DP-AMI-FL: Secure Framework for Machine Learning-based AMI Applications.
ISGT(2023)
摘要
Machine Learning (ML) algorithms have shown quite promising applications in smart meter data analytics enabling intelligent energy management systems for the Advanced Metering Infrastructure (AMI). One of the major challenges in developing ML applications for the AMI is to preserve user privacy while allowing active end-users participation. This paper addresses this challenge and proposes Differential Privacy-enabled AMI with Federated Learning (DP-AMI-FL), framework for ML-based applications in the AMI. This framework provides two layers of privacy protection: first, it keeps the raw data of consumers hosting ML applications at edge devices (smart meters) with Federated Learning (FL), and second, it obfuscates the ML models using Differential Privacy (DP) to avoid privacy leakage threats on the models posed by various inference attacks. The framework is evaluated by analyzing its performance on a use case aimed to improve Short-Term Load Forecasting (STLF) for residential consumers having smart meters and home energy management systems. Extensive experiments demonstrate that the framework when used with Long Short-Term Memory (LSTM) recurrent neural network models, achieves high forecasting accuracy while preserving users data privacy.
更多查看译文
关键词
Smart meter data analytics,federated machine learning,short-term load forecasting,user privacy,advanced metering infrastructure
AI 理解论文
溯源树
样例
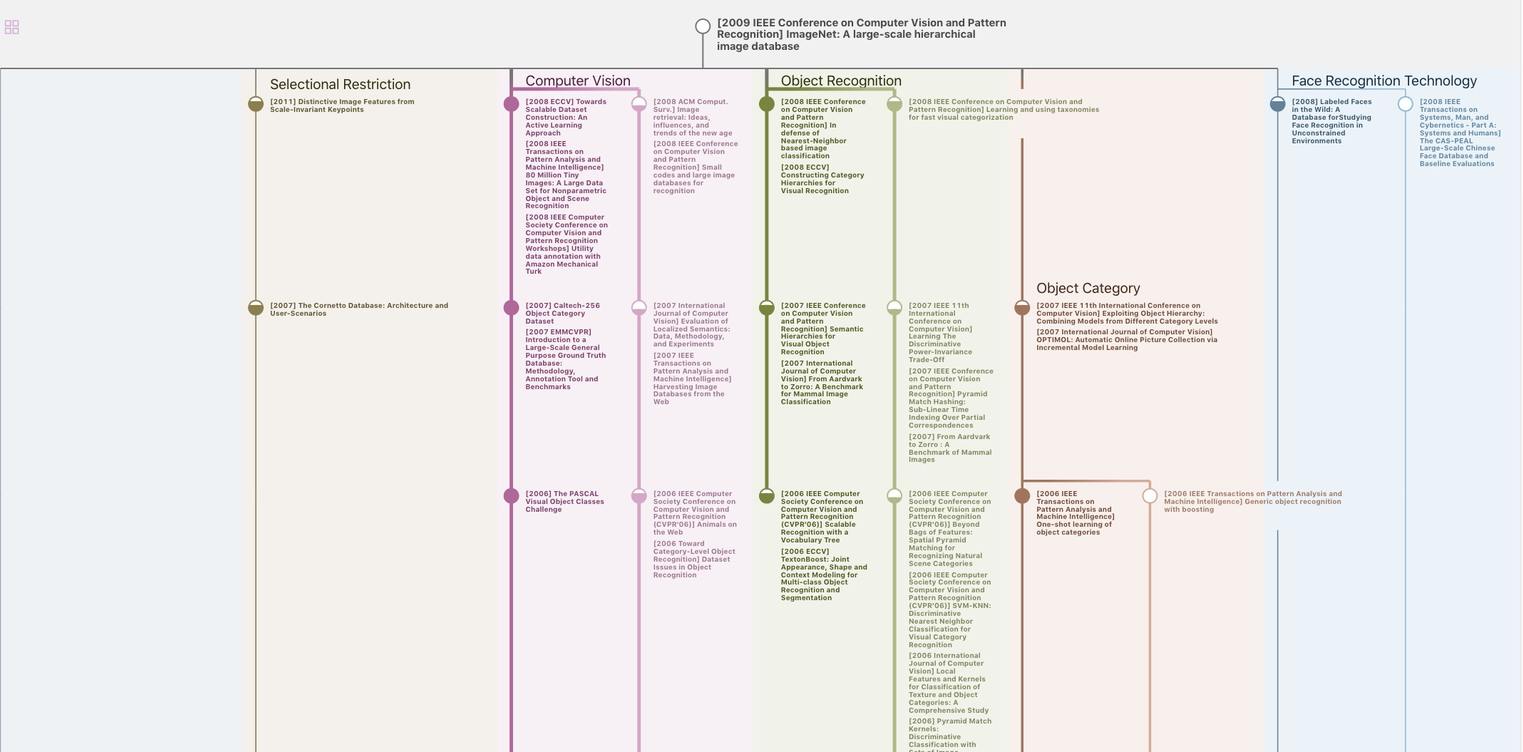
生成溯源树,研究论文发展脉络
Chat Paper
正在生成论文摘要