Medical Knowledge Discovery by Randomly Sampled “patient Characteristics” Formatted Data
2022 TENTH INTERNATIONAL SYMPOSIUM ON COMPUTING AND NETWORKING WORKSHOPS, CANDARW(2022)
Abstract
Statistical processing and Artificial Intelligence (AI) development utilizing big data have been actively researched recently. However, there are growing concerns about privacy violations due to the use of private data. In response to such concerns, the EU General Data Protection Regulation (GDPR) was introduced to regulate the handling of personal information. GDPR makes it difficult to discover medical knowledge through big data analysis in medical studies. However, GDPR is not con-cerned with handling non-personally identifiable statistical information. Statistical information is commonly published, collected, and analyzed. Yet, it is unknown whether collecting and analyzing such statistical information can generate medical evidence through variable-to-variable research, such as the relationship between tobacco and cancer. Therefore, in this paper, we propose to use statistical information that is not concerned by GDPR to estimate cross-tabulation tables, which are usually generated from personal information in medical research and are widely used for analysis between medical variables. In particular, as statistical information, we use “patient characteristics” formatted data, commonly published in medical research. The scope of this paper is the situation where the publisher of statistical information and the analyst of published statistical information differ. On the publisher side, we assume the publisher collects raw data from a target people group by random sampling multiple times and converts the data to patient characteristics formatted data. On the analyst side, we assume the analyst collects those published many random sampled patient characteristics formatted data and estimates the cross-tabulation table by the Law of Large Numbers (LLN). Theoretically, we model the publisher-analyst situation described above. Practically, we validate the model by experiment. In the experiment, the target people group data is 20000 personal data which have four categorical binary values. As the publisher model, we created 10000 patient characteristics, which are statistics for randomly sampled 50 data from the 20000 data. As the analyst model, we estimated the cross-tabulation table by the 10000 patient characteristics. As the results of 100 times patient characteristics creation and estimation experiments, we obtained the cross-tabulation tables with less than or equal to 1.5% error (2 standard deviations (SD)). From these results, we conclude that our proposed model of publishing and collecting patient characteristics formatted data allows precise estimation of the cross-tabulation table.
MoreTranslated text
Key words
Random sampling,health care,patient characteristics
AI Read Science
Must-Reading Tree
Example
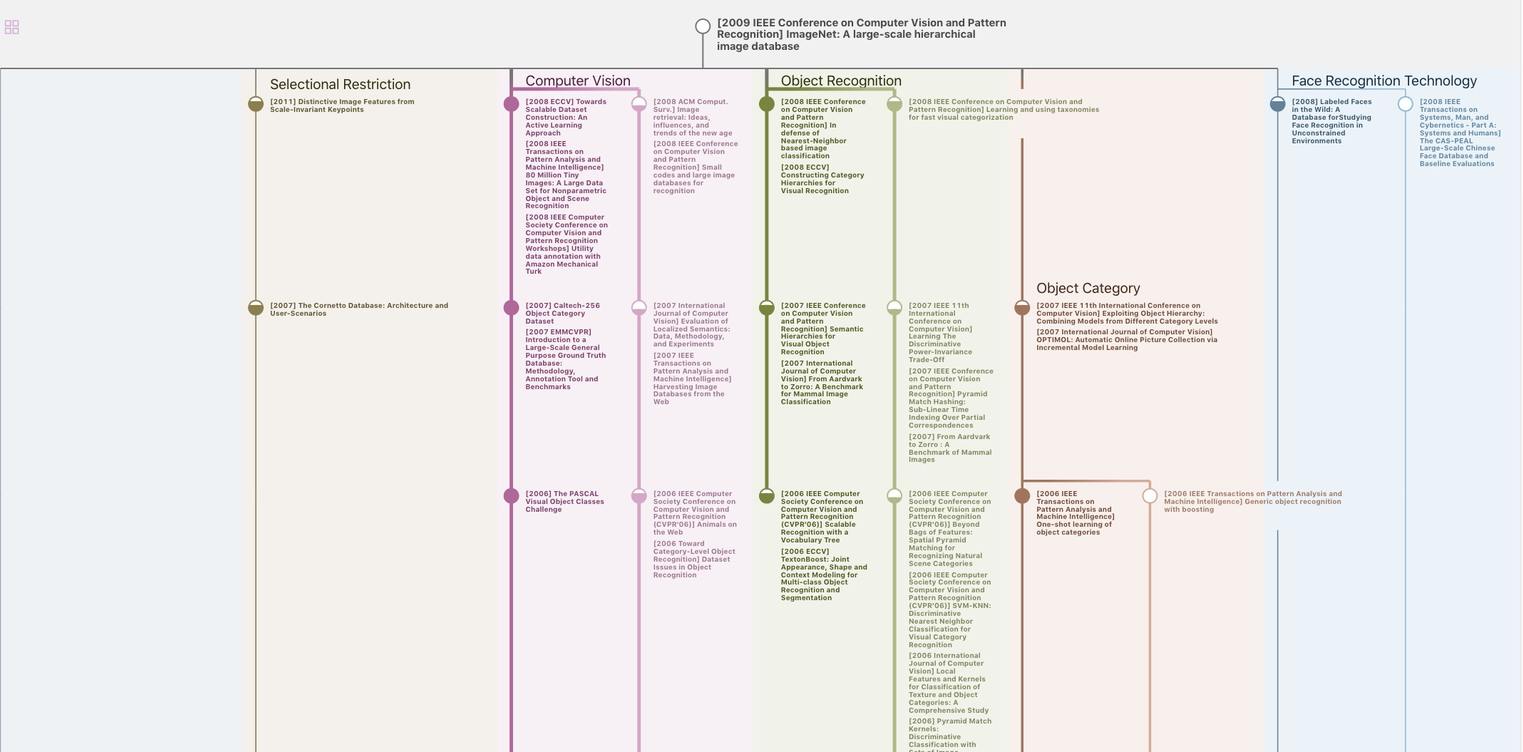
Generate MRT to find the research sequence of this paper
Chat Paper
Summary is being generated by the instructions you defined