Machine Learning based Occupant Behavior Prediction in Smart Building to Improve Energy Efficiency
ISGT(2023)
摘要
The demand for quick and precise occupancy prediction in building management system is rapidly growing as a result of more complex Internet of Things (IoT) devices and their widespread implementation in smart building automation. This is done to save electricity while maintaining occupant's comfort. In this paper, we propose a one-layer Gated Recurrent Unit (GRU) neural network for smart building occupancy prediction. We compare its performance with that of Long Short-Term Memory (LSTM) network which is widely used in the literature. We use a data-set collected from hundreds of digital passive infrared (PIR) occupancy sensors in a large academic building in California. The experimental findings reveal that GRU outperforms the LSTM network by obtaining lower error by 1.21% and requiring less parameters of 13.57% for training. As a result, GRU can be trained 10% faster and thus is better suited for large-scale occupancy prediction tasks in emerging smart buildings.
更多查看译文
关键词
Deep neural network,Energy consumption,Internet of things,GRU,LSTM,Occupancy prediction,Smart,building
AI 理解论文
溯源树
样例
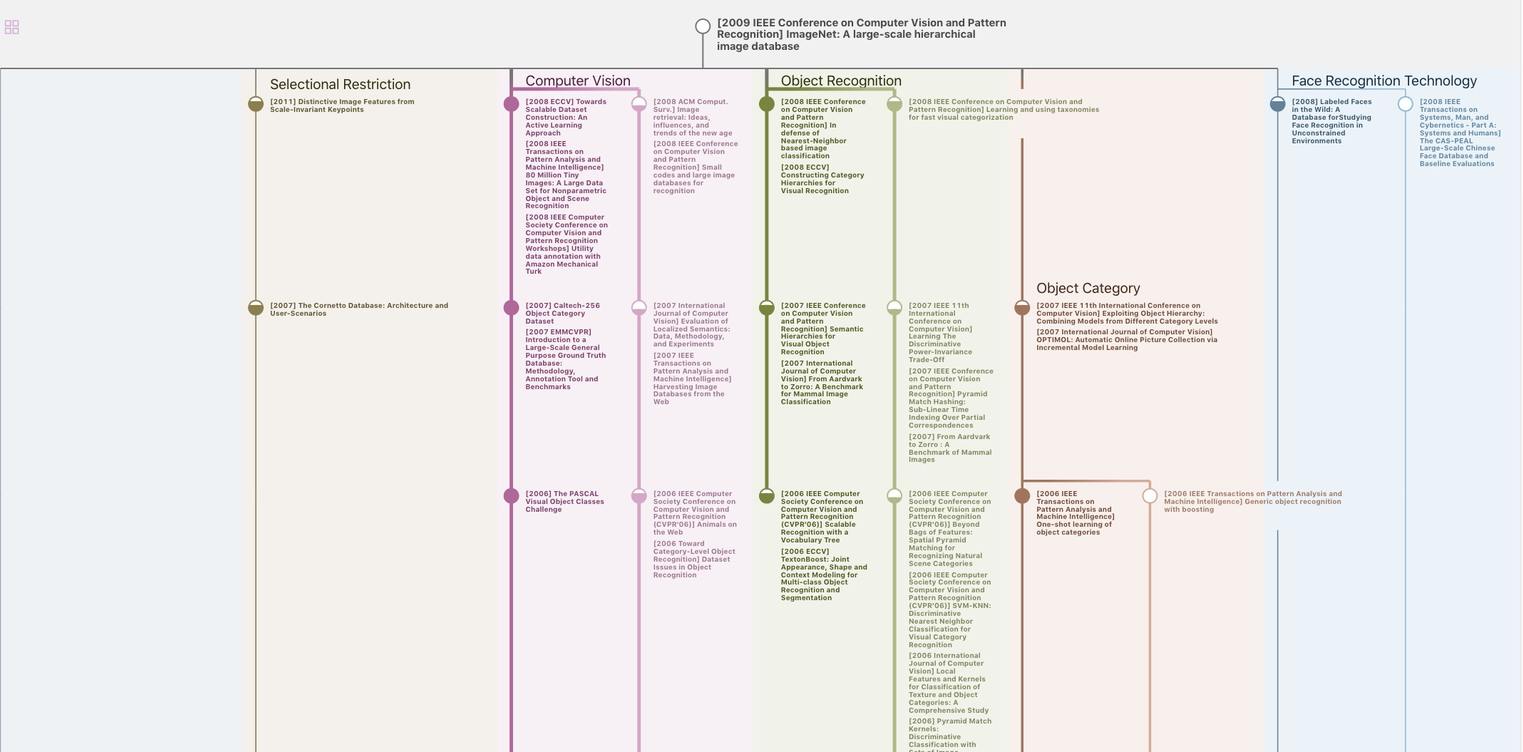
生成溯源树,研究论文发展脉络
Chat Paper
正在生成论文摘要