Interpretable User Behavioral Analysis and Personalized Recommendation with Side Information
WSC(2022)
Abstract
Due to information overload, recommender system is developed and widely applied to better predict customer behaviors empowered by interaction data. In this paper, we develop an interpretable recommender system to improve click behavior prediction accuracy. Our proposed recommender system consists of i) designing a user-item interaction rating system defined as the weighted-sum of the multiple interaction variables, where the weight vector is regarded as hyperparameter, ii) using matrix factorization technique to capture the latent structure from the observable user-item interaction, and predict personalized ratings for items not shown to users, and iii) determining the weight vector by optimizing AUC which evaluates click behavior prediction accuracy on a validation set. To be specific, an algorithm with radial basis function surrogates is applied for hyperparameter optimization. We also develop a case study with user behavioral data from Netease music and observe a 15.5% improvement in AUC when incorporating multiple information sources.
MoreTranslated text
Key words
click behavior prediction accuracy,customer behavior prediction,hyperparameter optimization,information overload,interaction data,interaction variables,interpretable recommender system,interpretable user behavioral analysis,matrix factorization,multiple information sources,Netease music,personalized recommendation,radial basis function,side information,user behavioral data,user-item interaction rating system,weight vector
AI Read Science
Must-Reading Tree
Example
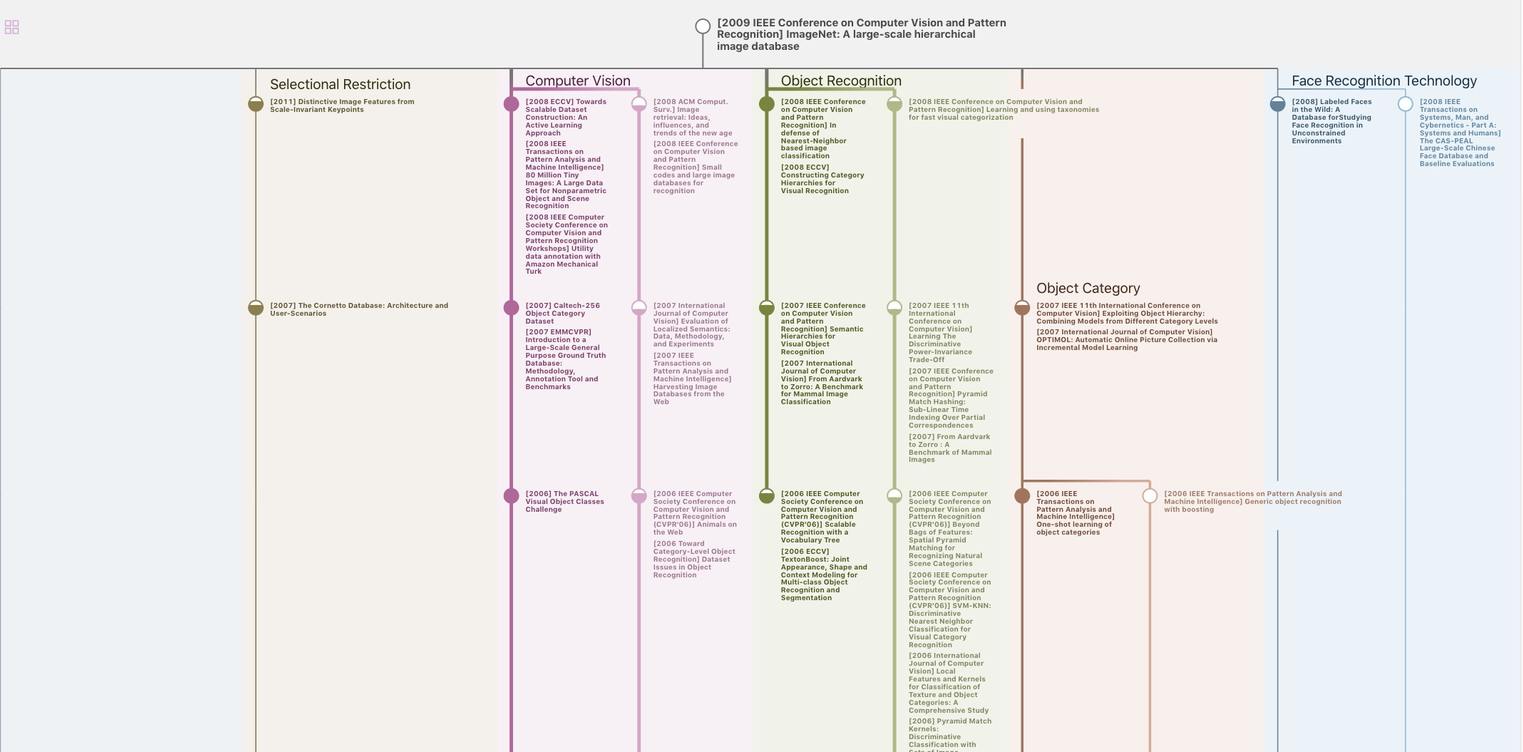
Generate MRT to find the research sequence of this paper
Chat Paper
Summary is being generated by the instructions you defined