Public-attention-based Adversarial Attack on Traffic Sign Recognition
2023 IEEE 20TH CONSUMER COMMUNICATIONS & NETWORKING CONFERENCE, CCNC(2023)
摘要
Autonomous driving systems (ADS) can instantaneously and accurately recognize traffic signs by using deep neural networks (DNNs). Although adversarial attacks are well-known to easily fool DNNs by adding tiny but malicious perturbations, most attack methods require sufficient information about the victim models (white-box) to perform. In this paper, we propose a black-box attack in the recognition system of ADS, Public Attention Attacks (PAA), that can attack a black-box model by collecting the generic attention patterns of other white-box DNNs to transfer the attack. Particularly, we select multiple dual or triple attention patterns of white-box model combinations to generate the transferable adversarial perturbations for PAA attacks. We perform the experimentation on four well-trained models in different adversarial settings separately. The results indicate that when more white-box models the adversary collects to perform PAA, the higher the attack success rate (ASR) he can achieve to attack the target black-box model.
更多查看译文
关键词
Adversarial attack,attention heat map,trans-ferability,deep neural networks,traffic sign recognition
AI 理解论文
溯源树
样例
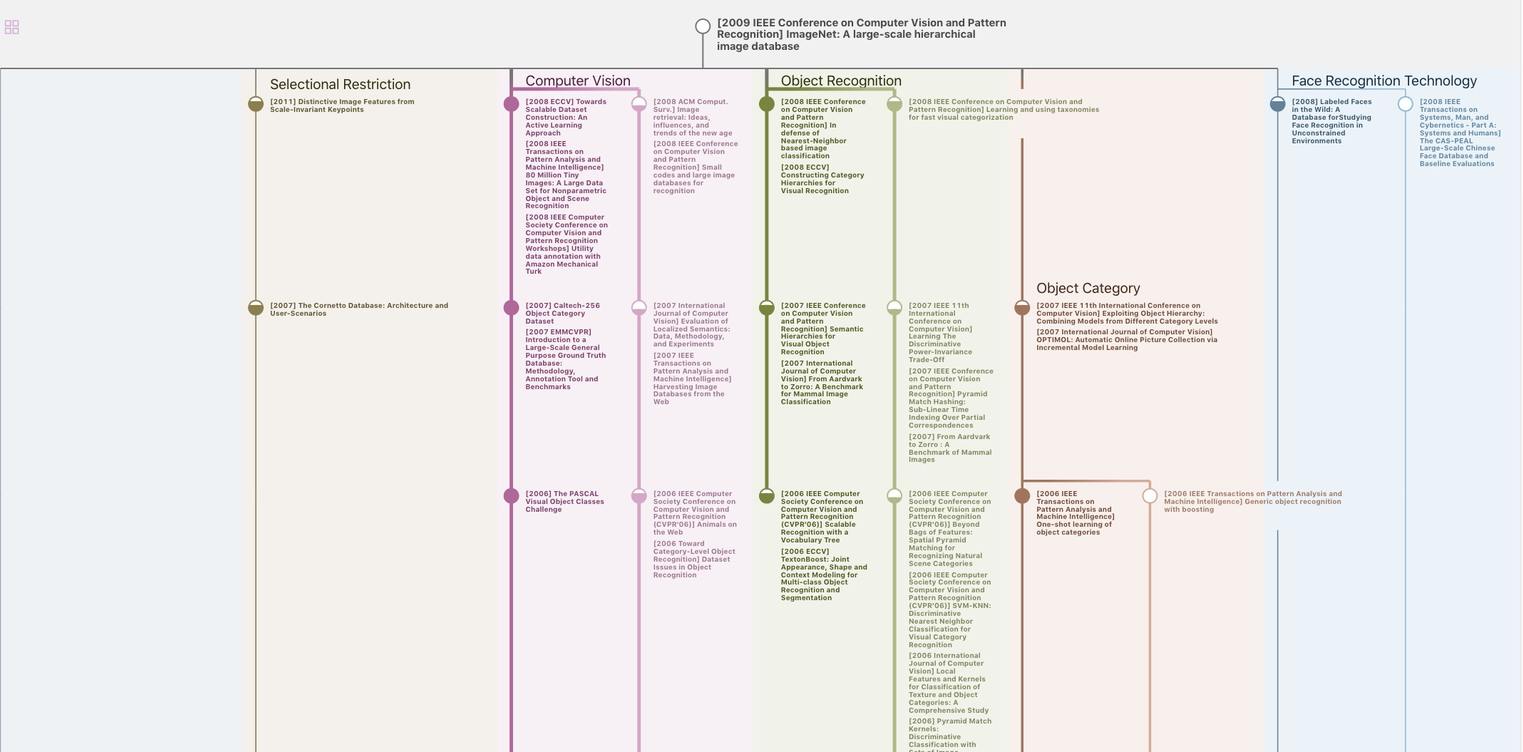
生成溯源树,研究论文发展脉络
Chat Paper
正在生成论文摘要