Discovering the Ancient Tomb under the Forest Using Machine Learning with Timing-Series Features of Sentinel Images: Taking Baling Mountain in Jingzhou as an Example
REMOTE SENSING(2023)
摘要
Cultural traces under forests are one of the main problems affecting the identification of archaeological sites in densely forested areas, so it is full of challenges to discover ancient tombs buried under dense vegetation. The covered ancient tombs can be identified by studying the time-series features of the vegetation covering the ancient tombs on the multi-time series remote sensing images because the ancient tombs buried deep underground have long-term underground space structures, which affect the intrinsic properties of the surface soil so that the growth status of the covering vegetation is different from that of the vegetation in the area without ancient tombs. We first use the highly detailed DSM data to select the ancient tombs that cannot be visually distinguished on the optical images. Then, we explored and constructed the temporal features of the ancient tombs under the forest and the non-ancient tombs in the images, such as the radar timing-series features of Sentinel 1 and the multi-spectral and vegetation index timing-series features of Sentinel 2. Finally, based on these features and machine learning, we designed an automatic identification algorithm for ancient tombs under the forest. The method has been validated in Baling Mountain in Jingzhou, China. It is very feasible to automatically identify ancient tombs covered by surface vegetation by using the timing-series features of remote sensing images. Additionally, the identification of large ancient tombs or concentrated ancient tombs is more accurate, and the accuracy is improved after adding radar features. The paper concludes with a discussion of the current limitations and future directions of the method.
更多查看译文
关键词
ancient tombs identification under a forest,spectral timing-series features,remote sensing archaeology,satellite,machine learning
AI 理解论文
溯源树
样例
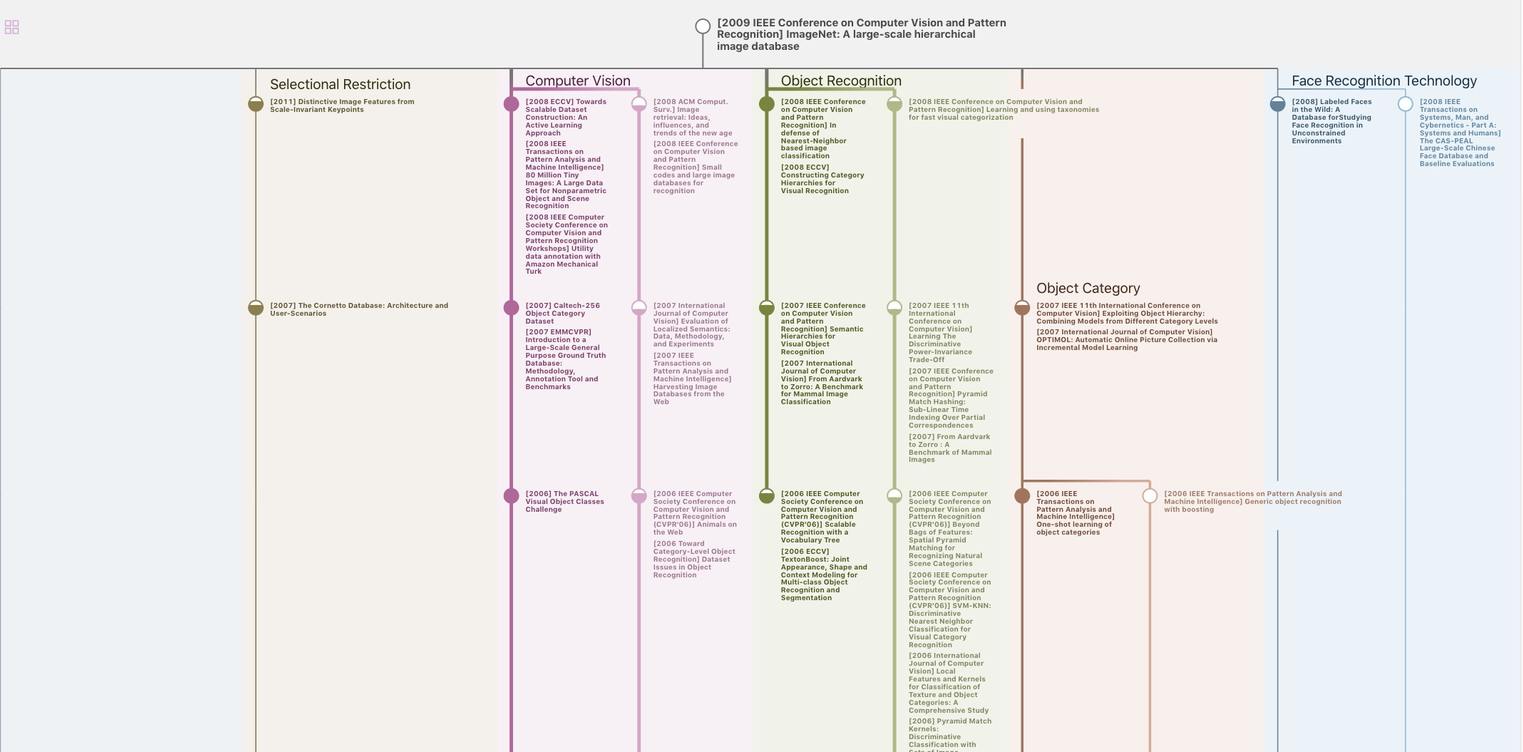
生成溯源树,研究论文发展脉络
Chat Paper
正在生成论文摘要