Multi-Fidelity Discrete Optimization via Simulation.
WSC(2022)
摘要
Digital simulation is a prevalent tool to evaluate the performance of complex industrial systems. In this paper, we consider the discrete optimization via simulation (DOvS) problems, where the design space is an integer lattice. In addition, we are interested in leveraging cheap low-fidelity models to enhance optimization efficiency. An innovative Gaussian Markov random field (GMRF), which adaptively evolves along with the optimization process, is introduced to exploit the spatial and inter-model relationships among the objective function values of designs. We then propose the Multi-Fidelity Gaussian Markov Improvement Algorithm (MFGMIA) under the Bayesian global optimization framework. The numerical experiments show that it can achieve significant performance improvement by properly using low-fidelity information.
更多查看译文
关键词
optimization,simulation,multi-fidelity
AI 理解论文
溯源树
样例
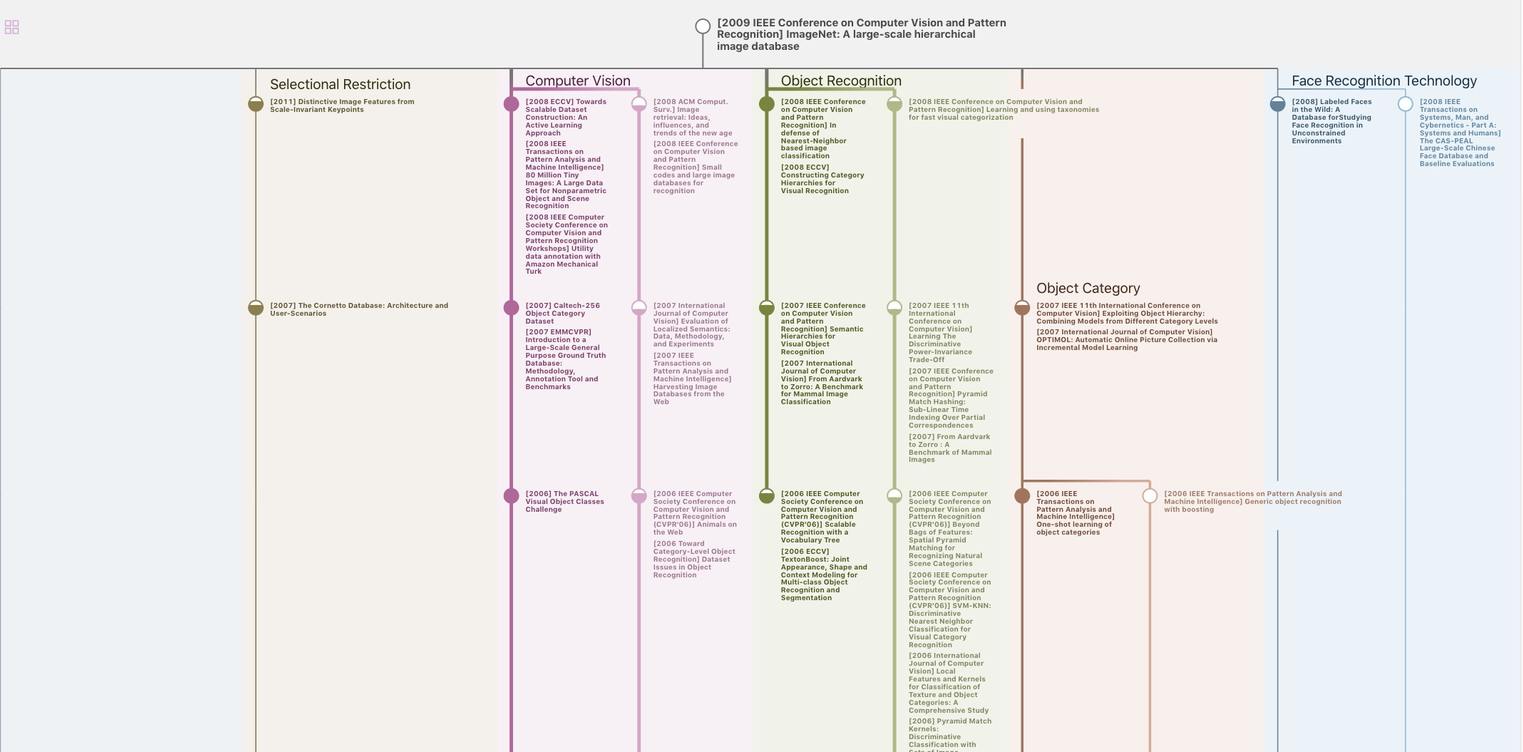
生成溯源树,研究论文发展脉络
Chat Paper
正在生成论文摘要