Nonlocal Correntropy Matrix Representation for Hyperspectral Image Classification.
IEEE Geosci. Remote. Sens. Lett.(2023)
摘要
Hyperspectral image (HSI) classification is a hot topic in the remote sensing community. However, it is challenging to fully use spatial-spectral information for HSI classification due to the high dimensionality of the data, high intraclass variability, and the limited availability of training samples. To deal with these issues, we propose a novel feature extraction method called nonlocal correntropy matrix (NLCM) representation in this letter. NLCM can characterize the spectral correlation and effectively extract discriminative features for HSI classification. We verify the effectiveness of the proposed method on two widely used datasets. The results show that NLCM performs better than the state-of-the-art methods, especially when the training set size is small. Furthermore, the experimental results also demonstrate that the proposed method outperforms compared methods significantly when the land covers are complex and with irregular distributions.
更多查看译文
关键词
Feature extraction,Training,Symmetric matrices,Hyperspectral imaging,Support vector machines,Classification algorithms,Image segmentation,hyperspectral image (HSI) classification,nonlocal correntropy matrix (NLCM)
AI 理解论文
溯源树
样例
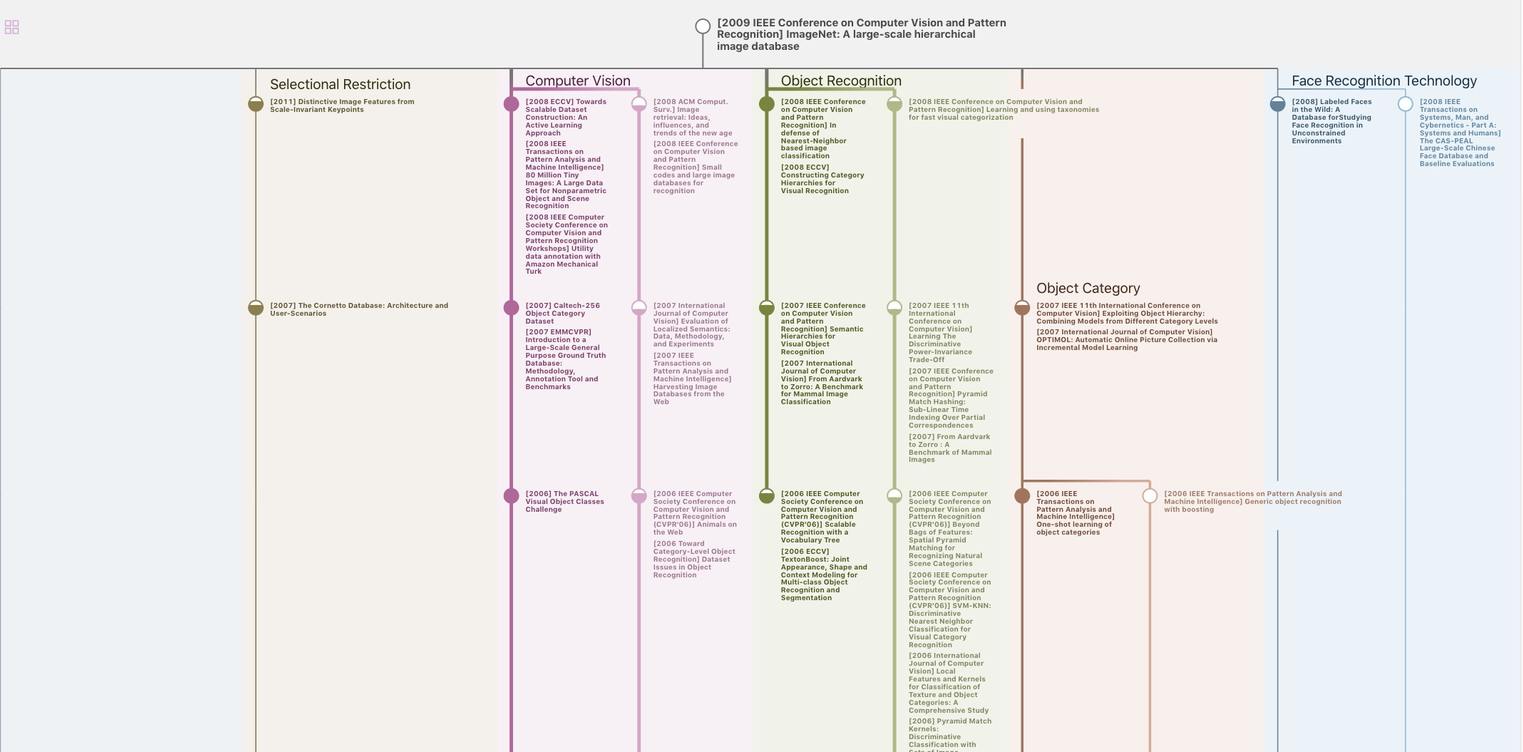
生成溯源树,研究论文发展脉络
Chat Paper
正在生成论文摘要