Towards Online Monitoring of Concrete Dam Displacement Subject to Time-Varying Environments: an Improved Sequential Learning Approach.
Advanced engineering informatics(2023)
摘要
Data-based models are widely applied in concrete dam health monitoring. However, most existing models are restricted to offline modeling, which cannot continuously track the displacement behavior with dynamic evolution patterns, especially in time-varying environments. In this paper, sequential learning is introduced to establish an online monitoring model for dam displacement behavior. This approach starts by considering the timeliness difference between old and new data using the forgetting mechanism, and a novel adaptive forgetting extreme learning machine (AF-ELM) is presented. A primary predictor based on AF-ELM is then formulated, which aims to sequentially learn the complex nonlinear relationship between dam displacement and main environmental factors. Considering the chaotic characteristics contained in the residual sequence of the primary predictor, a multi-scale residual-error correction (REC) strategy is devised based on divide-and-conquer scheme. Specifically, time-varying filter-based empirical mode decomposition is adopted to decompose the raw chaotic residual-error series into a set of subseries with more stationarity, which are further aggregated and reconstructed by fuzzy entropy theory and suitable approximation criterion. Finally, the corrected residual sequence is superimposed with the preliminary predictions from AF-ELM to generate the required modeling results. The effectiveness of the proposed model is verified and assessed by taking a real concrete dam as an example and comparing prediction performance with state-of-the-art models. The results show that AF-ELM performs better in displacement prediction compared with benchmark models, and the multi-scale REC can effectively identify the valuable information within the residual sequence. The proposed online monitoring model can more closely track the dynamic variations of displacement data, which provides a fire-new solution for dam behavior prediction and analysis.
更多查看译文
关键词
Dam health monitoring,Displacement prediction,Sequential learning,Residual-error correction,Sequence decomposition and reconstruction
AI 理解论文
溯源树
样例
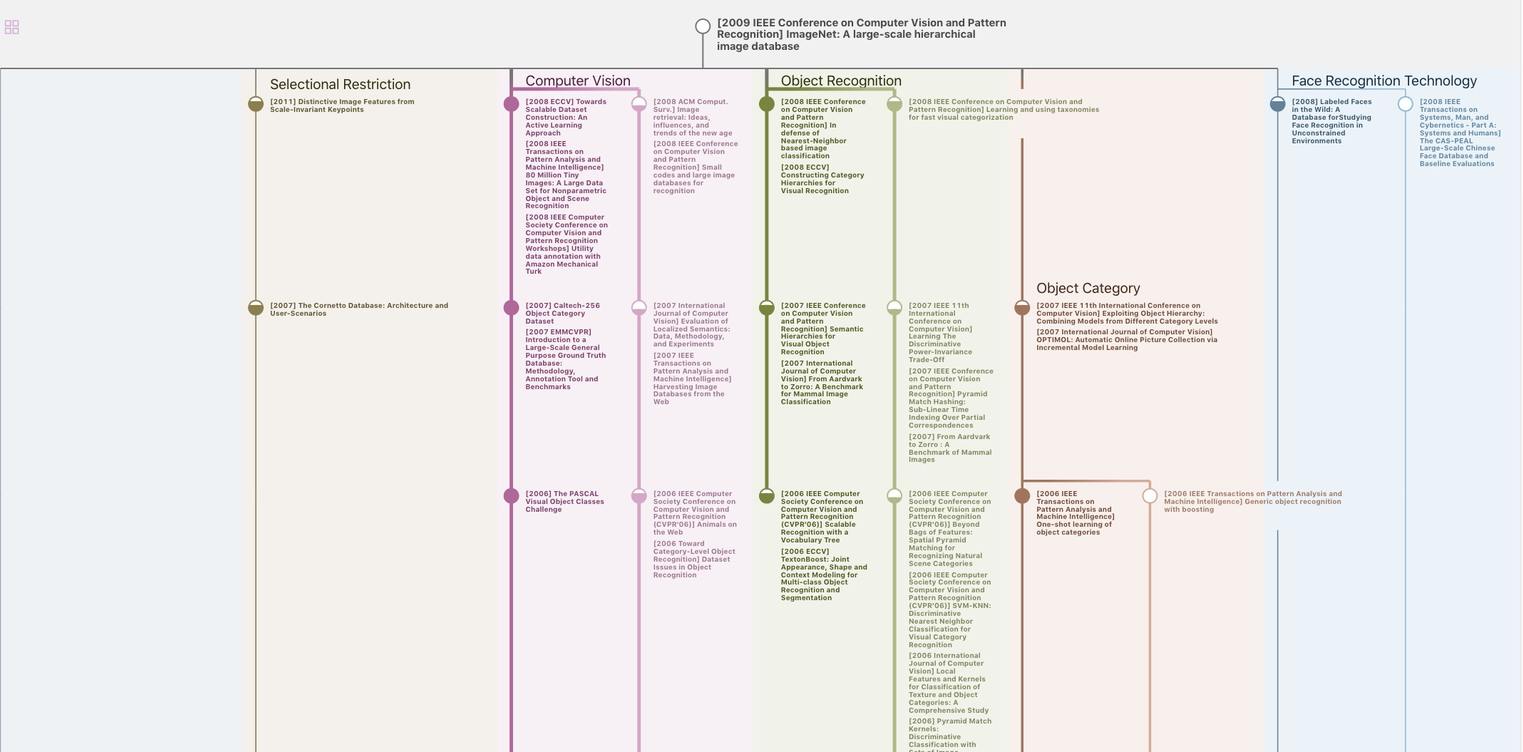
生成溯源树,研究论文发展脉络
Chat Paper
正在生成论文摘要