Optimal Graph Transformer Viterbi knowledge inference network for more successful visual navigation.
Adv. Eng. Informatics(2023)
摘要
Visual navigation recently incorporates priors in reinforcement learning (RL), which endows agent's associative ability in searching object task and has made promising results. However, there is the problem of poor exploration in end-to-end learning to new scenes. Besides, most of the priors only serve as the spatial features of scene layout without fully and directly providing guidance for RL's policy, thus making the navigation inefficient. To address these issues, we design a Graph Transformer Viterbi inference network (GTV), which finds new object relations and explores potential graph-based optimal actions in policy. Our method decomposes the RL's action to learn the adaptive priors in state representations and GTV based actions in policy, which generates a dense end-to-end learning signal and new graph edges in novel scenes. This, in turn, enables object-centric relational RL agents to learn policies faster and improve generalization ability. The results demonstrate our framework outperforms the baseline by 25.85% relatively on SPL (Success weighted by Path Length) and 30.64% on success rate, which also requires only 1/5 number of iterations to converge, compared with the baselines. Our code will be made publicly available in the scientific community.
更多查看译文
关键词
Visual navigation,Knowledge graph,Reinforcement learning,Graph transformer network,Knowledge inference
AI 理解论文
溯源树
样例
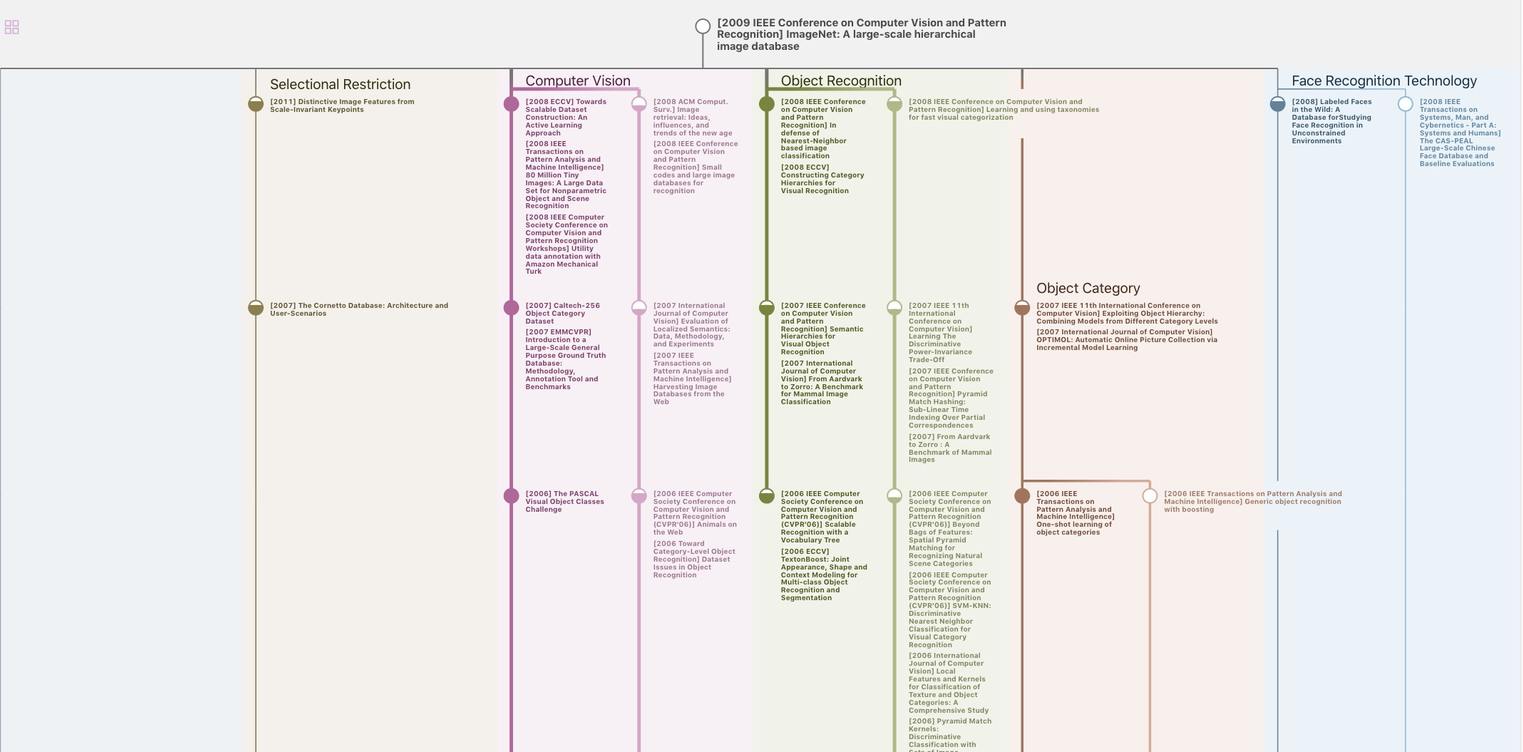
生成溯源树,研究论文发展脉络
Chat Paper
正在生成论文摘要