Robot-Network Co-optimization Using Deep Reinforcement Learning.
CCNC(2023)
摘要
The evolution of a cell phone network and a wireless local area network (LAN) has enabled various devices to be connected to wireless networks anytime, anywhere. This has led to the emergence of various applications, such as automatic transportation of parts and cargo by automated guided vehicles, remote control of unmanned vehicles, and drones. It will be a challenge to maintain high-quality connections, which satisfy the requirements of such emerging applications, for a large number of intelligent connected devices. To improve the network performance, we must control the wireless network setting and the behavior of the connected vehicles, i.e., robots. From this perspective, this proposes a novel framework, CoRein, that achieves simultaneous optimization of robot behavior and wireless network setting. In contrast to the existing studies, CoRein provides optimal actions for robots and wireless networks using deep reinforcement learning (DRL) architecture. To the best of our knowledge, our study firstly attempts to solve different tasks corresponding to robots and networking with a unique DRL architecture. To evaluate the effectiveness of CoRein, we considered a scenario where two robots were moving back and forth while communicating with one of two access points. CoRein calculates the robot velocities and AP selection. We carried out simulations and indoor experiments with our robot testbeds to evaluate the network performances. Evaluation results confirmed that the two robots adjusted their positions and the access points to increase the wireless network's performance, i.e., throughput and round trip time while changing the speed of their movements.
更多查看译文
关键词
Robot,Deep Reinforcement Learning
AI 理解论文
溯源树
样例
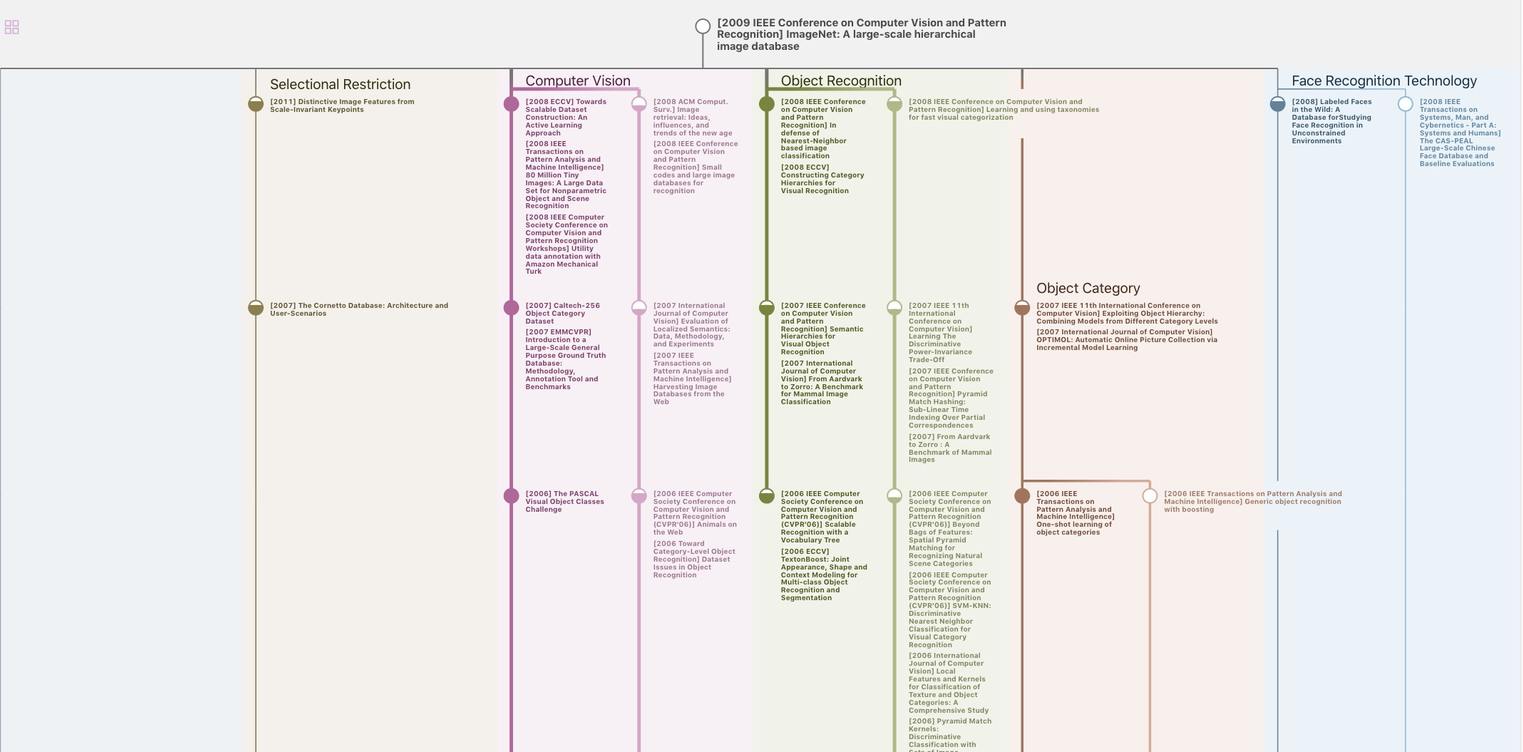
生成溯源树,研究论文发展脉络
Chat Paper
正在生成论文摘要