Semantic Representation Based on AMR Graph for Document Level Question Generation
MLNLP(2022)
摘要
Question Generation (QG) is the task of generating questions from reference sentences and answers specified in the sentences. Intuitively, as one type of semantic representations, Abtract Meaning Representation (AMR) can be helpful for question generation by enforcing meaning preservation and handling data sparsity due to being abstracted from syntactic representations. However, little work has been done to leverage rich semantics in AMR for question generation. Therefore, we design AMR Question Generation (AMRQG) model to construct document-level AMR graphs from sentencelevel AMR graphs. The model solves the alignment issue between nodes of AMR graph and words in sequence text at graph-level and node-level. To our knowledge, we are the first to leverage AMR representation into question generation for document-level input. Automatic evaluation results demonstrate that incorporating AMR as additional knowledge can significantly improve a sequence-to-sequence natural question generation model and show our model's superiority.
更多查看译文
关键词
Abstract Meaning Representation, Graph Neural Network
AI 理解论文
溯源树
样例
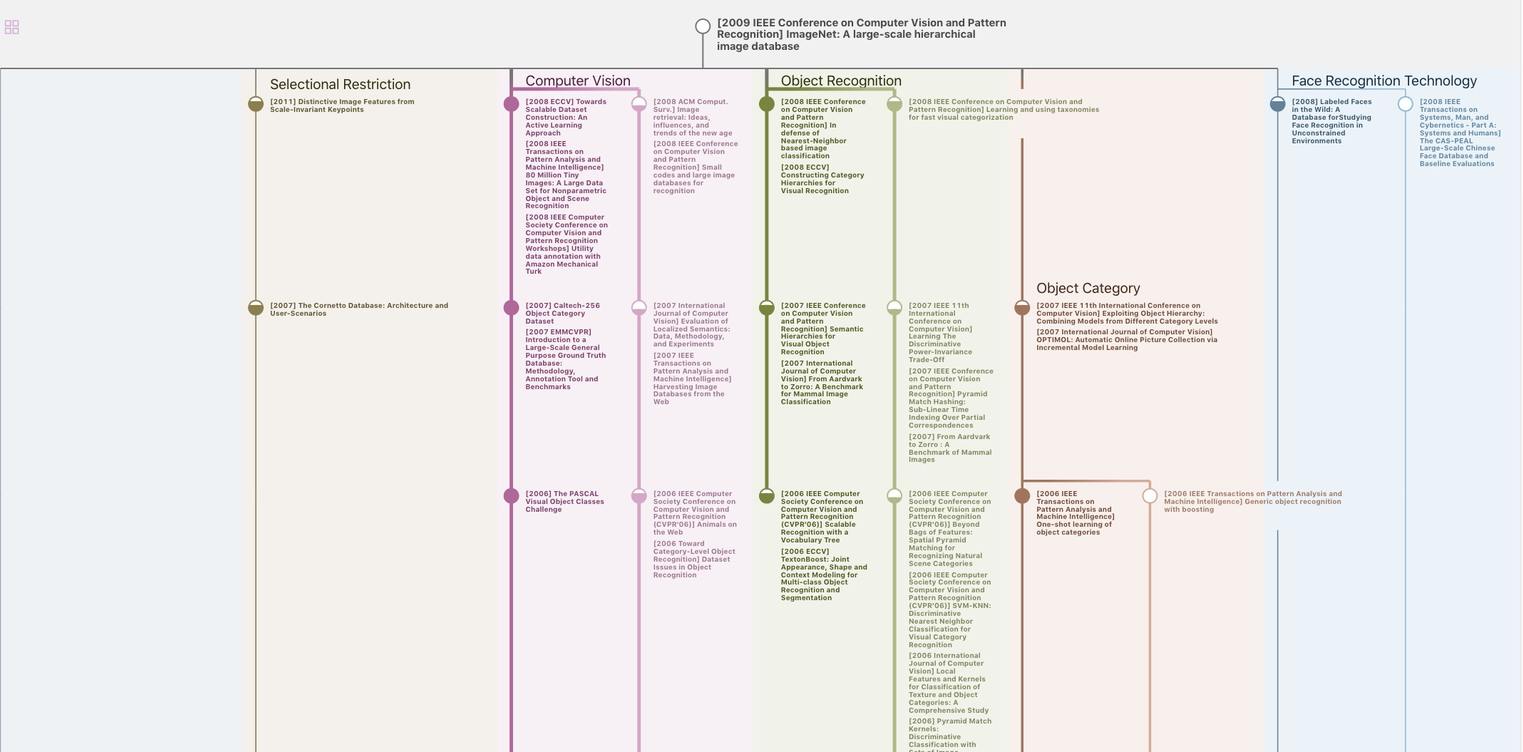
生成溯源树,研究论文发展脉络
Chat Paper
正在生成论文摘要