Reinforcement Learning Method with Dynamic Learning Rate for Real-Time Route Guidance Based on SUMO
2022 17th International Conference on Control, Automation, Robotics and Vision (ICARCV)(2022)
摘要
The increasing number of vehicles and dynamic changes in traffic situations make real-time route planning strongly necessary. The route-guiding method is supposed to cope with dynamic traffic situations. In addition, the ability to adapt to the second fastest route is very important when traffic congestion suddenly occurs on the fastest path. This paper proposes a method of using reinforcement learning to solve dynamic route planning problems, and the adaptation from a static learning rate to a dynamic learning rate enhances the capability to deal with emergent congestion. Meanwhile, the waiting time before each traffic light also is considered as a reward factor in the proposed algorithm. Contrast experiments have been conducted on the simulation network by SUMO, which has demonstrated well that our proposed method has better performance than other methods.
更多查看译文
关键词
dynamic changes,dynamic learning rate,dynamic route planning problems,dynamic traffic situations,fastest path,fastest route,real-time route guidance,real-time route planning,reinforcement learning,route-guiding method,static learning rate,SUMO,traffic congestion,traffic light,waiting time
AI 理解论文
溯源树
样例
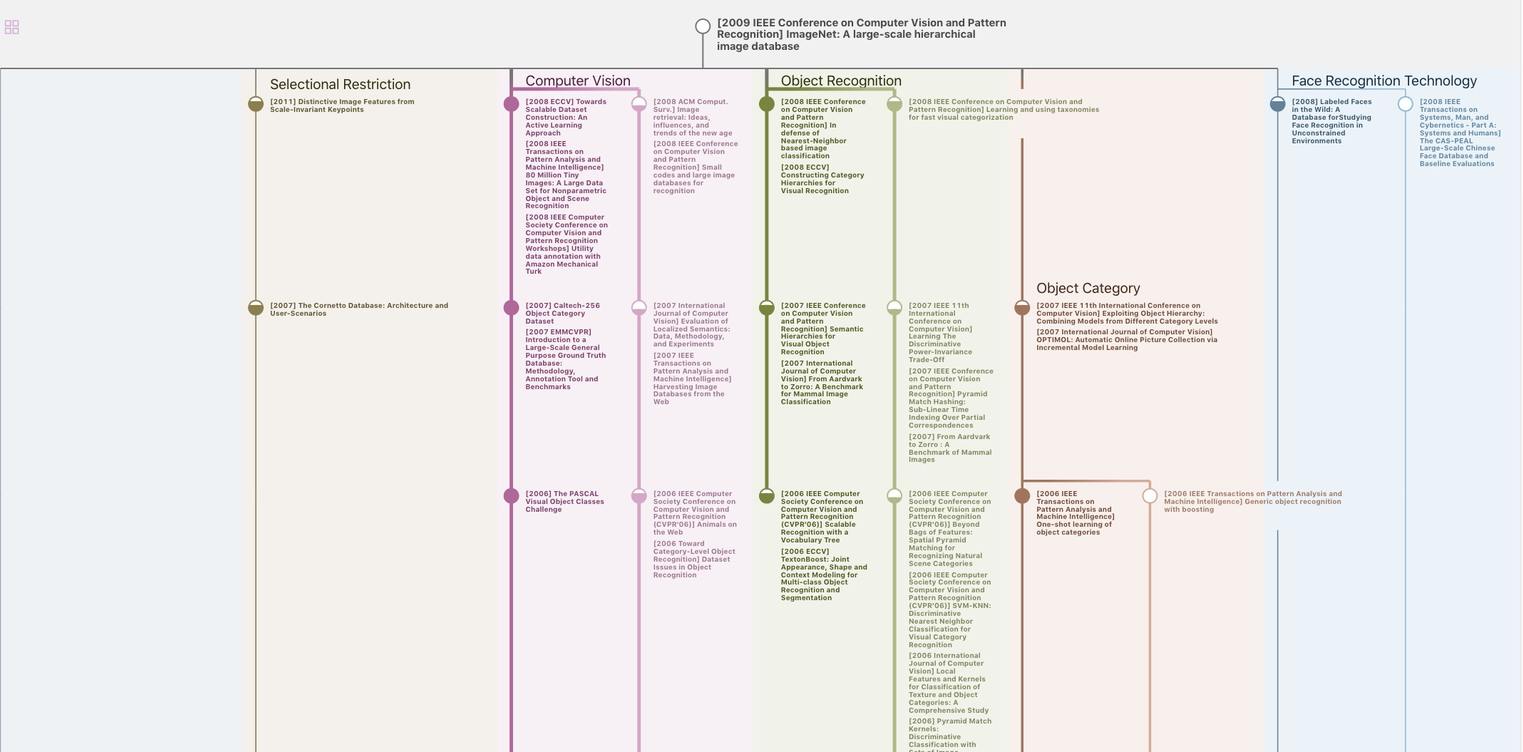
生成溯源树,研究论文发展脉络
Chat Paper
正在生成论文摘要