Fuzzy similarity phrases for interpretable data classification.
Inf. Sci.(2023)
摘要
Data classification is a core component of many intelligent systems, and it has been almost monopolizing the interest of the scientific community for decades. However, developing classifiers capable of explaining how a classification result was derived, in a way that is compatible to the human perception, is still a challenge. Steps towards this direction have been made via interpretable fuzzy classifiers capable of extracting linguistically expressible rules from data. Motivated by the need for interpretable data classification, this study proposes a novel interpretable fuzzy classification framework based on Fuzzy Similarity Phrases (FSPs), aiming to provide an improved tradeoff between interpretability and classification performance. This framework incorporates the following novel components: a) a generic vocabulary-based feature extraction scheme which considers similarity features and fuzzy sets to construct FSPs; b) a rule generation methodology based on FSPs; c) an embedded feature selection methodology targeting to keep both the number of extracted rules and their length low; and an interpretable class prediction mechanism based on the interpretation of the FSPs. The experimental evaluation of the proposed framework on various publicly available datasets, in comparison with well-known and state-of-the-art classifiers, validate that it can provide a better average classification performance with fewer rules.
更多查看译文
关键词
Fuzzy data representation,Feature extraction,Classification,Interpretable fuzzy rules,Fuzzy similarity phrases
AI 理解论文
溯源树
样例
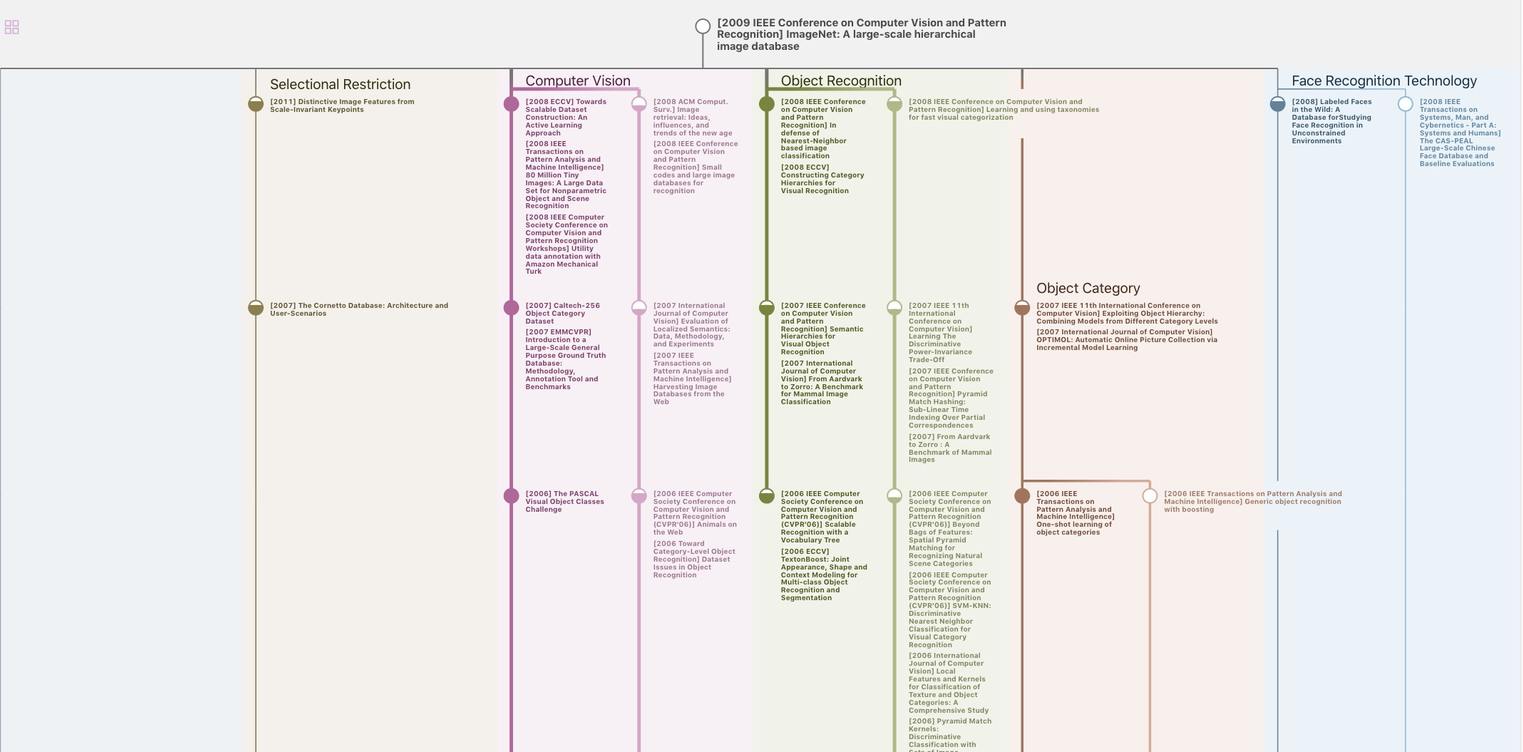
生成溯源树,研究论文发展脉络
Chat Paper
正在生成论文摘要