Off-policy reinforcement learning for tracking control of discrete-time Markov jump linear systems with completely unknown dynamics.
J. Frankl. Inst.(2023)
摘要
In this paper, a model-free off-policy reinforcement learning (RL) algorithm is proposed to address the optimal tracking control (OTC) problem for discrete-time Markov jump linear systems (MJLSs). The tracking reference signal is firstly augmented into discrete-time MJLSs, whereby the original tracking control problem is converted to the optimal control problem of the augmented system. The corresponding augmented coupled game algebraic Riccati equation (ACGARE) is then derived. On this basis, an online RL algorithm is developed to solve the OTC problem by using the policy iteration (PI) technique. Then, a novel model-free method is proposed, which eliminates the requirement of the system dynamics and transition probability. Finally, a simulation example is provided to prove the convergence and validate the effectiveness of the proposed algorithm.
更多查看译文
关键词
linear systems,control,off-policy,discrete-time
AI 理解论文
溯源树
样例
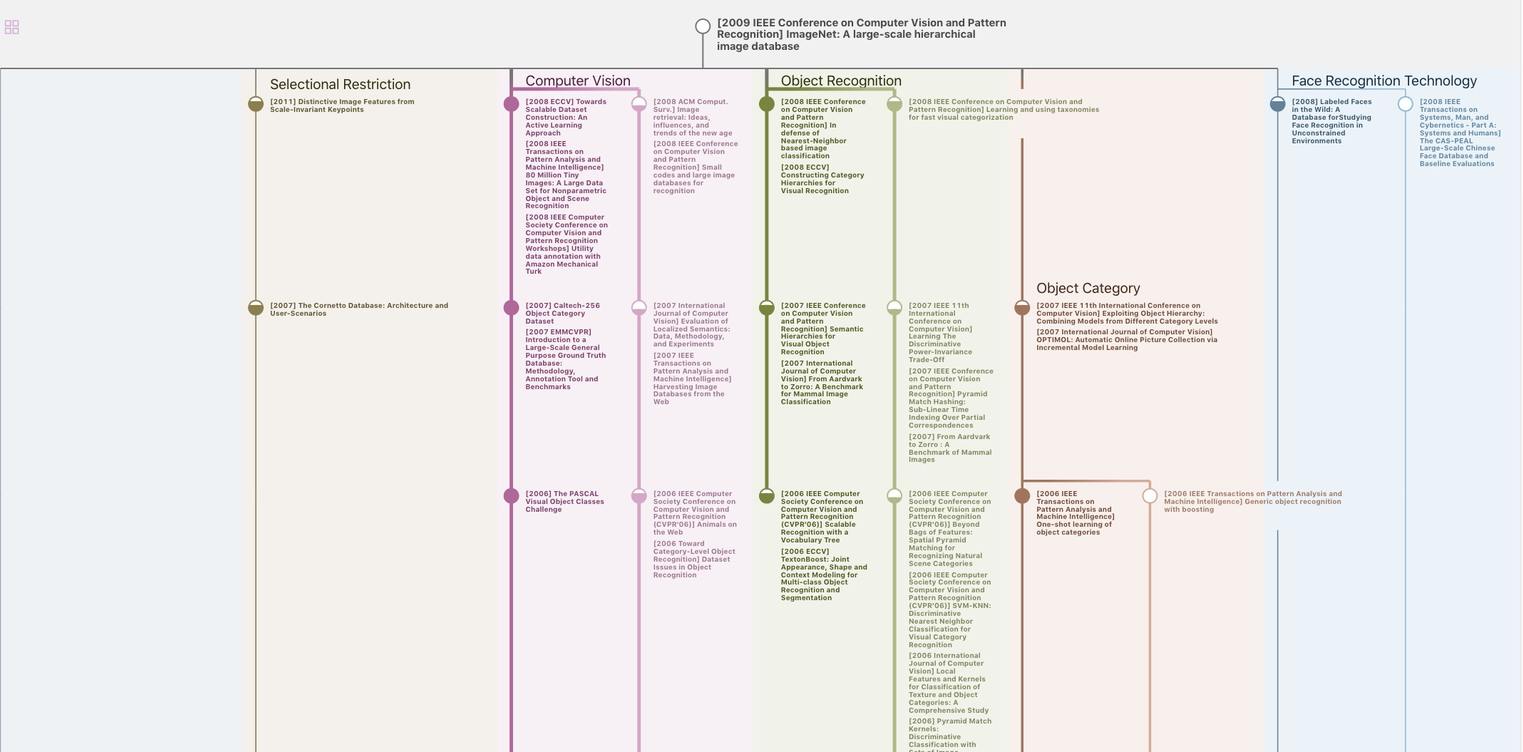
生成溯源树,研究论文发展脉络
Chat Paper
正在生成论文摘要