Ensemble CNN Attention-Based BiLSTM Deep Learning Architecture for Multivariate Cloud Workload Prediction
PROCEEDINGS OF THE 24TH INTERNATIONAL CONFERENCE ON DISTRIBUTED COMPUTING AND NETWORKING, ICDCN 2023(2023)
Abstract
Cloud computing has drastically changed the nature of computing in recent years. However, despite its countless benefits, it also suffers from some major challenges including autoscaling of resources and high energy consumption resulting inefficient and costly cloud systems. Among these challenges, the auto-scaling of resources has received significant research attention. However, most of the works on auto-scaling utilize the reactive approach on workload fluctuations. Therefore, we develop a deep learning based prediction architecture applying ensemble of attention mechanism (AM) with bi-directional long short-term memory (BiLSTM) and convolution neural network (CNN). Through experimental evaluation using real-time cloud workload traces, the proposed framework reduces RMSE by 8.93% and reduces MAE by 11.43% compared to the existing prediction models.
MoreTranslated text
Key words
Deep learning,Cloud computing,Data center,autoscaling,Workload prediction
AI Read Science
Must-Reading Tree
Example
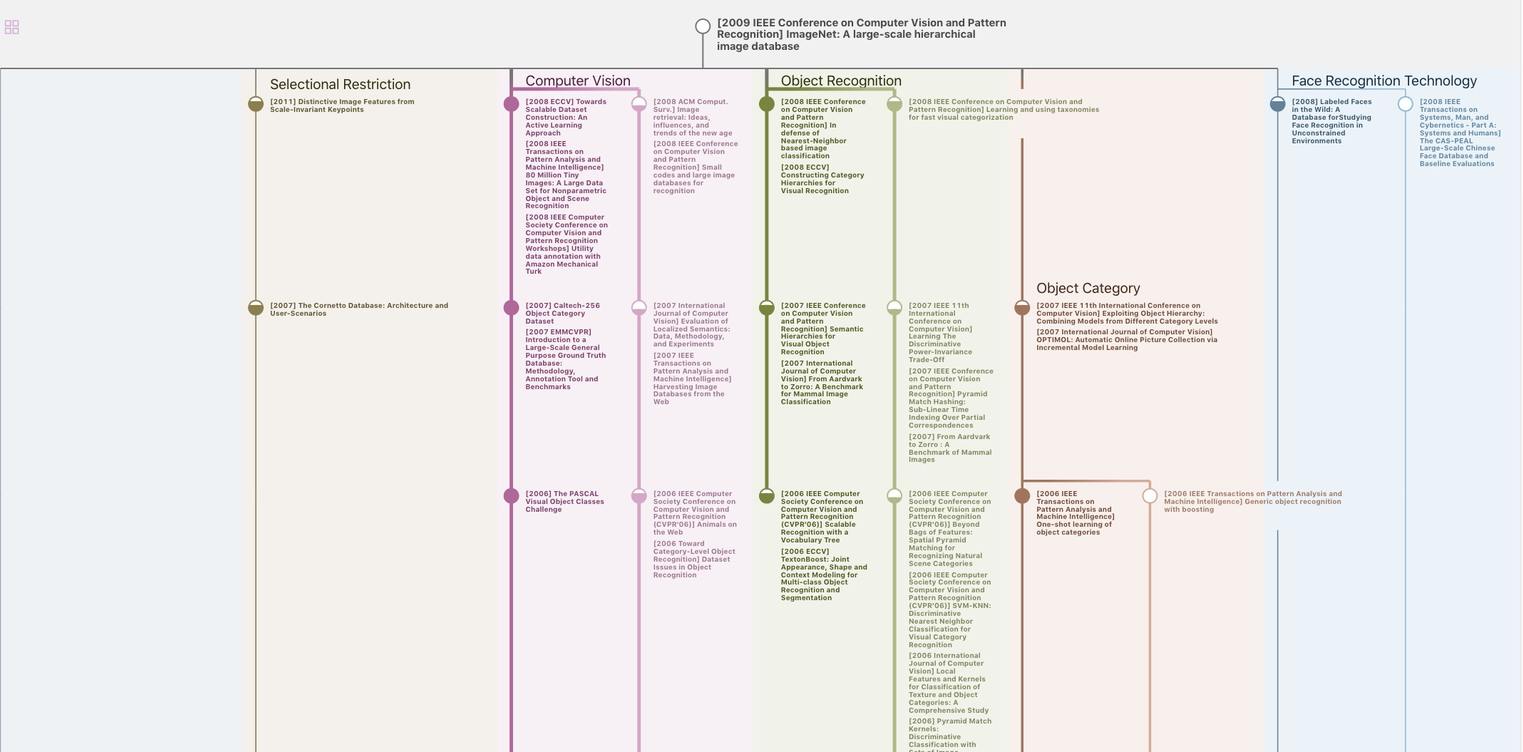
Generate MRT to find the research sequence of this paper
Chat Paper
Summary is being generated by the instructions you defined