MATTE: Multi-task multi-scale attention.
Comput. Vis. Image Underst.(2023)
摘要
In this work, we propose a general method for learning task and scale based attention representations in Multi-Task Learning (MTL) for vision. It relies on learning and maintaining cross-task and cross-scale representations of visual information, whose interaction contributes to a symmetrical improvement across the entire task pool. Apart from learning data representations, we additionally optimize for the most beneficial interaction between tasks and their representations at different scales. Our method adds an attention modulated feature as residual information to the processing of each scale stage within the model, including the final layer of task outputs. We empirically show the effectiveness of our method through experiments with current multi-modal and multi-scale architectures on diverse MTL datasets. We evaluate MATTE on high and low level vision MTL problems, against MTL and single task learning (STL) counterparts. For all experiments we report solid performance improvements in both qualitative and quantitative performance.
更多查看译文
关键词
attention,multi-task,multi-scale
AI 理解论文
溯源树
样例
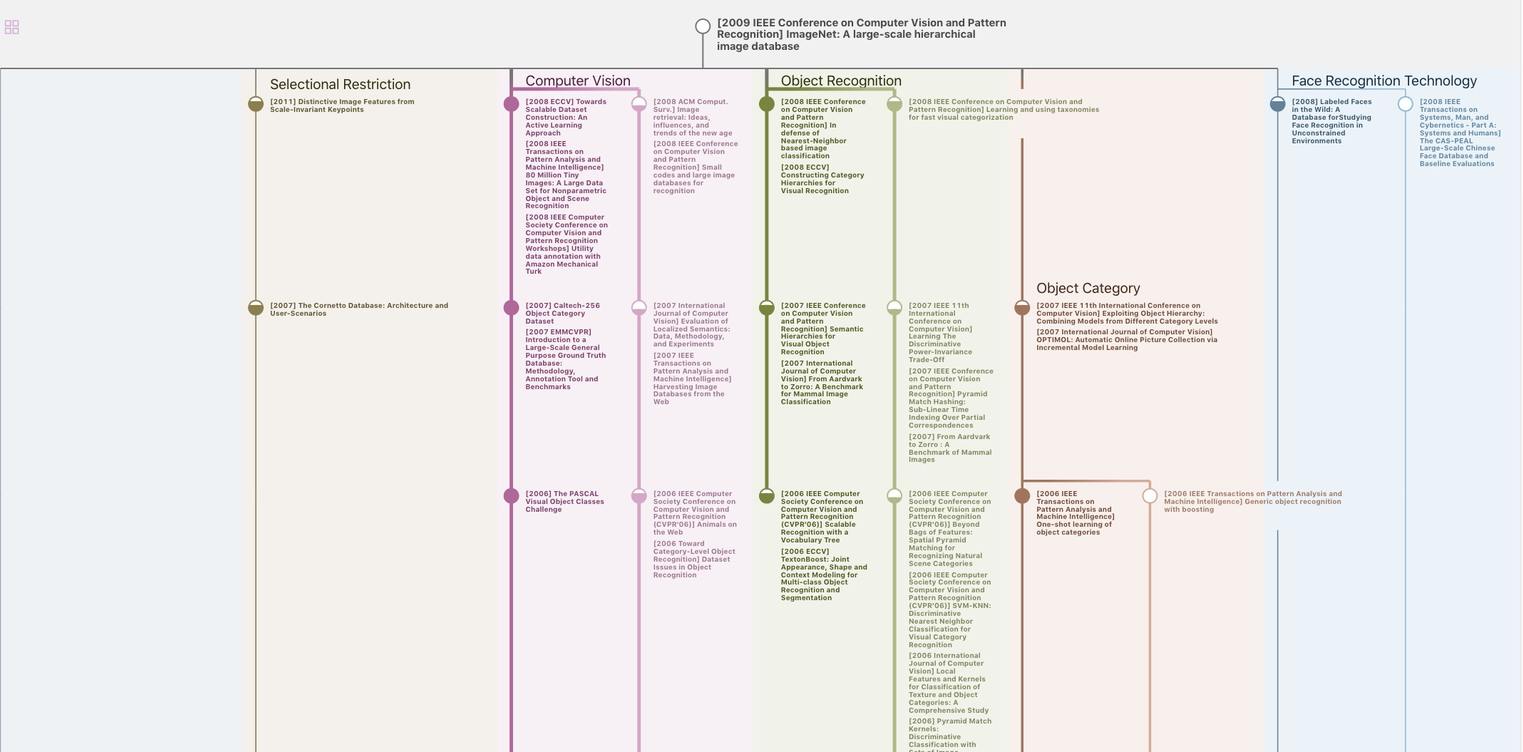
生成溯源树,研究论文发展脉络
Chat Paper
正在生成论文摘要