RoI Uncertainty for RGB-Thermal Image Segmentation.
MLMI(2022)
摘要
Estimating uncertainty for deep networks is a good way to assess the reliability of prediction results and helps self-driving cars avoid traffic accidents. Compared to single RGB or thermal image segmentation, fusing RGB and thermal adds more sources of uncertainty to object boundaries including blurred boundaries of thermal images and the discrepancy of object boundary between RGB and thermal images because the objects can not be perfectly aligned. Besides, it is important to model the relationship between uncertainty and segmentation quality of RGB-T segmentation. To address these challenges, we propose Region of Interest uncertainty (RoI uncertainty) to focus on the uncertainty inside an object with indicative meaning and ignore the boundary uncertainty due to the imaging mechanism and alignment mechanism. We improve the correlation between accuracy and RoI uncertainty compared to the correlation between accuracy and conventional uncertainty. The RoI uncertainty can be obtained not only by morphological operations based on estimated uncertainty but by deep learning-based RoI uncertainty networks using uncertainty as input. We design two types of RoI uncertainty estimation networks, one using RoI as labels called RoI-Net and the other using region uncertainty as labels called RoI-Unc-Net. Furthermore, we compare three uncertainty estimation methods including Monte Carlo Dropout (MC Dropout), Deep Ensemble, and MC Dropout Ensemble, and three measures including predictive entropy, mutual information, and variance that are introduced to quantify uncertainty. From extensive experiments on the RGB-T dataset, MC Dropout with entropy measure shows better uncertainty estimation and model calibration. The RoI uncertainty based on MC Dropout with entropy can be used to show the segmentation quality. Besides, it is an unsupervised metric to indicate the degree of risk of the segmentation result in real road scenes. We compare the growth rate of RoI uncertainty on several state-of-the-art RGB-T fusion methods, and the results show that fusing RGB and thermal features in multiple stages is more effective than a single-stage fusion strategy.
更多查看译文
AI 理解论文
溯源树
样例
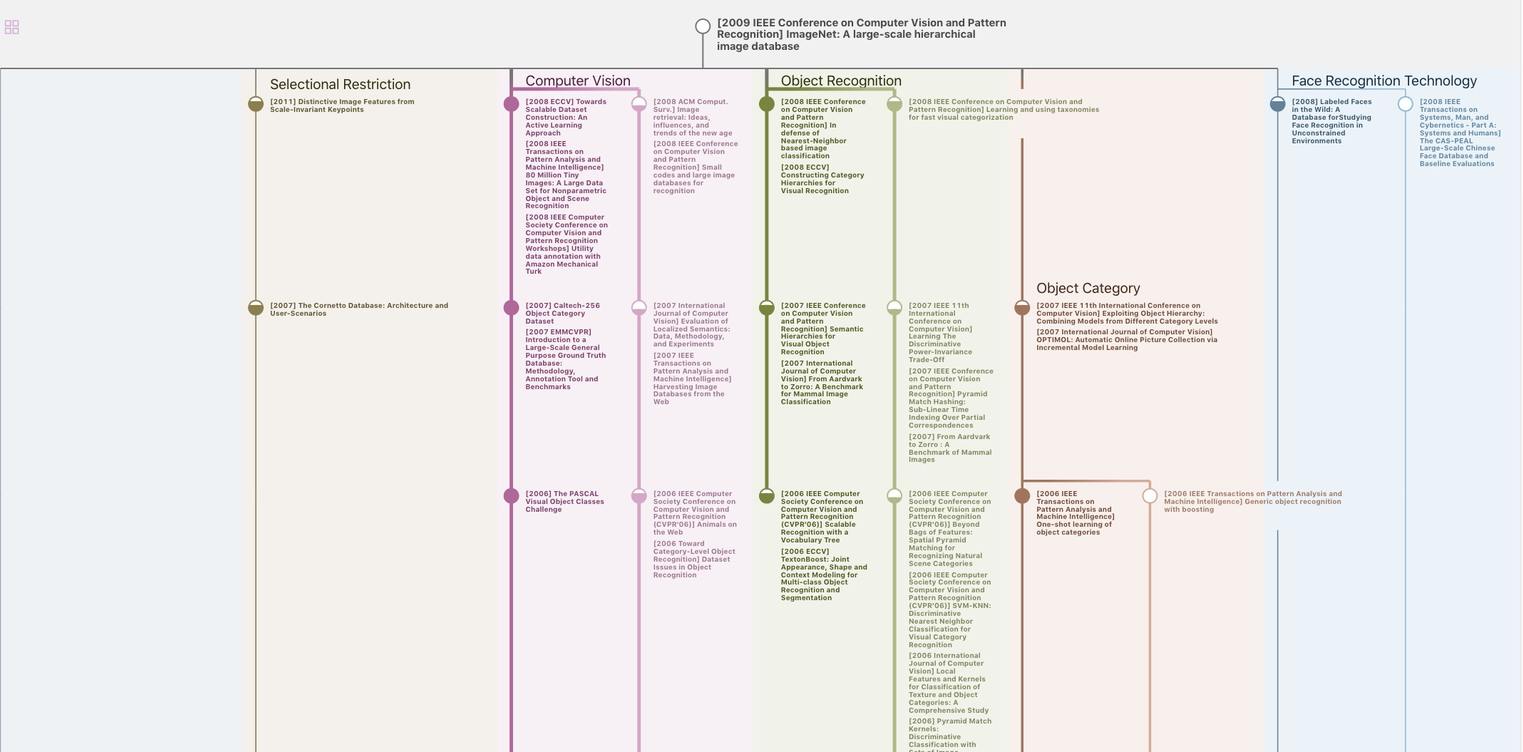
生成溯源树,研究论文发展脉络
Chat Paper
正在生成论文摘要