A Hybrid Deep Learning Based Anomaly Detection Framework dedicated for Big Research Infrastructures.
ICARCV(2022)
摘要
Anomaly detection has been an active artificial intelligence area in research and industrial communities with many unique problem complexities and challenges that require advanced approaches. In this research we concentrate on the analysis of anomalies occurring in big research infrastructures including signal measurement analysis as well as X-ray radiation images classification. The proposed hybrid end-to-end anomaly score learning framework consists of two deep learning based approached for anomaly detection dedicated for two different sources of high-dimensional data. The aim of the system is to identify abnormal status of sensors in certain time steps. In this study, we deploy two transfer learning architectures by examining pre-trained VGG-16, VGG-19, InceptionV3, Xception, as well as EfficientNet CNN models with an adjusted densely-connected classifiers. The system achieved overall accuracy of 94%, what is a state-of-the-art result in such research infrastructures.
更多查看译文
关键词
active artificial intelligence area,anomaly detection framework,big research infrastructures,densely-connected classifiers,EfficientNet CNN models,hybrid deep learning,hybrid end-to-end anomaly score learning,InceptionV3,industrial communities,pretrained VGG-16,signal measurement analysis,transfer learning architectures,unique problem complexities,VGG-19,X-ray radiation image classification,Xception
AI 理解论文
溯源树
样例
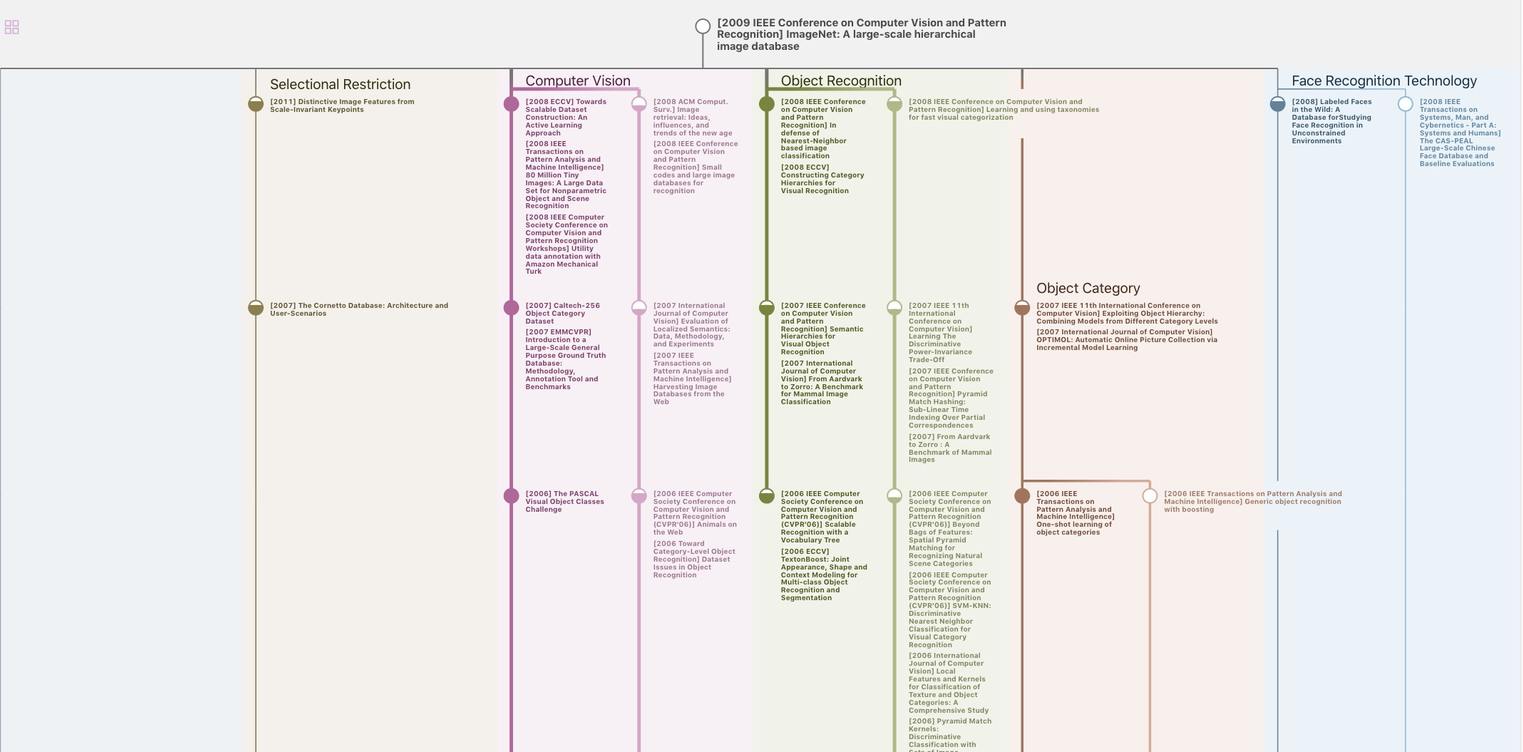
生成溯源树,研究论文发展脉络
Chat Paper
正在生成论文摘要