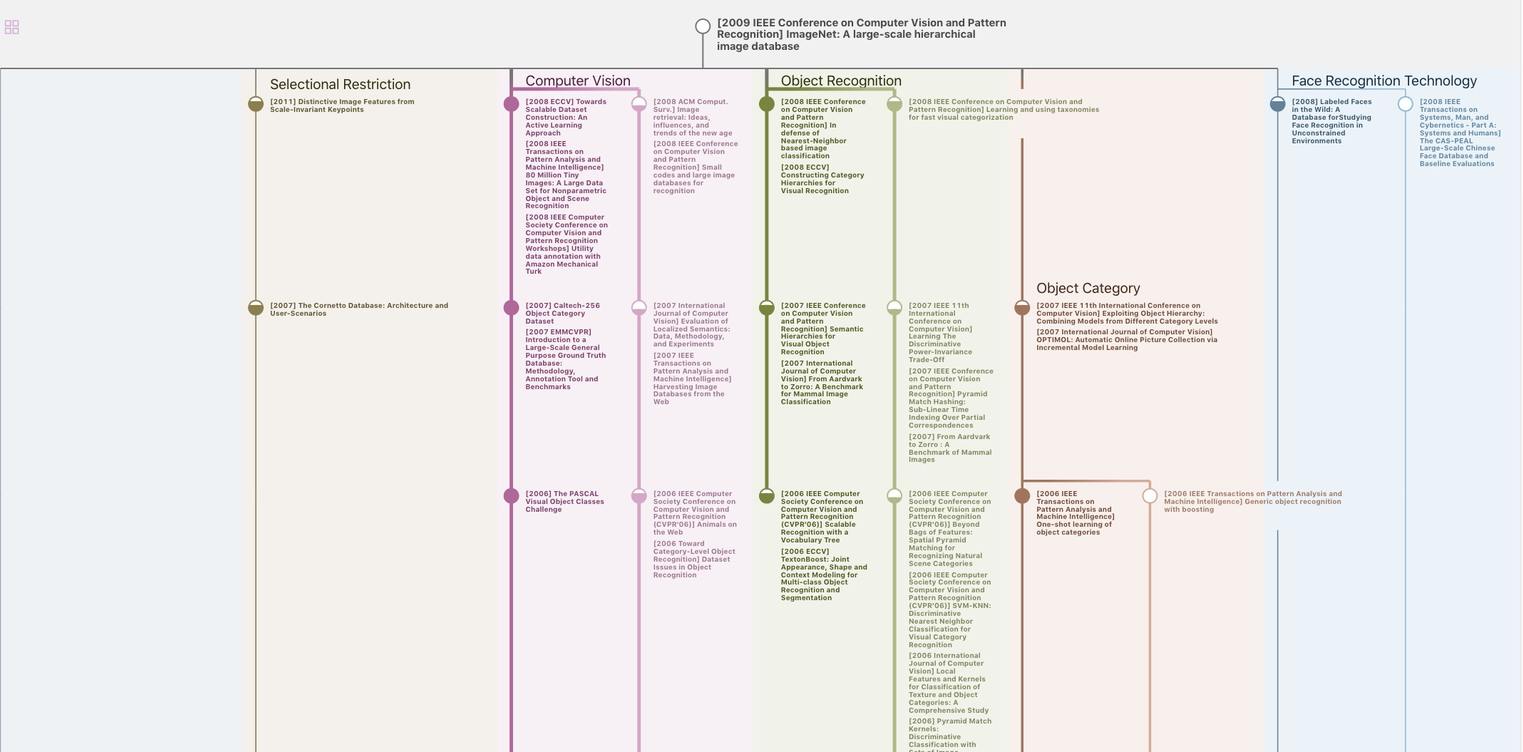
生成溯源树,研究论文发展脉络
Chat Paper
正在生成论文摘要