Semi-supervised Multi-task Learning with Auxiliary data
Inf. Sci.(2023)
摘要
Compared with single-task learning, multi-tasks can obtain better classifiers by the information provided by each task. In the process of multi-task data collection, we always focus on the target task data in the training process, and ignore the non-target task data and unlabeled data that may be contained in the target task. In response to this issue, this paper introduces auxiliary or Universum into semi-supervised multi-task problem, and proposes a multi-task support vector machine (SU-MTLSVM) method based on semi-supervised learning to handle the case where each task contains the labeled, unlabeled, and Universum samples in the training set. This method introduces Universum as prior knowledge and provides high-dimensional information for semi-supervised learning, and builds a unique classifier from a large amount of unlabeled data. We then use KKT conditions and Lagrangian method to optimize the formulation of the model, and get the model parameters. Finally, we collect different data sets in the experiment part, and compare the performance of multiple baselines with the proposed method. Experiments prove that the method proposed in this paper is more effective for multi-task applications.
更多查看译文
关键词
Multi-task learning,Universum
AI 理解论文
溯源树
样例
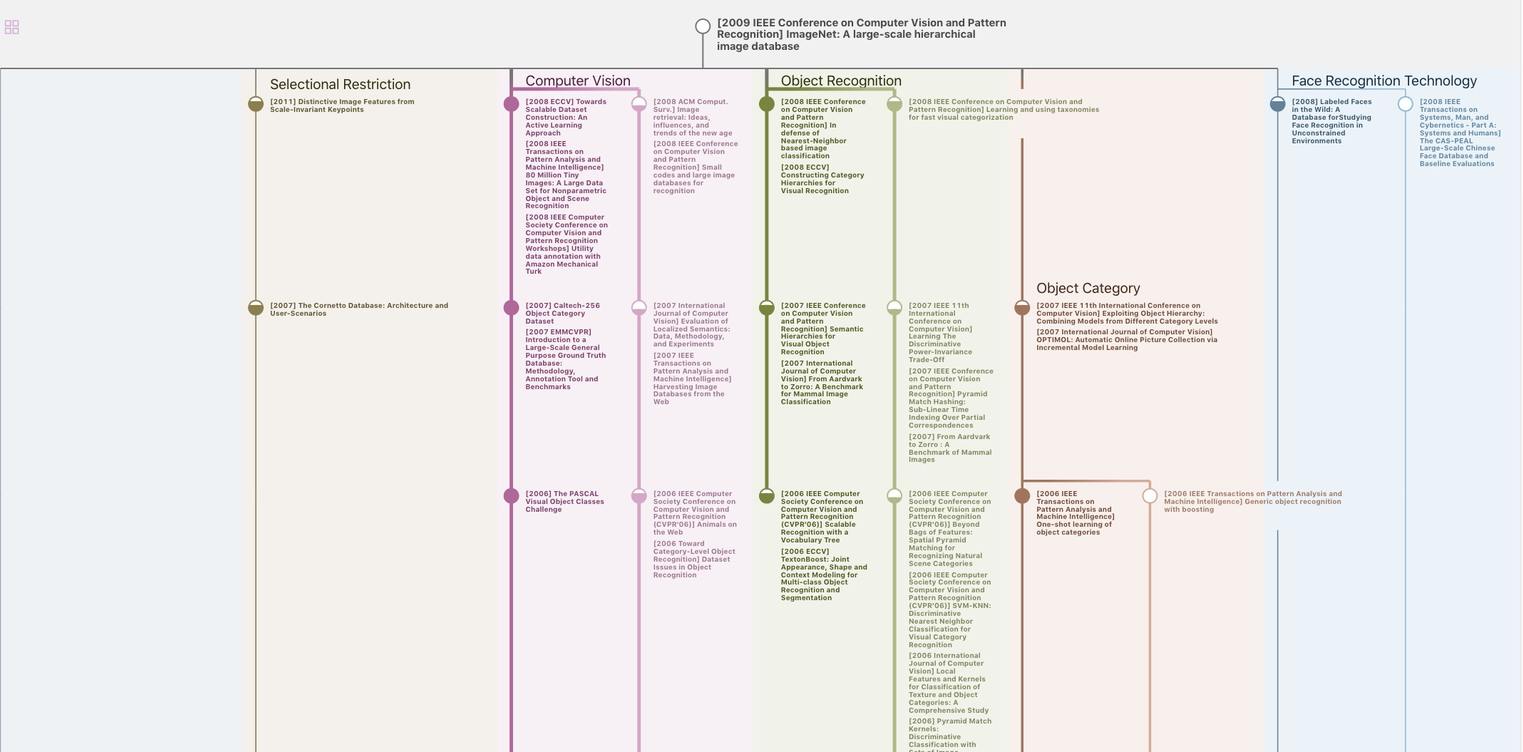
生成溯源树,研究论文发展脉络
Chat Paper
正在生成论文摘要