Identifying Dependent Annotators in Crowdsourcing.
IEEECONF(2022)
摘要
Crowdsourcing is the learning paradigm that aims to combine noisy labels provided by a crowd of human annotators. To facilitate this label fusion, most contemporary crowdsourcing methods assume conditional independence between different annotators. Nevertheless, in many cases this assumption may not hold. This work investigates the effects of groups of correlated annotators in multiclass crowdsourced classification. To deal with this setup, a novel approach is developed to identify groups of dependent annotators via second-order moments of annotator responses. This in turn, enables appropriate dependence aware aggregation of annotator responses. Preliminary tests on synthetic and real data showcase the potential of the proposed approach.
更多查看译文
关键词
Crowdsourcing, Weak supervision, Ensemble Learning, Classification
AI 理解论文
溯源树
样例
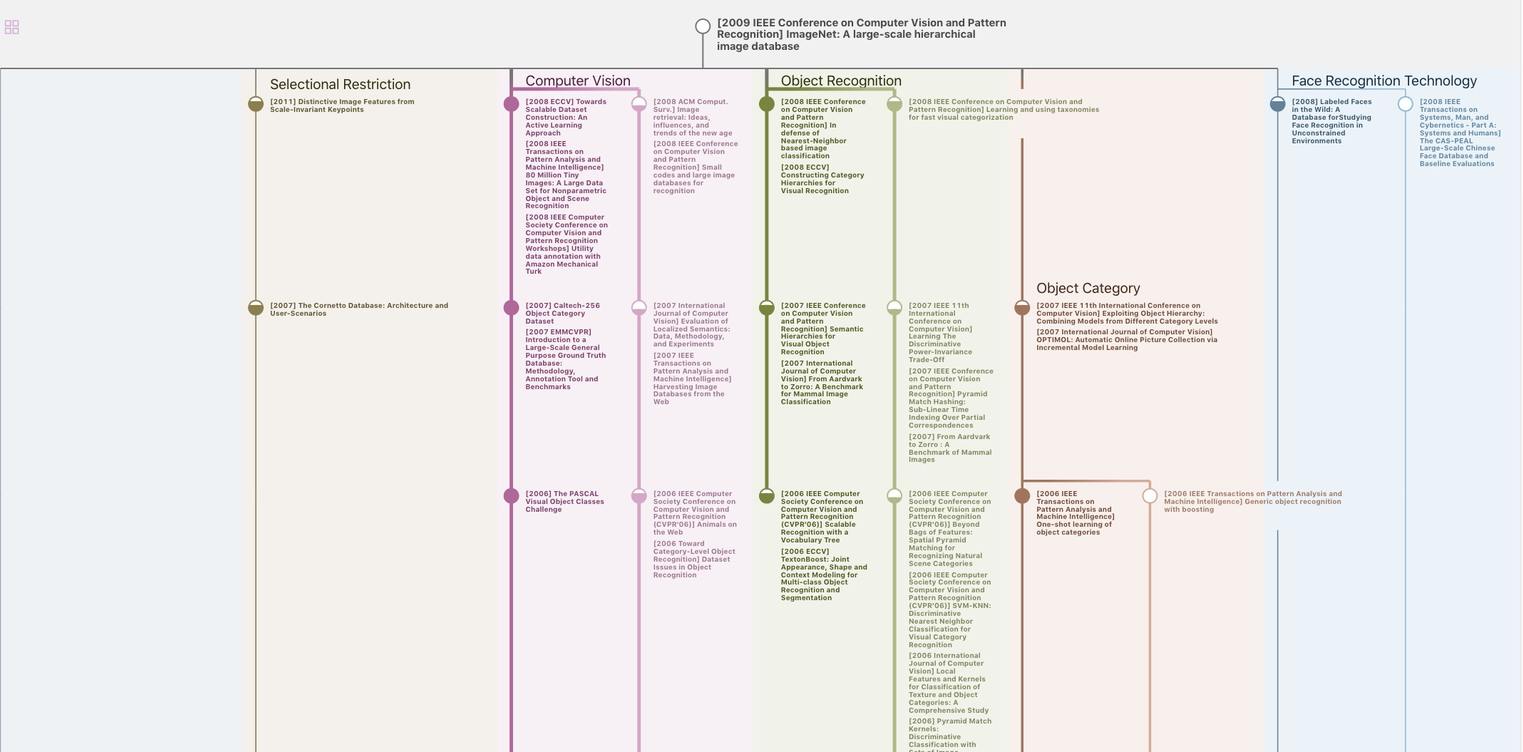
生成溯源树,研究论文发展脉络
Chat Paper
正在生成论文摘要