Wasserstein Distance-Based Full-Waveform Inversion With a Regularizer Powered by Learned Gradient
IEEE Trans. Geosci. Remote. Sens.(2023)
摘要
Full-waveform inversion (FWI) is a powerful technique for building high-quality subsurface geological structures. It is known to suffer from local minima problems when a good starting model is lost. To obtain a desirable solution, regularization constraints are needed to impose suitable priors, in particular for salt models. Recent studies have allowed cooperating the denoising operator as a specific prior with optimization algorithms to effectively solve various image reconstruction tasks. Inspired by the promising performance of regularization by denoising (RED), we propose a flexible unsupervised learning FWI framework, in which the regularizer is an extensive variant of RED powered by learned gradient for addressing salt models’ inversion. To further mitigate local minima issues, the Wasserstein distance induced by the optimal transport (OT) theory with a new preprocessing transformation is applied as a measure in data domain. Integrating the physical constraints featured by the wave equation and the model priors featured by the deep convolutional neural network (CNN), our method is able to produce measurement consistent and high-resolution results. We experimentally compare the proposed method with the traditional total variation regularized FWI and RED regularized FWI on the well-known geological models. The numerical results demonstrate the effectiveness of our method for handling the inversion task of high-contrast media in the presence of a free-surface case. Moreover, our framework is adaptive to restrict the solutions by leveraging the regularizer with learned gradient, whose training does not need any geological images, thus paving the way to develop FWI algorithms with state-of-the-art deep learning (DL) techniques in interdisciplinary research.
更多查看译文
关键词
regularizer powered,distance-based,full-waveform
AI 理解论文
溯源树
样例
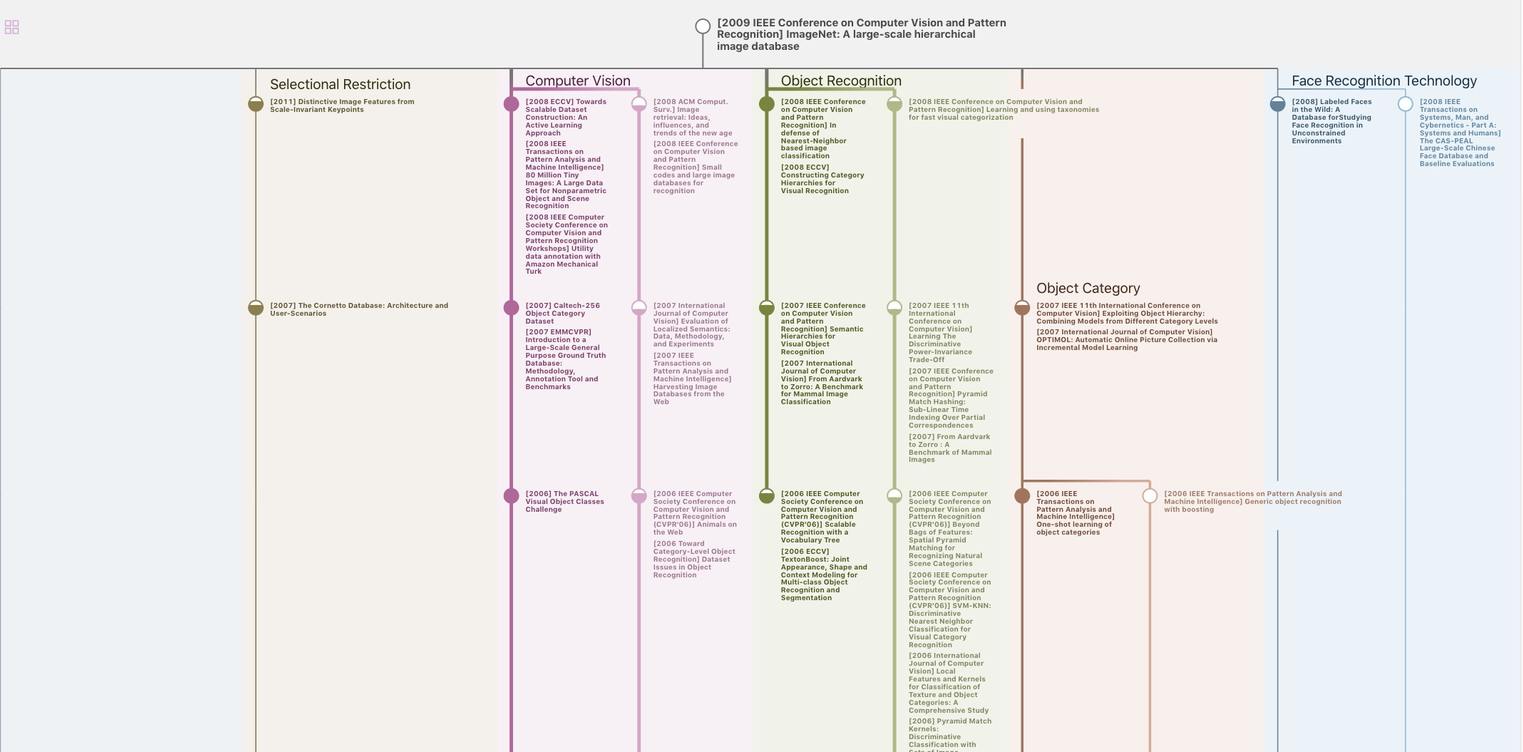
生成溯源树,研究论文发展脉络
Chat Paper
正在生成论文摘要