SURE: Screening Unlabeled Samples for Reliable Negative Samples Based on Reinforcement Learning.
INFORMATION SCIENCES(2023)
摘要
For many classification tasks, particularly in the bioinformatics field, only experimentally validated positive samples are available, and experimentally validated negative samples are not recorded. The lack of negative samples poses a challenge for using machine learning to perform such tasks. To address this problem, we propose a novel deep reinforcement learning-based model to screen reliable negative samples from unlabeled samples, named SURE. The model has two modules: a sample selector and a sample inspector. The sample selector screens reliable negative samples from unlabeled samples by two reinforcement strategies (learn to identify positive samples and reduce sample noise) and feeds the screened samples into the sample inspector. The sample inspector classifier provides rewards to the sample selector. The two modules are trained together to optimize the sample selector and sample inspector strategies. In this paper, we focus on one popular issue in the bioinformatics field: the ncRNA-protein interaction (NPI) prediction task, which lacks reliable negative samples. Thirty datasets for NPI prediction are used to test the screening effect of SURE. The experimental results show that our model has a robust negative sample screening capability and is superior to all outstanding sample screening methods used in the NPI prediction task. In addition, we refine 5 NPI datasets containing reliable negative samples screened by SURE, and a web server (ww.csbg-jlu.info/sure) is available offering the NPI prediction refined by SURE.
更多查看译文
关键词
Negative sample screening,Deep reinforcement learning,ncRNA-protein interaction
AI 理解论文
溯源树
样例
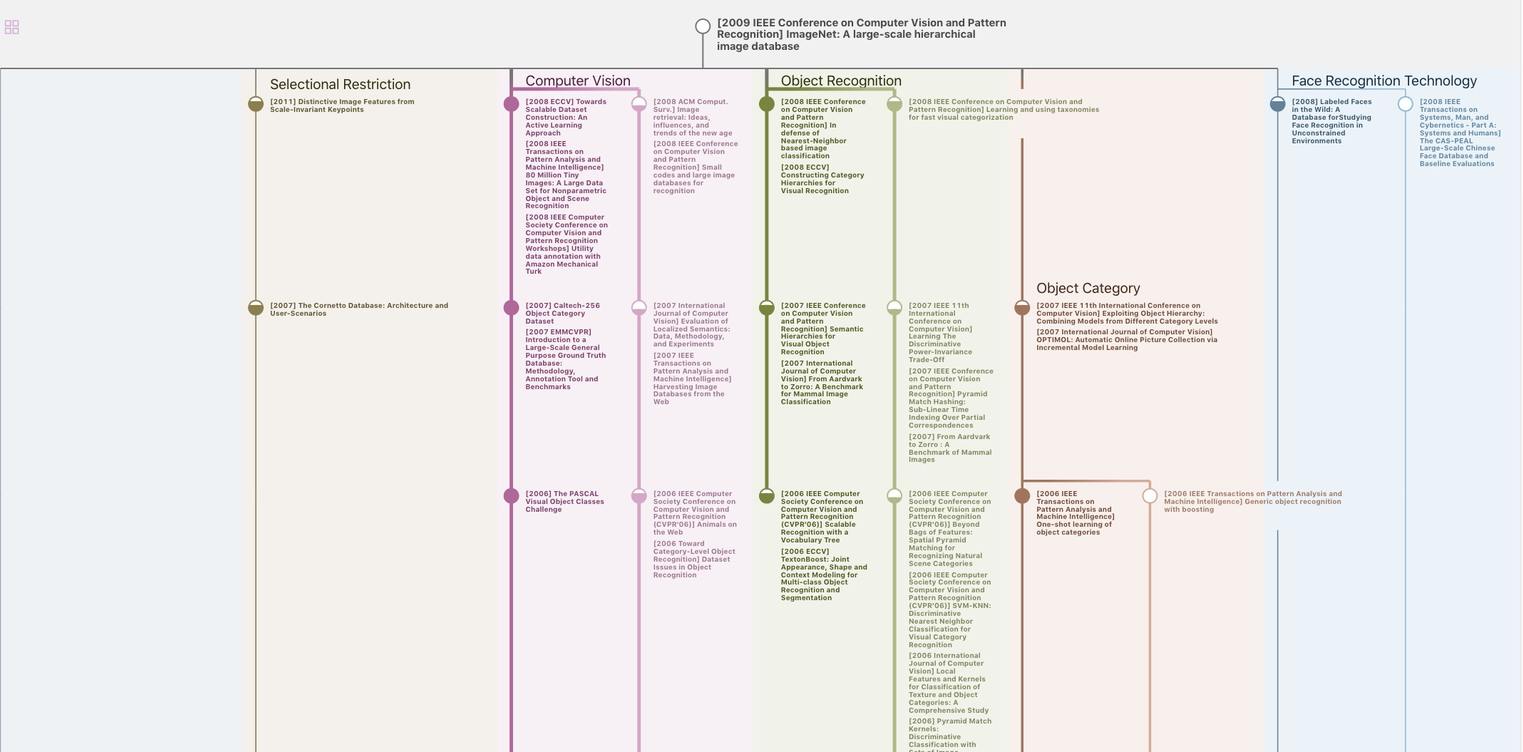
生成溯源树,研究论文发展脉络
Chat Paper
正在生成论文摘要