Attention to Both Global and Local Features: A Novel Temporal Encoder for Satellite Image Time Series Classification.
Remote. Sens.(2023)
摘要
Satellite image time series (SITS) classification is a challenging application concurrently driven by long-term, large-scale, and high spatial-resolution observations acquired by remote sensing satellites. The focus of current SITS classification research is to exploit the richness of temporal information in SITS data. In the literature, self-attention mechanism-based networks, which are capable of capturing global temporal attention, have achieved state-of-the-art results in SITS classification. However, these methods lack attention to local temporal information, which is also significant for SITS classification tasks. To explore the potential of different scales of temporal information in SITS data, a global-local temporal attention encoder (GL-TAE) is proposed in this paper. GL-TAE has two submodules set up in parallel, one of which is a lightweight temporal attention encoder (LTAE) for extracting global temporal attention and the other is lightweight convolution (LConv) for extracting local temporal attention. Compared with methods exploring global-only or local-only temporal features, the proposed GL-TAE can achieve better performance on two public SITS datasets, which proves the effectiveness of hybrid global-local temporal attention features. The experiments also demonstrate that GL-TAE is a lightweight model, which achieves the same performance as other models but with fewer parameters.
更多查看译文
关键词
satellite image time series (SITS),self-attention,lightweight temporal attention encoder (LTAE),lightweight convolution,land cover classification
AI 理解论文
溯源树
样例
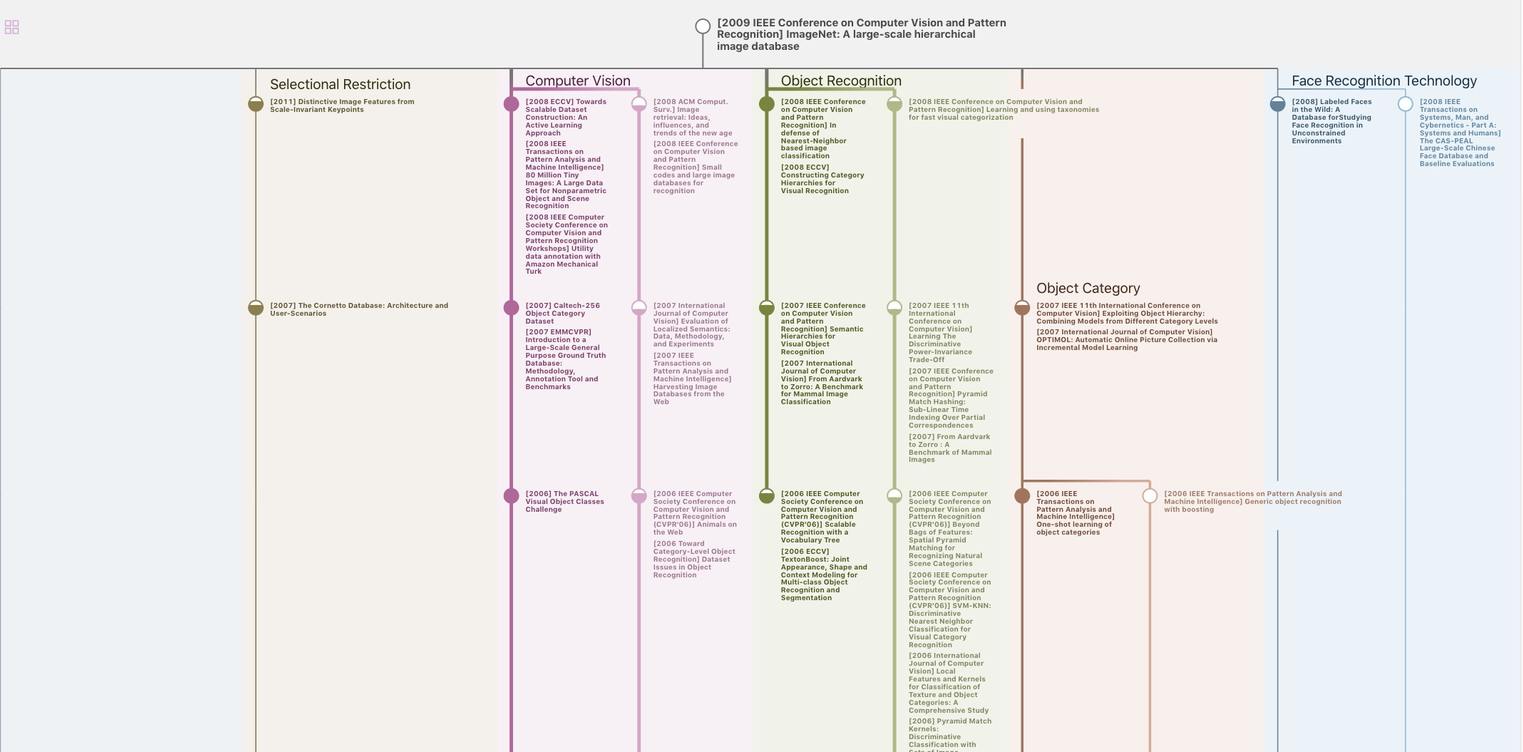
生成溯源树,研究论文发展脉络
Chat Paper
正在生成论文摘要