EEG-based visual stimuli classification via reusable LSTM
Biomed. Signal Process. Control.(2023)
摘要
Visual classification based on electroencephalography (EEG) signals has attracted increasing attention. However, the classification accuracy still needs to be improved. In this study, a classification model Reusable LSTM Network (RLN) is proposed, which combines LSTM (Long Short-Term Memory) with a one-dimensional convolutional network to extract features for each channel separately. Using only 0.79M parameters, our method RLN achieves 52.69 % accuracy on the six-class classification task on the Object Category EEG Dataset (OCED), outperforming all other prior solutions. We further explored whether the classification of EEG signals is based on primary visual features or higher-level conceptual features. The results show that the highest accuracy for classification in this task is based on signals from the visual area (occipital lobe) where the primary visual features are represented. Signals from the frontal regions, however, were the least effective. It is speculated that the current classification based on EEG signals may rely more on primary visual features, instead of higher level conceptual features.
更多查看译文
关键词
Visual stimuli,Electroencephalography,Brain -computer interface,Neural networks,Deep learning
AI 理解论文
溯源树
样例
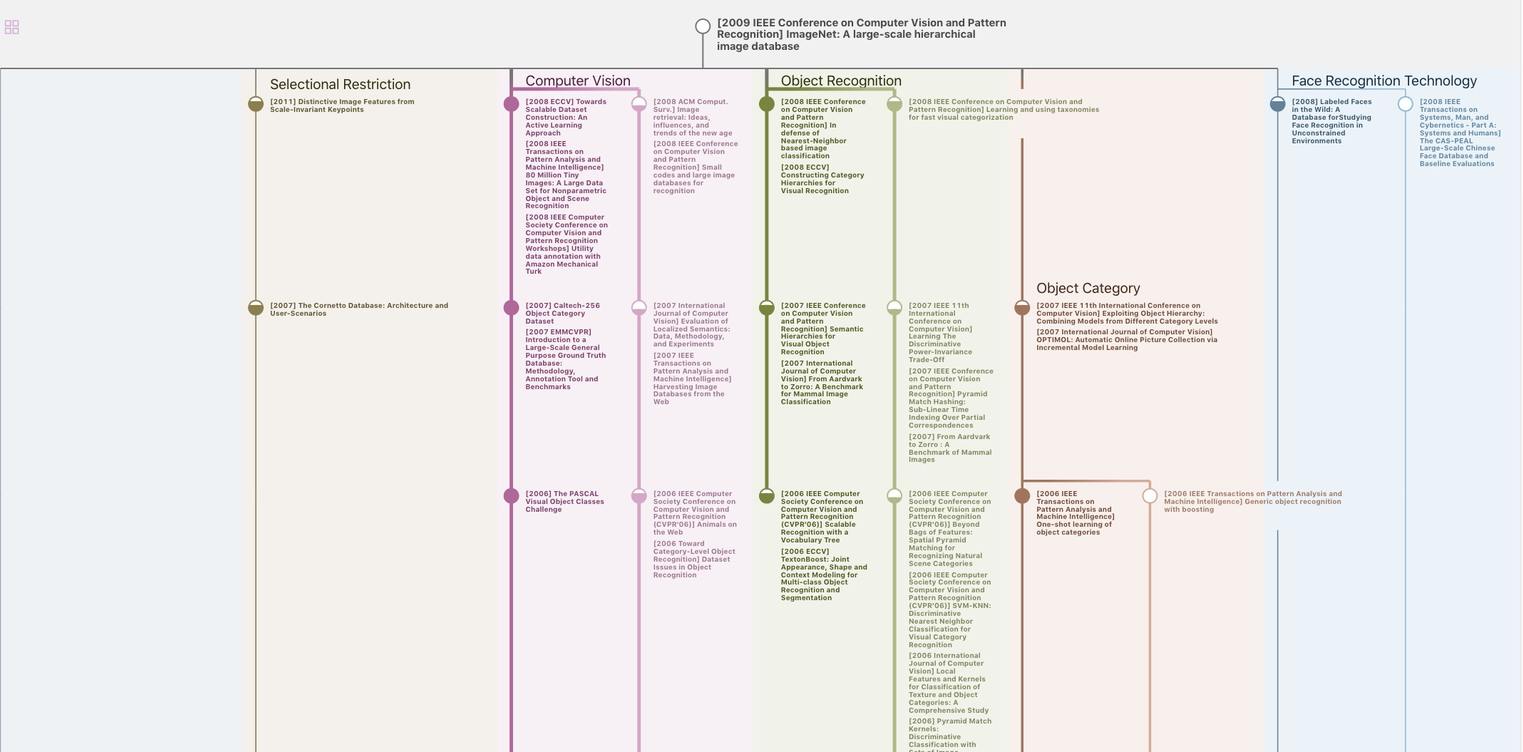
生成溯源树,研究论文发展脉络
Chat Paper
正在生成论文摘要