Bias Assessment Approaches for Addressing User-Centered Fairness in GNN-Based Recommender Systems.
Inf.(2023)
摘要
In today's technology-driven society, many decisions are made based on the results provided by machine learning algorithms. It is widely known that the models generated by such algorithms may present biases that lead to unfair decisions for some segments of the population, such as minority or marginalized groups. Hence, there is concern about the detection and mitigation of these biases, which may increase the discriminatory treatments of some demographic groups. Recommender systems, used today by millions of users, are not exempt from this drawback. The influence of these systems on so many user decisions, which in turn are taken as the basis for future recommendations, contributes to exacerbating this problem. Furthermore, there is evidence that some of the most recent and successful recommendation methods, such as those based on graphical neural networks (GNNs), are more sensitive to bias. The evaluation approaches of some of these biases, as those involving protected demographic groups, may not be suitable for recommender systems since their results are the preferences of the users and these do not necessarily have to be the same for the different groups. Other assessment metrics are aimed at evaluating biases that have no impact on the user. In this work, the suitability of different user-centered bias metrics in the context of GNN-based recommender systems are analyzed, as well as the response of recommendation methods with respect to the different types of biases to which these measures are addressed.
更多查看译文
关键词
recommender systems,GNN (graph neural network),bias amplification,fairness,sensitive features
AI 理解论文
溯源树
样例
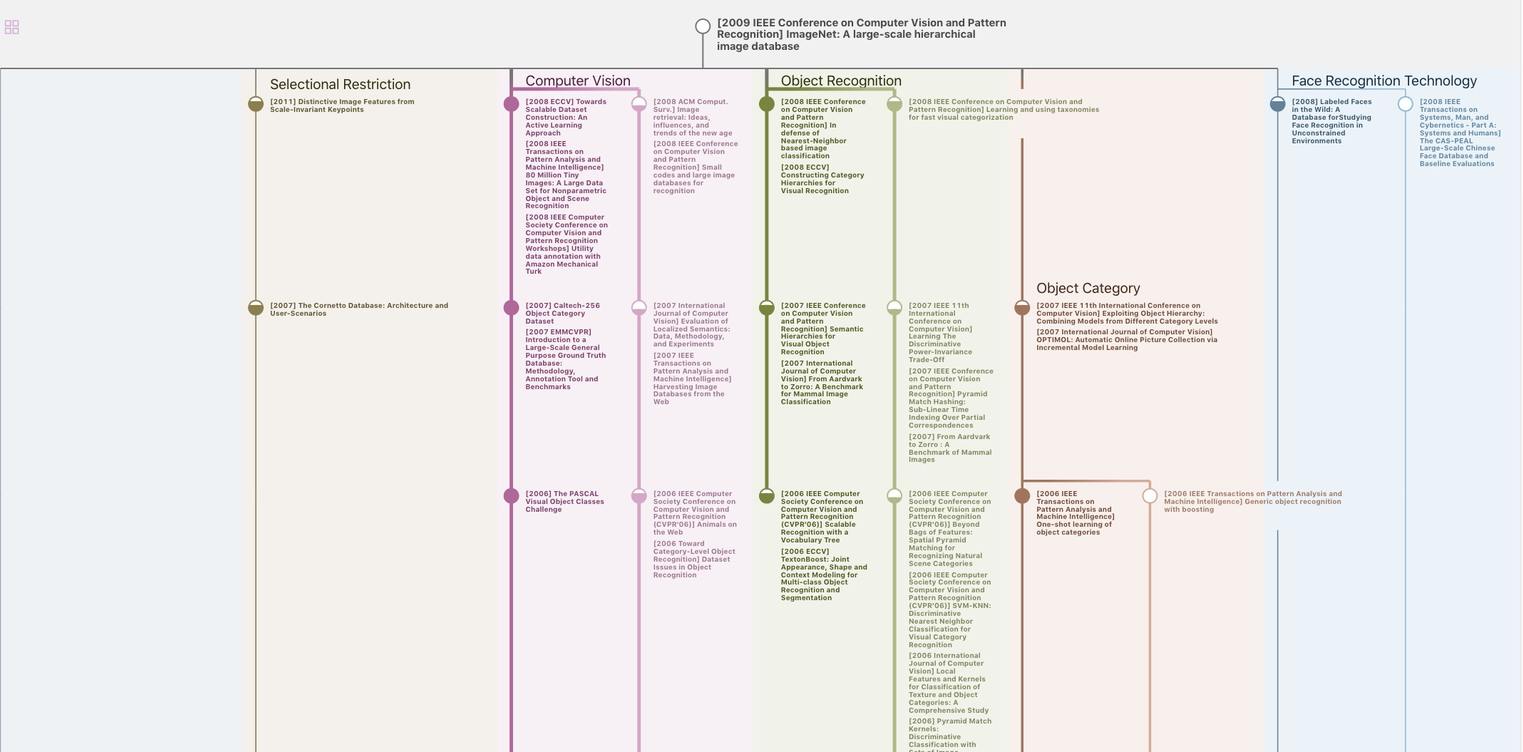
生成溯源树,研究论文发展脉络
Chat Paper
正在生成论文摘要