Mapping the Distribution and Dynamics of Coniferous Forests in Large Areas from 1985 to 2020 Combining Deep Learning and Google Earth Engine
Remote. Sens.(2023)
摘要
Mapping the distribution of coniferous forests is of great importance to the sustainable management of forests and government decision-making. The development of remote sensing, cloud computing and deep learning has provided the support of data, computing power and algorithms for obtaining large-scale forest parameters. However, few studies have used deep learning algorithms combined with Google Earth Engine (GEE) to extract coniferous forests in large areas and the performance remains unknown. In this study, we thus propose a cloud-enabled deep-learning approach using long-time series Landsat remote sensing images to map the distribution and obtain information on the dynamics of coniferous forests over 35 years (1985-2020) in the northwest of Liaoning, China, through the combination of GEE and U-2-Net. Firstly, to assess the reliability of the proposed method, the U-2-Net model was compared with three Unet variants (i.e., Resnet50-Unet, Mobile-Unet and U-Net) in coniferous forest extraction. Secondly, we evaluated U-2-Net's temporal transferability of remote sensing images from Landsat-5 TM, Landsat-7 ETM+ and Landsat-8 OLI. Finally, we compared the results obtained by the proposed approach with three publicly available datasets, namely GlobeLand30-2010, GLC_FCS30-2010 and FROM_GLC30-2010. The results show that (1) the cloud-enabled deep-learning approach proposed in this paper that combines GEE and U-2-Net achieves a high performance in coniferous forest extraction with an F1 score, overall accuracy (OA), precision, recall and kappa of 95.4%, 94.2%, 96.6%, 95.5% and 94.0%, respectively, outperforming the other three Unet variants; (2) the proposed model trained by the sample blocks collected from a specific time can be applied to predict the coniferous forests in different years with satisfactory precision; (3) Compared with three global land-cover products, the distribution of coniferous forests extracted by U-2-Net was most similar to that of actual coniferous forests; (4) The area of coniferous forests in Northwestern Liaoning showed an upward trend in the past 35 years. The area of coniferous forests has grown from 945.64 km(2) in 1985 to 6084.55 km(2) in 2020 with a growth rate of 543.43%. This study indicates that the proposed approach combining GEE and U-2-Net can extract coniferous forests quickly and accurately, which helps obtain dynamic information and assists scientists in developing sustainable strategies for forest management.
更多查看译文
关键词
coniferous forests,deep learning,U-2-Net,Google Earth Engine,Landsat
AI 理解论文
溯源树
样例
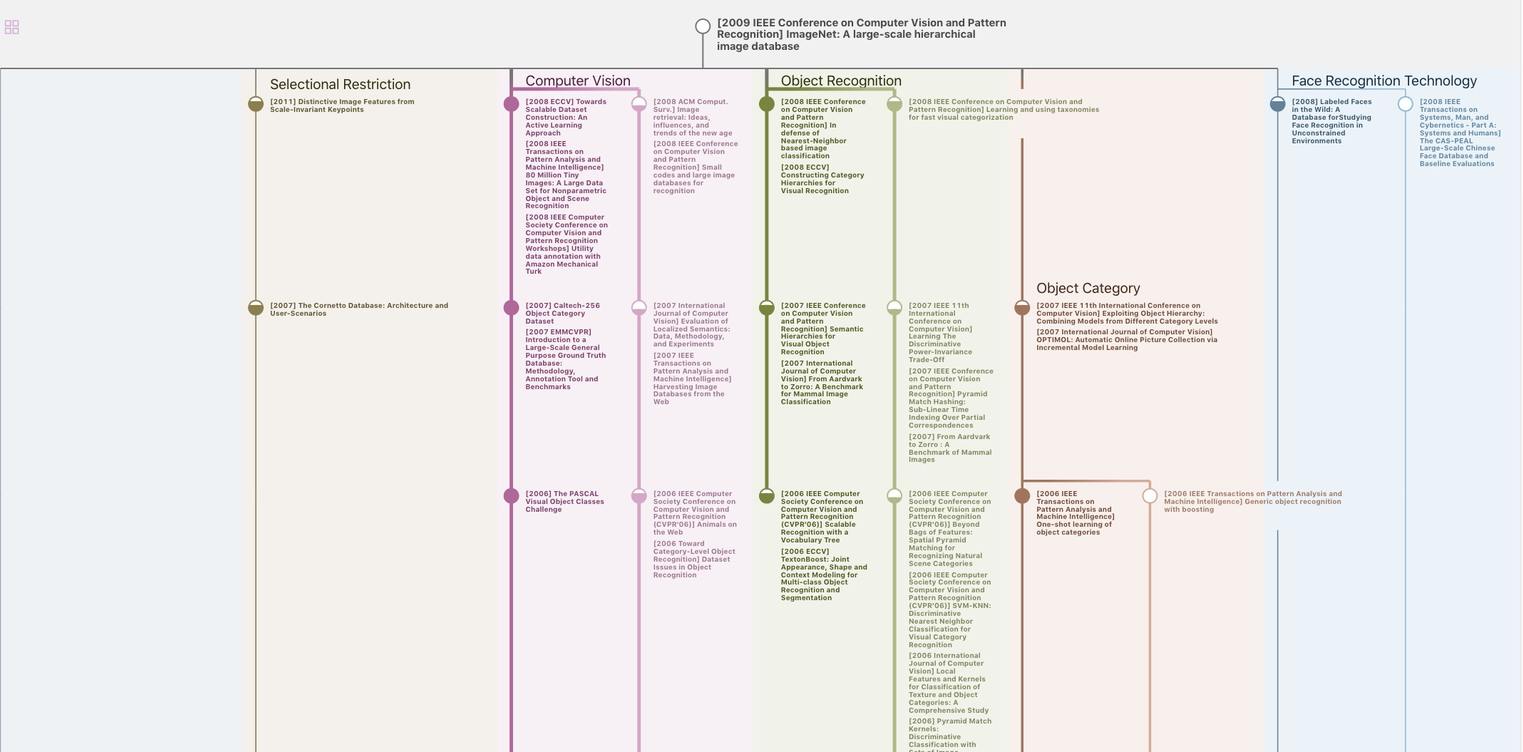
生成溯源树,研究论文发展脉络
Chat Paper
正在生成论文摘要