Supervised Machine Learning and Detection of Unknown Attacks: An Empirical Evaluation.
AINA (2)(2023)
摘要
Intrusion Detection Systems (IDS) have become one of the organizations’ most important security controls due to their ability to detect cyberattacks while inspecting network traffic. During the last decade, IDS proposals have increasingly used machine learning techniques (ML-based IDS) to create attack detection models. As this trend gains traction, researchers discuss whether these IDS can detect unknown attacks. Most ML-based IDS are based on supervised learning, which means they are trained with a limited collection of attack examples. Therefore, detecting attacks that were not covered during the training phase could be challenging for these systems. This work evaluates the ability of ML-based IDS to detect unknown attacks. Our general idea is to understand what happens when a detection model trained with a particular attack A receives incoming data from an unknown attack B. Using the CIC-IDS2017 dataset, we found that supervised intrusion detection models, in most cases, cannot detect unknown attacks. The only exception occurs with DoS attacks. For example, an intrusion detection model trained with HTTP Flood DoS (GoldenEye) samples could detect a different HTTP DoS attack type (Slowloris).
更多查看译文
关键词
unknown attacks,machine learning,empirical evaluation,detection
AI 理解论文
溯源树
样例
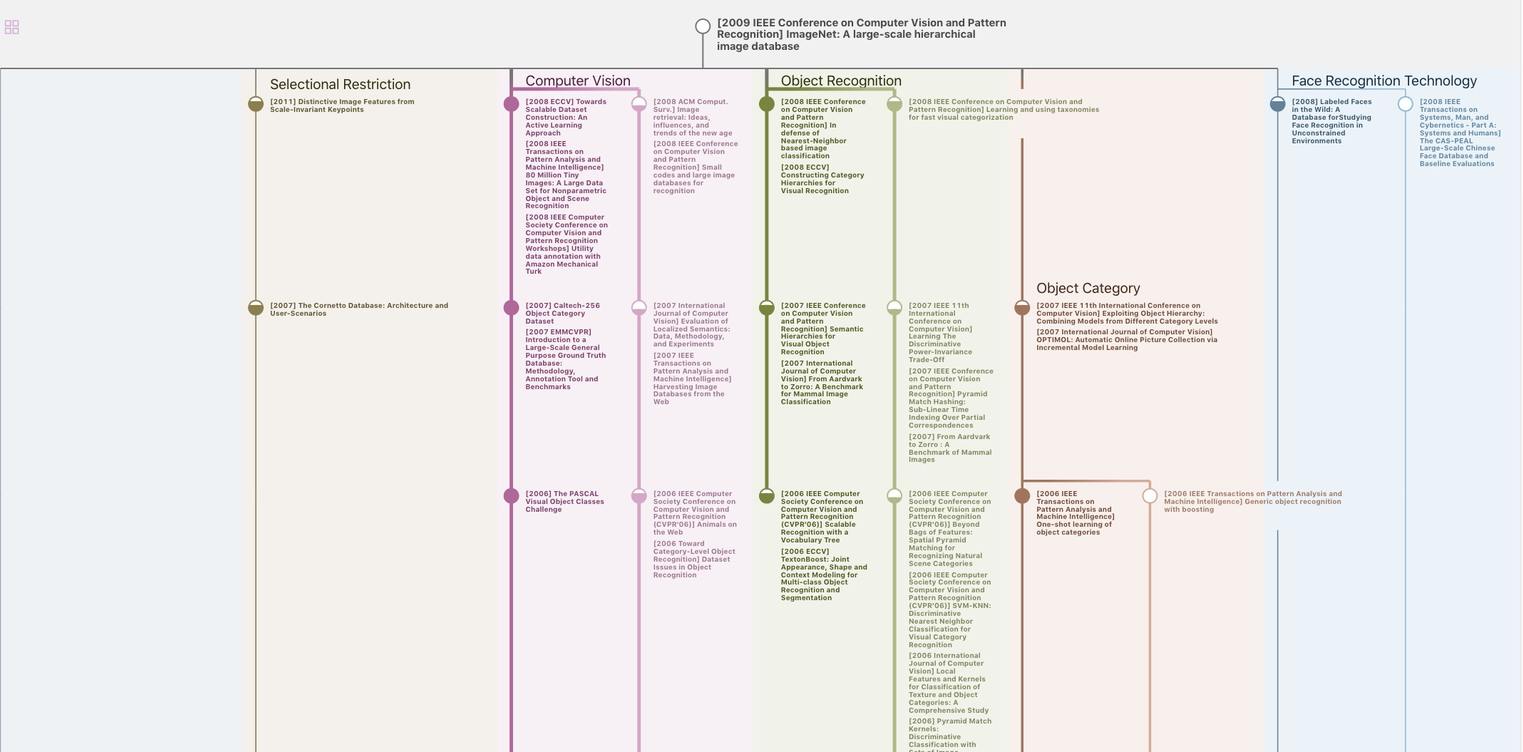
生成溯源树,研究论文发展脉络
Chat Paper
正在生成论文摘要