Robot suction region prediction method from knowledge to learning in disordered manufacturing scenarios.
Eng. Appl. Artif. Intell.(2023)
摘要
Suction plays an important role in the disordered manufacturing scenarios because of its single-point contact and reliability. The prediction of suction region is the primary problem needs to be solved in these applications. However, the traditional non-deep learning methods is insufficient for its poor generalization performance, and the deep learning method is inconvenient as it requires manually labeled datasets for training. Therefore, a suction region prediction method from knowledge to learning is proposed to overcome these challenges, which adopts a suction reliability matrix based on the depth image to label the disordered manufacturing datasets without manual labeling, where the effects of suction cup model, part centroid and depth are all considered. The disordered manufacturing dataset annotated is used to train the suction region prediction model based on fully convolutional neural network, which can be customized according to the model of suction cup and parts. Experiments and analysis show that the proposed method has the advantages of short detection time, high robustness and strong generalization ability.
更多查看译文
关键词
Disordered manufacturing scenarios,Suction reliability matrix,Depth image,Suction region prediction
AI 理解论文
溯源树
样例
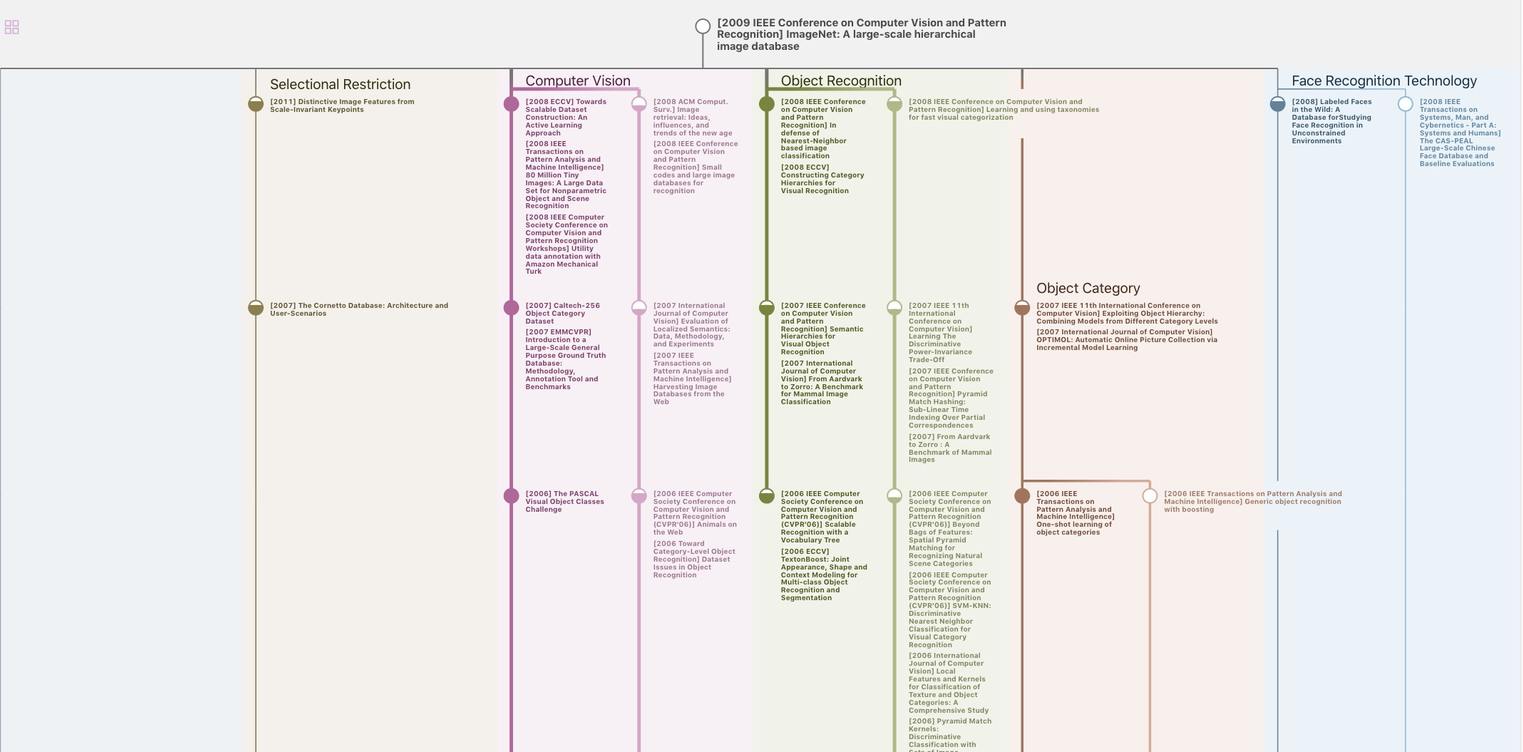
生成溯源树,研究论文发展脉络
Chat Paper
正在生成论文摘要