Comparison of the Combined Deep Learning Methods for Load Forecasting
2023 IEEE POWER & ENERGY SOCIETY INNOVATIVE SMART GRID TECHNOLOGIES CONFERENCE, ISGT(2023)
摘要
Accurate short-term electricity load forecasting is essential for reliability, stable system operation, planning, and network upgrade requirements. Atmospheric weather conditions play a crucial role in obtaining accurate electricity load forecasting. This study concentrates on multivariable combined deep-learning methods for hour-ahead load forecasting while considering weather conditions on ISO/New England (NE) datasets. Several combined models were compared and analyzed to determine which method is suitable for weather-related electricity load forecasting for the territory of the ISO/NE electricity load. Several models were examined, namely combined convolution neural network and recurrent neural network (CNNRNN), combined CNN and long short-term memory (CNNLSTM), and combined CNN and gated recurrent unit (CNNGRU), and compared their performance evaluation metrics: RMSE, MAE, MAPE.
更多查看译文
AI 理解论文
溯源树
样例
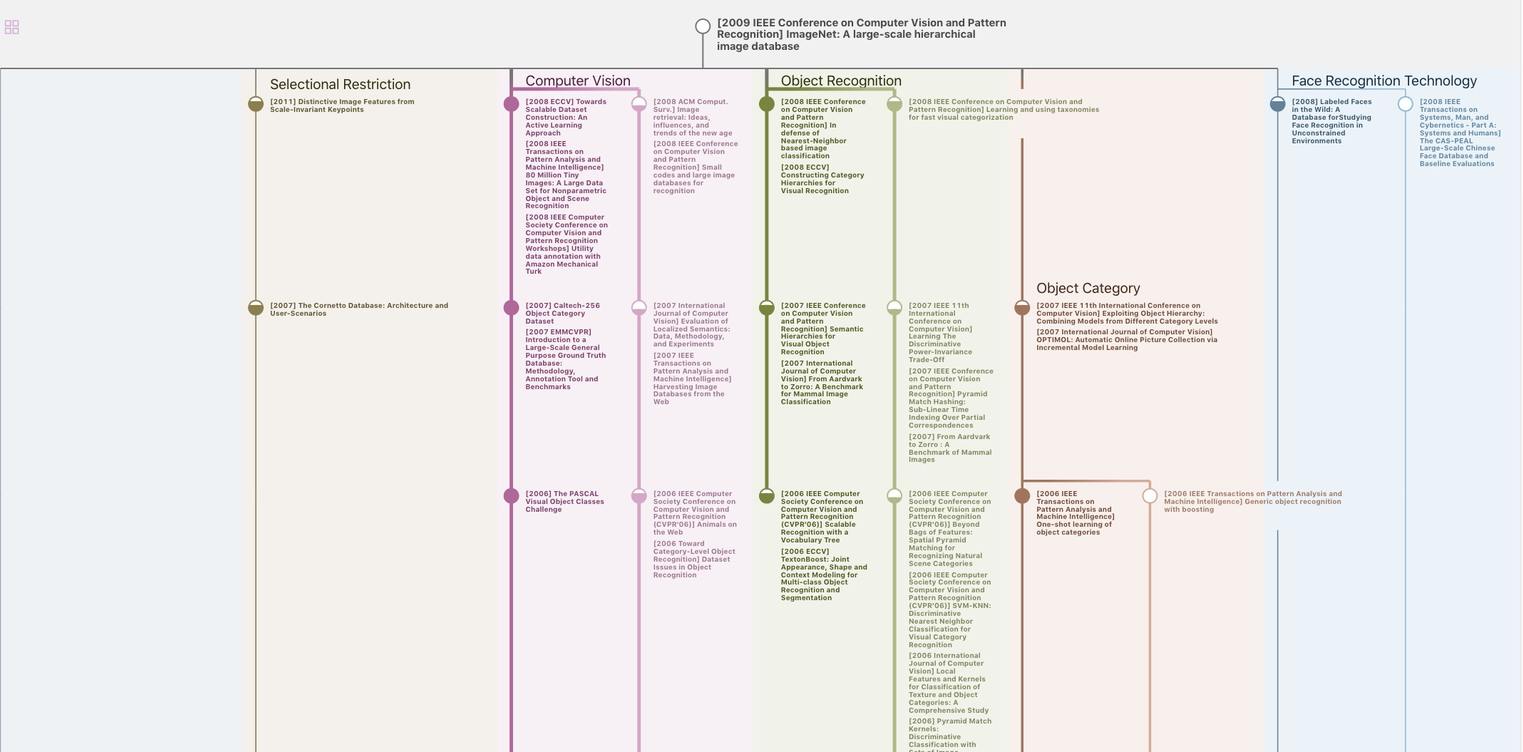
生成溯源树,研究论文发展脉络
Chat Paper
正在生成论文摘要