AutoOLA: Automatic object level augmentation for wheat spikes counting.
Comput. Electron. Agric.(2023)
摘要
The ability to count wheat spikes is one of the most critical indicators in wheat plant agriculture that correlates positively with yield estimation. Numerous studies have developed machine vision-based high-throughput phenotyping algorithms and demonstrated that the developed models could accurately detect and count wheat spikes. However, the drawback of previously developed models is their expensive and time-consuming data preparation process. While capturing images of wheat is relatively straightforward, annotating thousands of small wheat spikes in the collected dataset requires immense time and effort. This study, therefore, proposes a novel augmentation algorithm, Automatic Object Level Augmentation (AutoOLA), that can considerably reduce the required number of training samples in Deep Learning (DL) models. In this algorithm, different objects in the wheat images, including the spikes, leaves, stems, and backgrounds, are decoupled and augmented independently based on their augmentation policy. The augmented images are then generated by randomly assembling the augmented objects. In addition, an evolutionary optimization algorithm is used to automatically design the types of transformations and their magnitudes for each decoupled object. The results demonstrated that the proposed AutoOLA technique could improve wheat spike counting performance by up to 60 percent. With only one trainable image, the employed hybrid AutoOLA-DL model can predict the number of wheat spikes in the field with Root Mean Squared Error (RMSE), Mean Absolute Error (MAE), and Mean Absolute Percentage Error (MAPE) values of 2.695, 2.085, and 13.817, respectively.
更多查看译文
关键词
automatic object level augmentation,wheat spikes
AI 理解论文
溯源树
样例
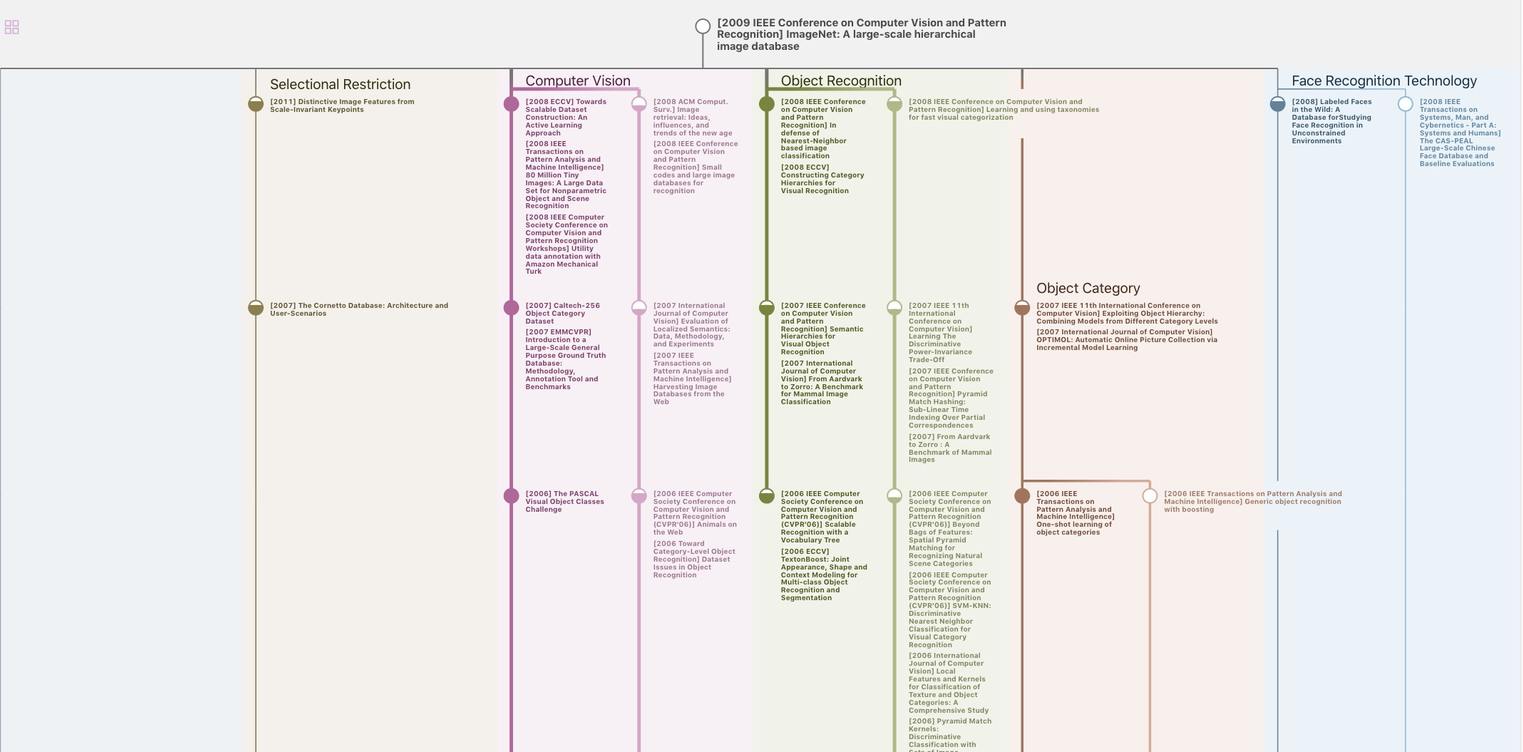
生成溯源树,研究论文发展脉络
Chat Paper
正在生成论文摘要