Visual Enhancement and Semantic Segmentation of Murine Tissue Scans with Pulsed THz Spectroscopy.
ICSC(2023)
摘要
Semantic Artificial Intelligence has certain qualities that are advantageous for deep learning-based medical imaging tasks. Medical images can be augmented by injecting semantic context into the underlying classification mechanism, increasing the information density of the scan and ultimately can provide more trust in the result. This work considers an application of semantic AI to segment tissue types from excised breast tumors imaged with pulsed terahertz (THz)–an emerging imaging technology. Prior work has demonstrated traditional data driven methodology for deep learning on THz has two key weaknesses: namely 1) low image resolution compared to other state-of-the-art imaging techniques and 2) a lack of expertly-labeled images due to domain transformation and tissue changes during histopathology. This work seeks to address these limitations through two semantic AI mechanisms. Specifically, we introduce a two stage pipeline using an unsupervised image-to-image translation network and a supervised segmentation network. The combination of these contributions enables enhanced near-real-time visualization of excised tissue through THz scans and a supervised segmentation and classification training strategy using only synthetic THz scans generated by our bi-directional image-to-image translation network.
更多查看译文
关键词
deep learning,image translation,semantic segmentation,pulsed terahertz imaging,breast cancer imaging
AI 理解论文
溯源树
样例
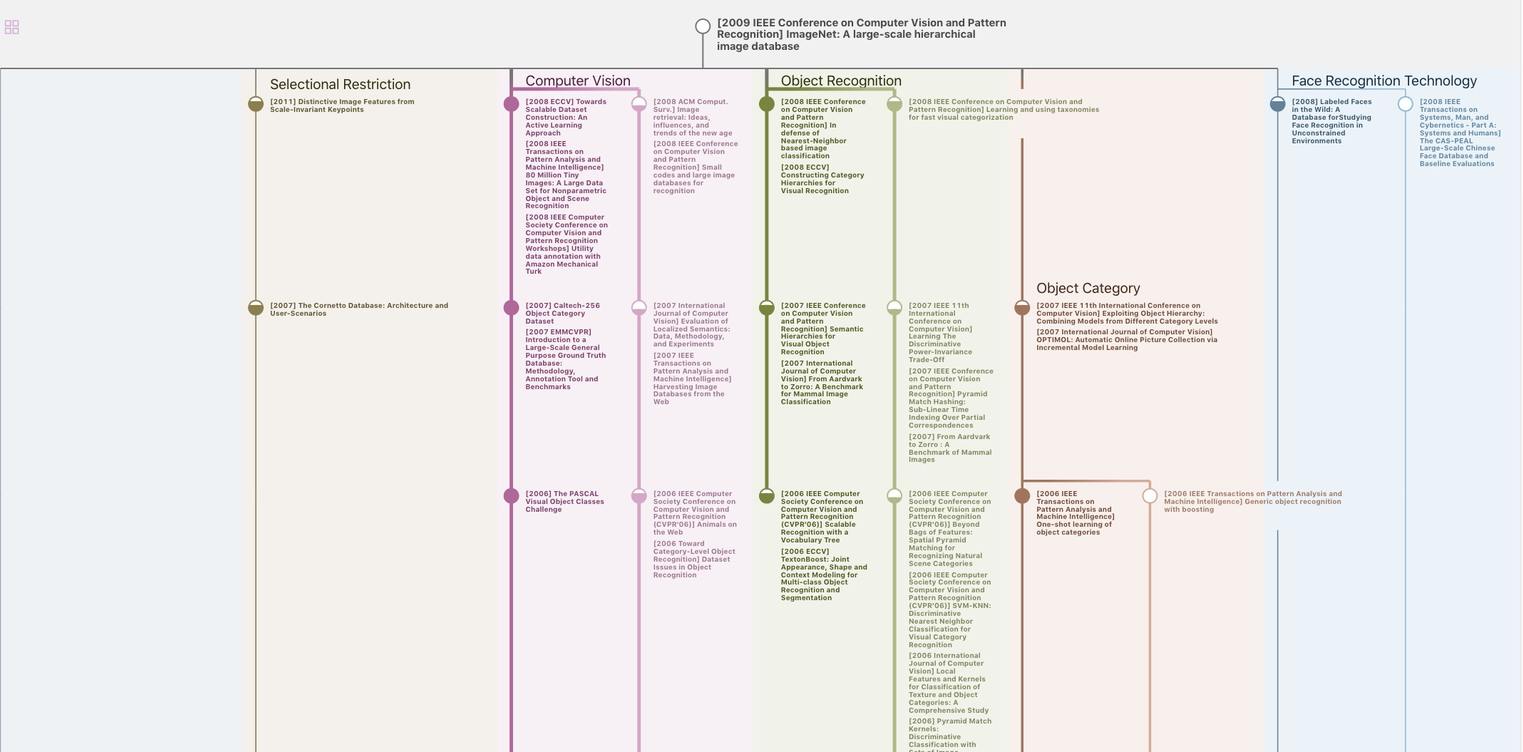
生成溯源树,研究论文发展脉络
Chat Paper
正在生成论文摘要