A Scenario Encoding Model for Long-Term Lane-Change Prediction Using Self-Organizing Map.
ICARCV(2022)
摘要
There is no doubt that in the near future, machines will share roads with human drivers [1] [2]. Therefore, the prediction of human drivers' lane changing behavior is imperative. Lane-change prediction is one of the most important ones. Both human drivers and autonomous vehicles should make sure that no other vehicle switches lanes or moves into the same region of the target lane as the ego vehicle. The existing short-term prediction algorithms can only provide a prediction horizon of 3 similar to 5s, leaving only a limited reaction time for drivers and autonomous path planning modules. Additionally, the majority of previous research analysed less on investigate lane segmentation or merging, simply the inference of lane shift in an expressway context. Most of earlier research only focused on the inference of lane-change in an expressway context, as opposed to the more typical urban environment. There are relatively few of these studies that can handle multi-scenario and scenario switching. In this paper, a Scenario Encoding Model (SEM) is proposed to help solve the problem of long-term lane-change prediction and the scenario switching problem in the existing short-term lane-change prediction. Even in the absence of road history data, the SEM can model the road scenario and encode the real-time road scene by using Self-Organizing Map (SOM) In the mean time, the established initial model has the ability to be further evolved into a historical bias model in the background of a large amount of road historical data. The evaluation test of this SEM has been done through the NGSIM dataset.
更多查看译文
关键词
scenario encoding model,map,long-term,lane-change,self-organizing
AI 理解论文
溯源树
样例
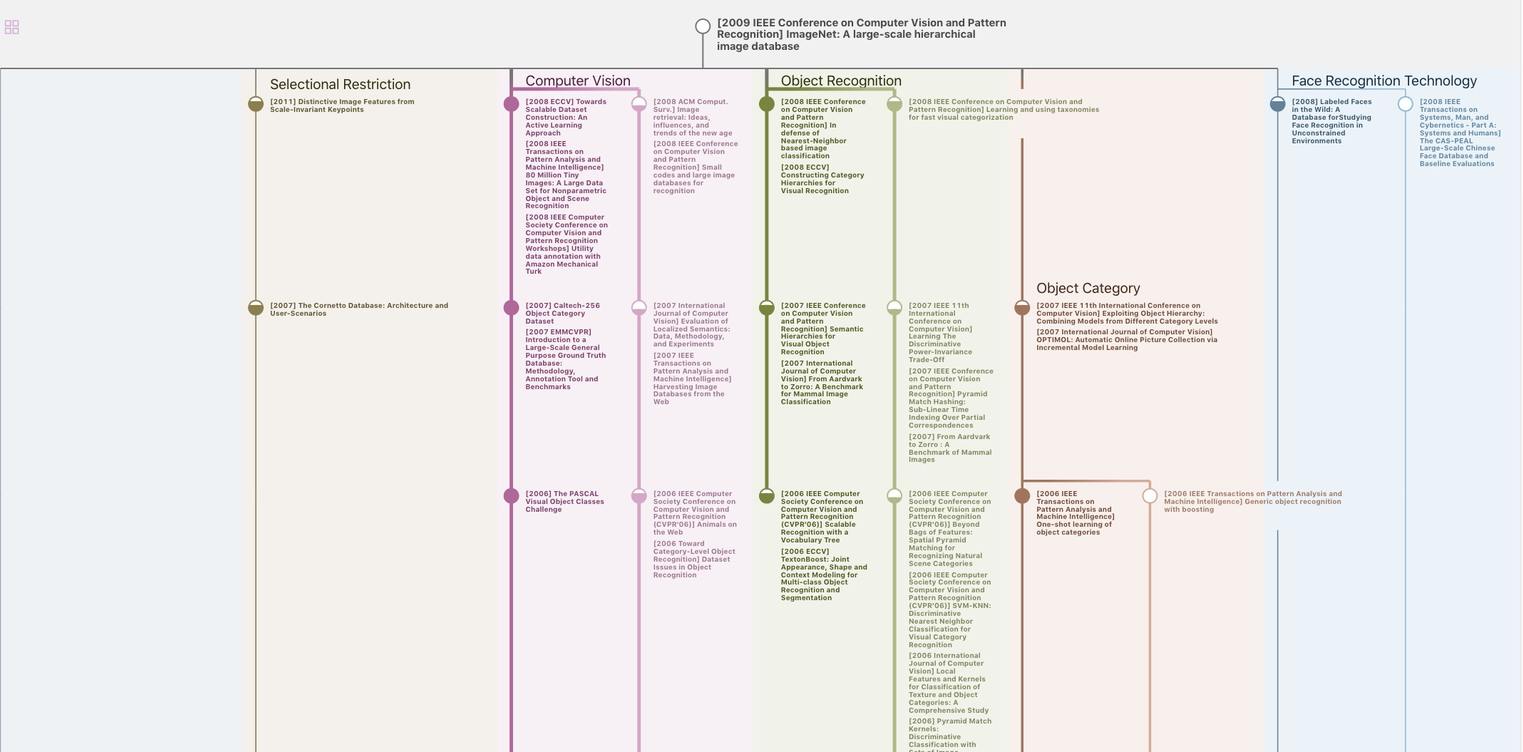
生成溯源树,研究论文发展脉络
Chat Paper
正在生成论文摘要