SG-Net: Semantic Guided Network for Image Dehazing.
ACCV (3)(2022)
摘要
From traditional handcrafted priors to learning-based neural networks, image dehazing technique has gone through great development. In this paper, we propose an end-to-end Semantic Guided Network (SG-Net (Codebase page: https://github.com/PaulTHong/Dehaze-SG-Net)) for directly restoring the haze-free images. Inspired by the high similarity (mapping relationship) between the transmission maps and the segmentation results of hazy images, we found that the semantic information of the scene provides a strong natural prior for image restoration. To guide the dehazing more effectively and systematically, we utilize the information of semantic segmentation with three easily portable modes: Semantic Fusion (SF), Semantic Attention (SA), and Semantic Loss (SL), which compose our Semantic Guided (SG) mechanisms. By embedding these SG mechanisms into existing dehazing networks, we construct the SG-Net series: SG-AOD, SG-GCA, SG-FFA, and SG-AECR. The outperformance on image dehazing of these SG networks is demonstrated by the experiments in terms of both quantity and quality. It is worth mentioning that SG-FFA achieves the state-of-the-art performance.
更多查看译文
关键词
Image dehazing, Semantic attention, Perception loss
AI 理解论文
溯源树
样例
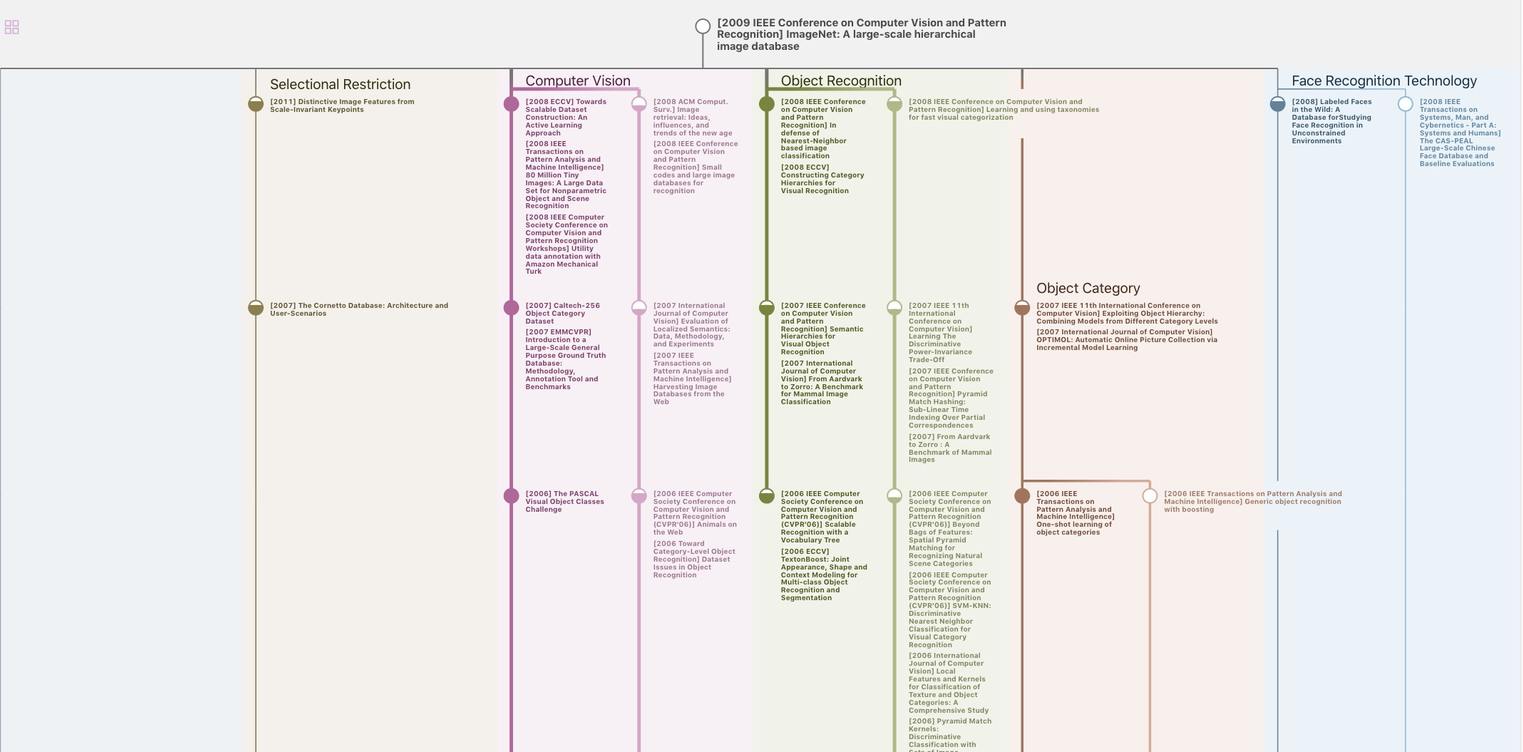
生成溯源树,研究论文发展脉络
Chat Paper
正在生成论文摘要