Content-Aware Hierarchical Representation Selection for Cross-View Geo-Localization.
ACCV (5)(2022)
摘要
Cross-view geo-localization (CVGL) aims to retrieve the images that contain the same geographic target content and are from different views. However, the target content usually scatters over the whole image, and they are indiscernible from the background. Thus, it is difficult to learn feature representation that focuses on these contents, rendering CVGL a challenging and unsolved task. In this work, we design a Content-Aware Hierarchical Representation Selection (CA-HRS) module, which can be seamlessly integrated into current deep networks to facilitate CVGL. This module can help focus more on the target content while ignoring the background region, thus as to learn more discriminative feature representation. Specifically, this module learns hierarchical important factors to each location of the feature maps according to their importance and enhances the feature representation based on the learned factors. We conduct experiments on several large-scale datasets (i.e., University-1652, CVUSA and CVACT), and the experiment results show the proposed module can obtain obvious performance improvement over current competing algorithms. Codes are available at https://github.com/Allen-lz/CA-HRS.
更多查看译文
关键词
Geo localization, Feature selection, Image retrieval
AI 理解论文
溯源树
样例
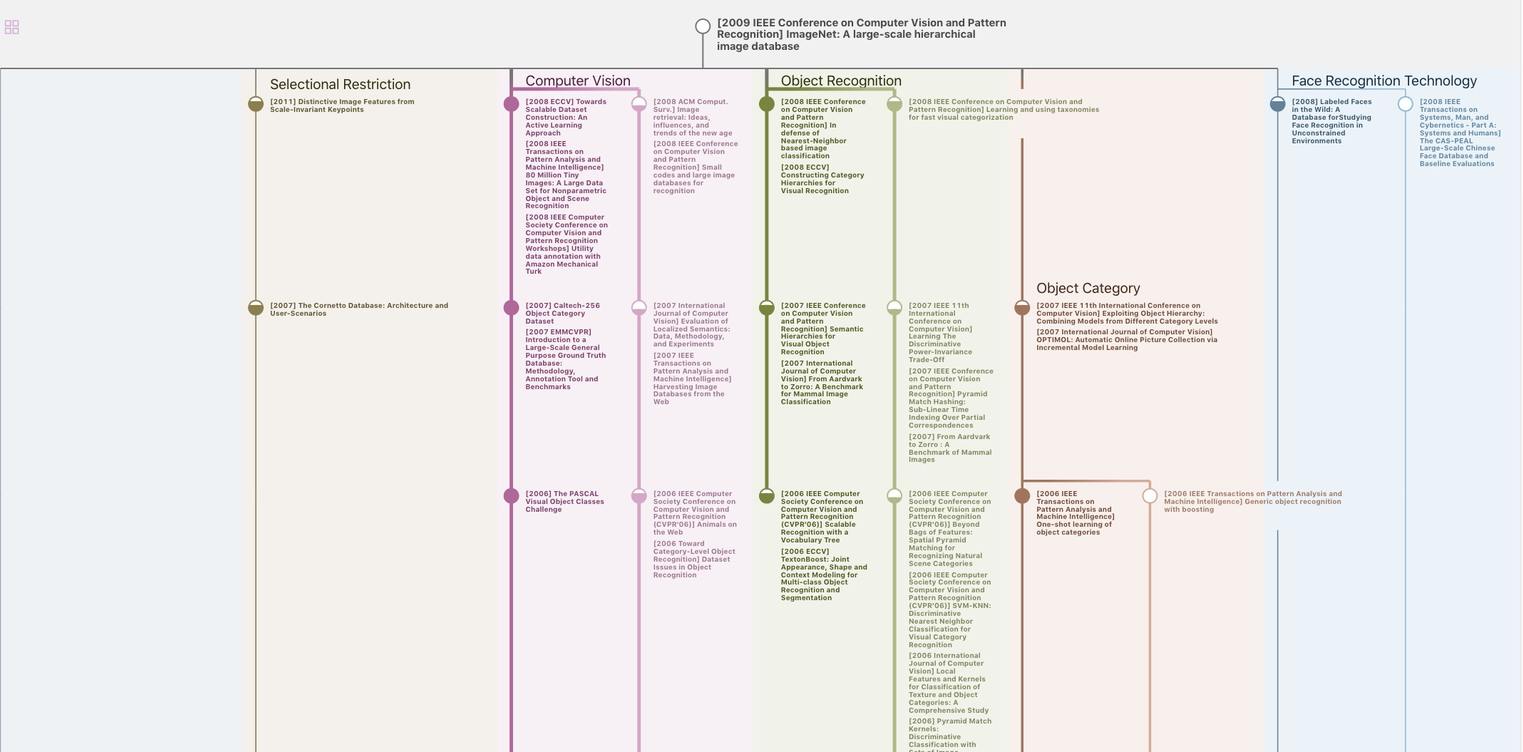
生成溯源树,研究论文发展脉络
Chat Paper
正在生成论文摘要