Adaptive iterative extended state observer-based data-driven iterative learning model predictive control for semiconductor silicon single crystal batch process
JOURNAL OF THE FRANKLIN INSTITUTE-ENGINEERING AND APPLIED MATHEMATICS(2023)
摘要
Aiming at the problems of unstable batch control of key crystal quality parameters and susceptibility to batch-to-batch non-repetitive disturbances during repeated operation of single crystal furnaces, this paper proposes a data-driven iterative learning model predictive control method based on an adaptive iterative extended state observer (IESO) for designing melt temperature and crystal diameter learning controllers with disturbance suppression. By applying dynamic linearization techniques and model pre-dictive control strategies along the iterative axis, an ILMPC scheme with disturbance compensation terms using only input and output data of the system is designed. Among them, adaptive IESO is used to estimate the disturbance compensation terms. Then, the theoretical analysis shows that the tracking error of the ILMPC scheme can converge to a bounded range as the number of iterations increases. The experimental results verify the effectiveness of the proposed control method, which not only ensures that the control system has learning ability, but also achieves stable and accurate control of crystal quality parameters. (c) 2023 The Franklin Institute. Published by Elsevier Inc. All rights reserved.
更多查看译文
关键词
iterative learning model,predictive control,semiconductor silicon,adaptive,observer-based,data-driven
AI 理解论文
溯源树
样例
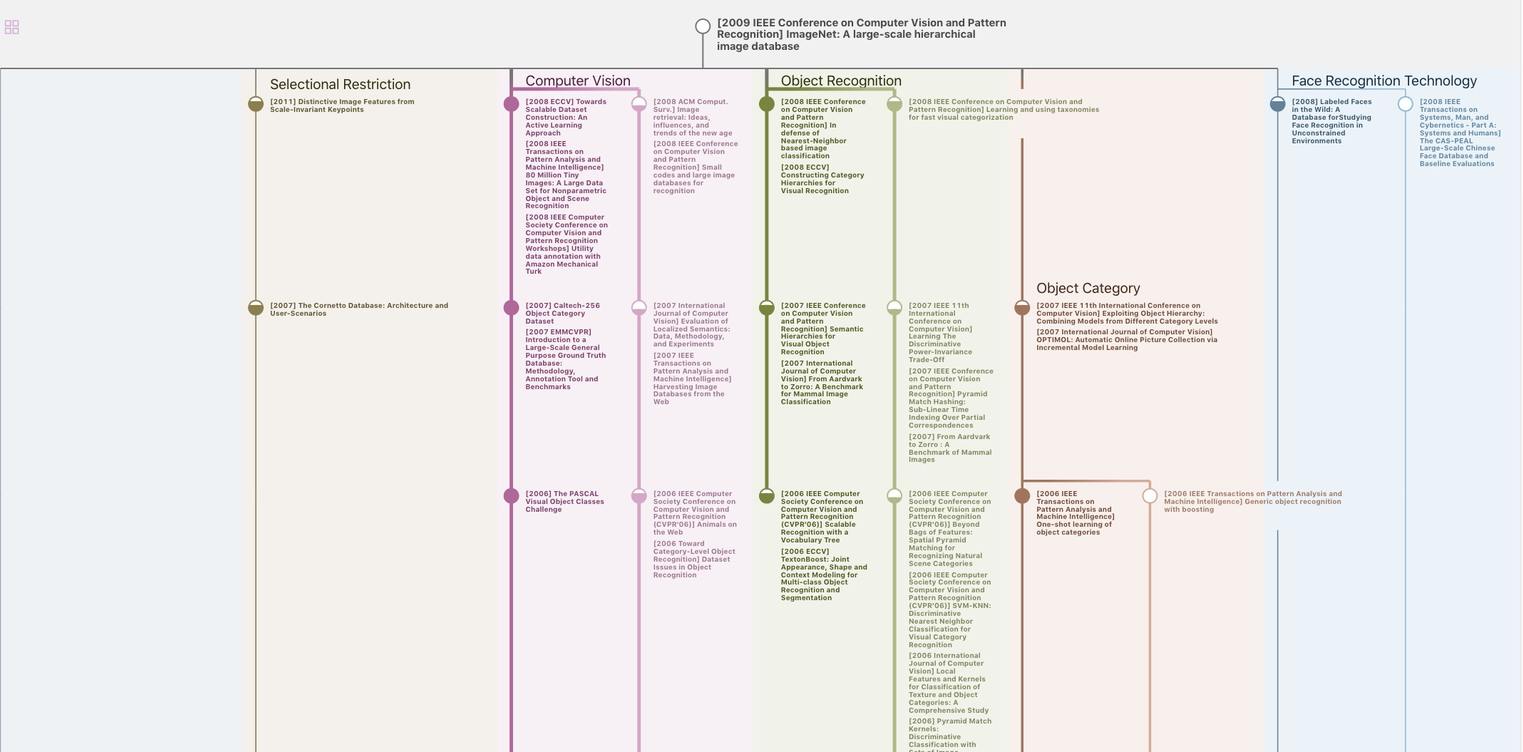
生成溯源树,研究论文发展脉络
Chat Paper
正在生成论文摘要