Towards AI Robustness Multi-Agent Adversarial Planning in Game Play.
WSC(2022)
摘要
This paper applied Monte Carlo Tree Search (MCTS) and its variant algorithm "Upper Confidence bounds applied to Trees" (UCT) to Chinese checker game and visualized it in the Ludii games portal. Since the MCTS approach cannot guarantee a finite game length in our simulation and the winning strategy is inefficient, we developed a Convolution Neural Network (CNN) model formulated on top of the MCTS algorithm to improve the performance of the Chinese checker game. Our experiment and simulations show that by using the weak labels generated by MCTS algorithm, the CNN-based model could learn without supervised signals from human interventions and execute the game strategy in a finite time. PyGame and the Ludii game portal are used in our simulation to visualize the game and show the final game results.
更多查看译文
关键词
robustness,game play,planning,multi-agent
AI 理解论文
溯源树
样例
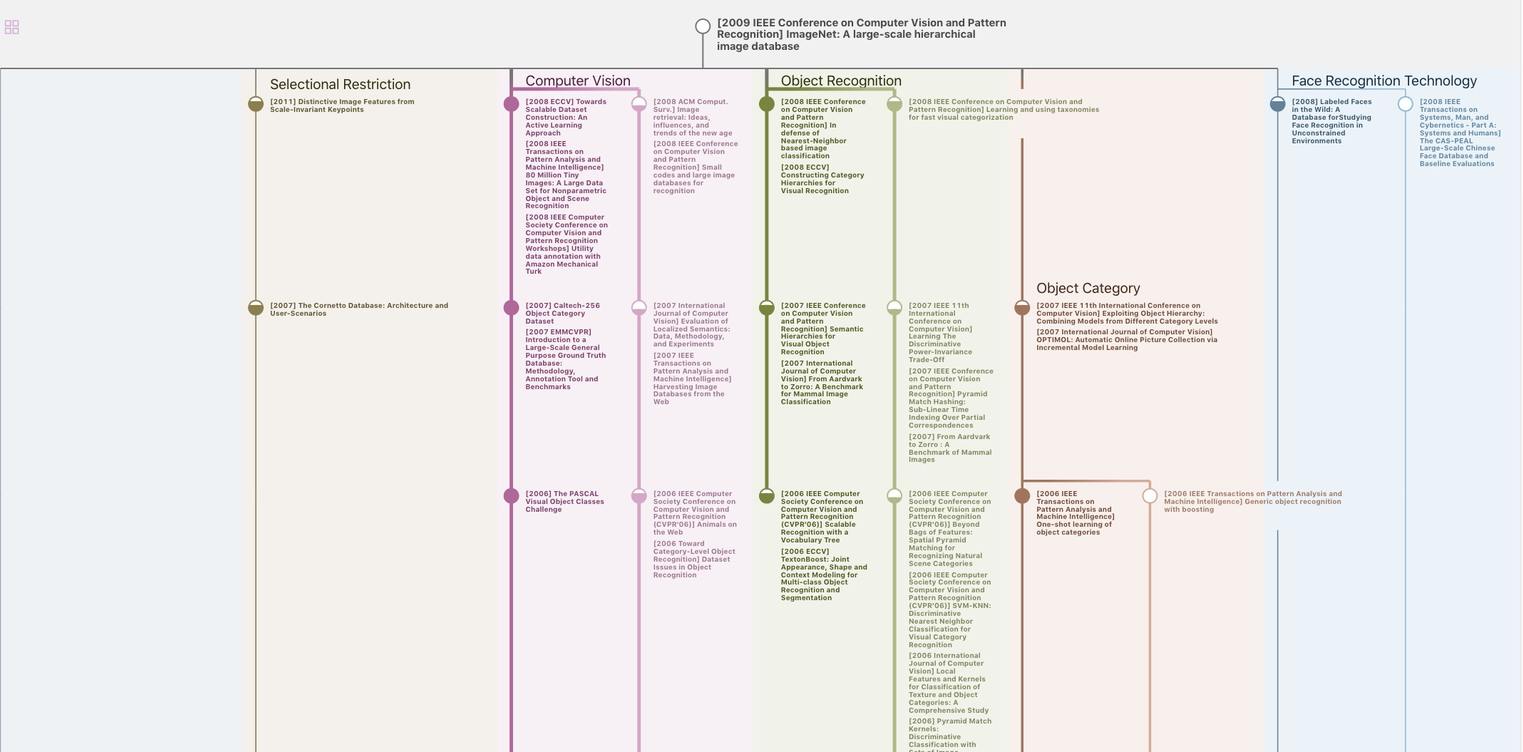
生成溯源树,研究论文发展脉络
Chat Paper
正在生成论文摘要