A High Accuracy and Adaptive Anomaly Detection Model With Dual-Domain Graph Convolutional Network for Insider Threat Detection.
IEEE Trans. Inf. Forensics Secur.(2023)
摘要
Insider threat is destructive and concealable, making addressing it a challenging task in cybersecurity. Most existing methods transform user behavior into sequential information and analyze user behavior while neglecting structural information among users, resulting in high false positives. To solve this problem, in this paper, we propose Dual-Domain Graph Convolutional Network (referred to as DD-GCN), a graph-based modularized method for high accuracy and adaptive insider threat detection. The central idea is to convert user features and structural information into heterogeneous graphs in the light of various relationships and take user behavior and relationship into account together. To this end, a weighted feature similarity mechanism is applied to balance the feature similarity of users and original linkages among them so as to generate the fused structure. Next, specific graph embeddings are extracted from the original topology structure and fused structure simultaneously, which convert behavior information into high-level representations. Furthermore, an attention mechanism is applied to learn the adaptive importance weights of the user's features in the corresponding embedding. The combination and difference constraints are proposed to enhance the learned embeddings' commonality and the ability to capture different information. Extensive experiments on two real-world datasets clearly show that our proposed DD-GCN extracts the most correlated information from structural topology and feature information substantially, and achieves improved accuracy with a clear margin.
更多查看译文
关键词
Behavioral sciences,Feature extraction,Convolutional neural networks,Topology,Adaptation models,Network topology,Couplings,Insider threat detection,anomaly detection,graph convolutional network
AI 理解论文
溯源树
样例
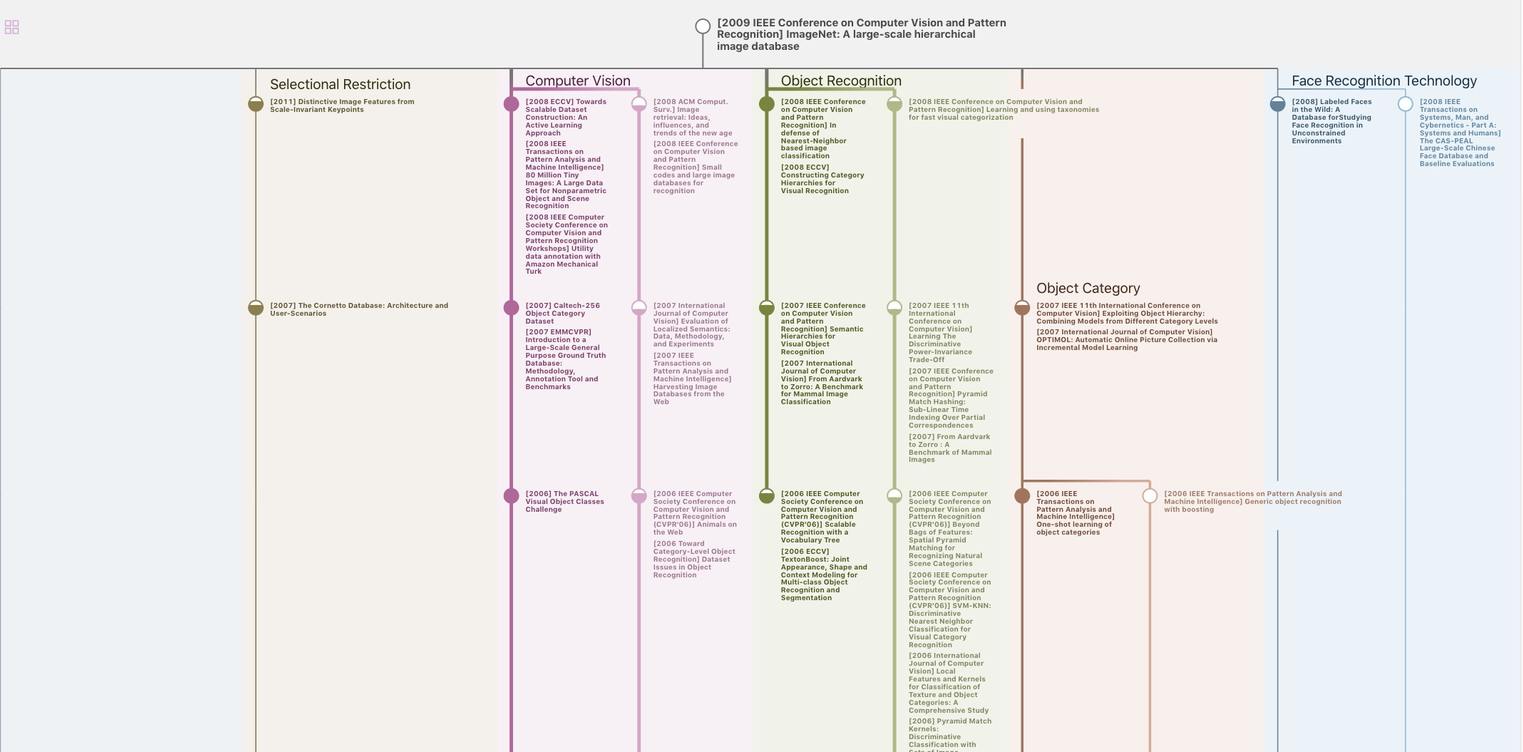
生成溯源树,研究论文发展脉络
Chat Paper
正在生成论文摘要