Online training data acquisition for federated learning in cloud-edge networks.
Comput. Networks(2023)
摘要
Federated learning (FL) is an effective approach to exploiting different data sources for collaborative model training while maintaining the privacy of users. Adequate data is necessary to improve the accuracy of the federated learning. However, it is difficult for all data sources to acquire sufficient data for each iteration of federated training. In this paper, we study the data acquisition problem in cloud-edge networks, where edges acquire data from users and conduct the local training while the cloud aggregates the local models. Since data acquired by users with uncertainty, it is not easy to make the data acquisition decision for the FL online. Even worse, due to the unknown cost of data, it is difficult to make a macro-timescale decision. We propose a two-timescale online scheduling for federated learning to confront the uncertainty of the data acquisition. By learning empirical state information of the system with a carefully designed Lyapunov virtual queue and coordinating the data acquisition in different timescales in an online manner, the proposed approach minimizes the data acquisition cost of federated learning, reduces the data transmission delay and accelerates the convergence speed of federated learning. Rigorous theoretical analysis shows strong performance guarantees of the proposed two-timescale Lyapunov optimization algorithm and extensive trace-driven experimental results suggests that the algorithm achieves outstanding performance gains over existing benchmarks.
更多查看译文
关键词
federated learning,online training data acquisition,cloud–edge cloud–edge,networks
AI 理解论文
溯源树
样例
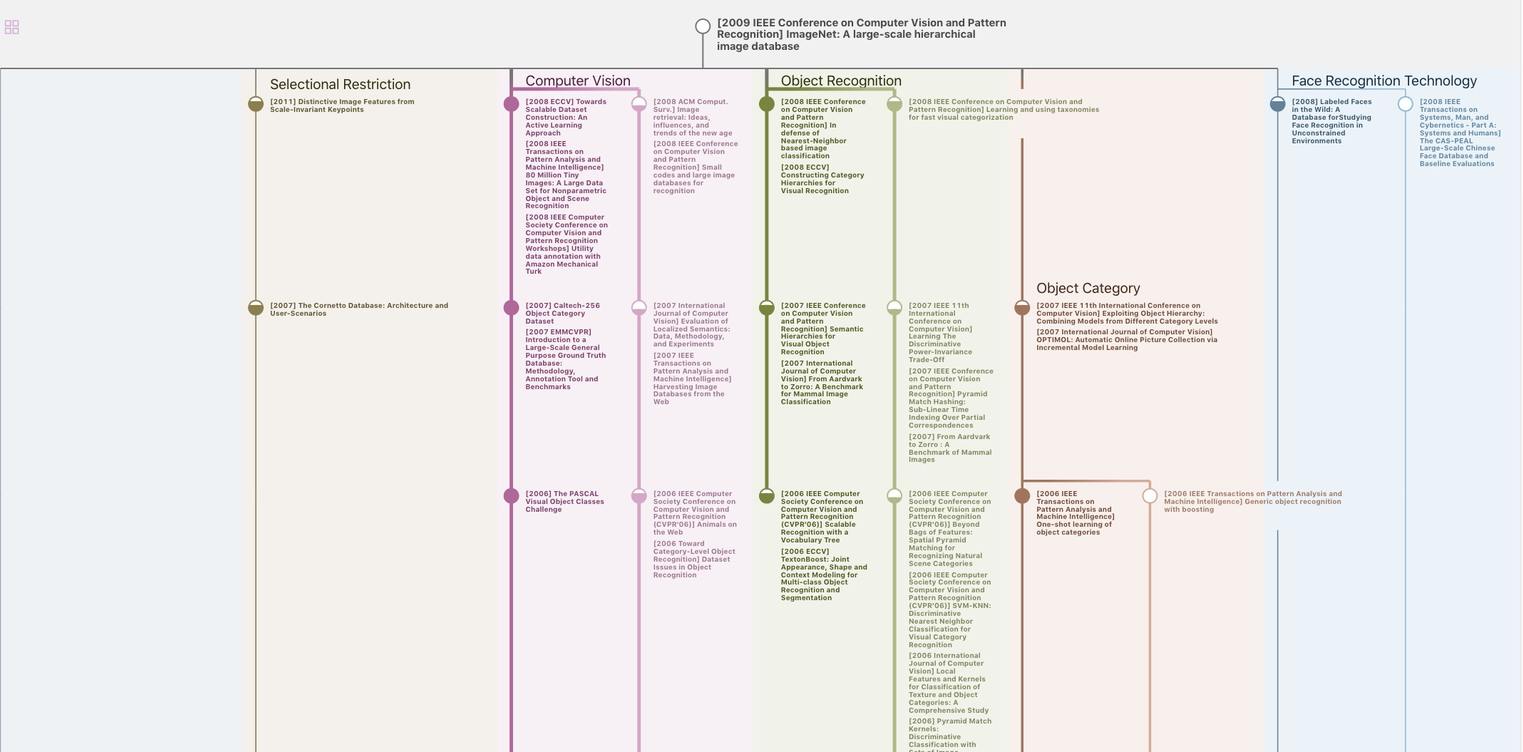
生成溯源树,研究论文发展脉络
Chat Paper
正在生成论文摘要