HRLE-SARDet: A Lightweight SAR Target Detection Algorithm Based on Hybrid Representation Learning Enhancement.
IEEE Trans. Geosci. Remote. Sens.(2023)
摘要
In recent years, deep learning has been widely used in remote sensing, especially in the field of synthetic aperture radar (SAR) image target detection. However, all of these deep learning models continue increasing the network's depth and width without maintaining a good balance between accuracy and speed. Therefore, in this article, we propose a hybrid representation learning-enhanced SAR target detection algorithm based on the unique features of SAR images from a lightweight perspective called HRLE-SARDet. First, we design a lightweight and scattering feature extraction backbone that is more suitable for SAR image data. Second, for the multiscale feature discrepancy, we design a new multiscale feature fusion neck. Next, to better extract the scattering information from small targets of SAR images and improve the detection accuracy, we design a lightweight hybrid representation learning enhancement module. Finally, to better fit target detection for SAR image datasets, we redesign a more flexible loss function, which allows for an easy adjustment of the importance of polynomial bases according to the target task and dataset. Extensive experimental results on three SAR image ship target datasets (SSDD, AIR-SARShip-2.0, and HRSID) and a newly released large multiclass target SAR dataset (MSAR-1.0) show that our HRLE-SARDet achieves 98.4%, 79.2%, 92.5%, and 88.4% mean average precision (mAP) with only 1.09 M parameters and 2.5 G floating-point operations (FLOPs) on the SSDD, AIR-SARShip-2.0, HRSID, and MSAR-1.0 datasets, respectively, which is an excellent performance.
更多查看译文
关键词
detection,target,hrle-sardet
AI 理解论文
溯源树
样例
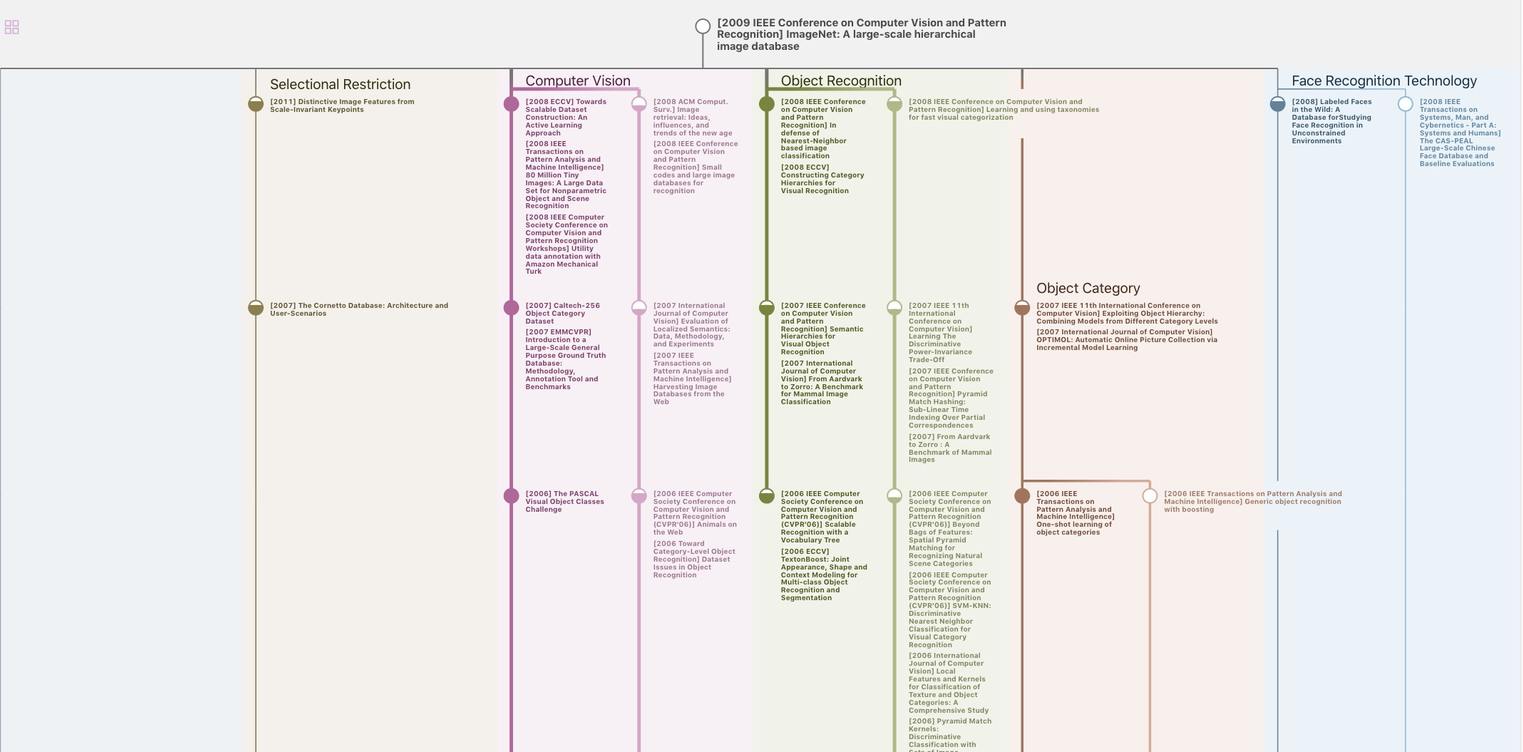
生成溯源树,研究论文发展脉络
Chat Paper
正在生成论文摘要