Hierarchical Knowledge Representation of Complex Tasks Based on Dynamic Motion Primitives.
ICCSIP(2022)
摘要
Current service robots without learning ability are not qualified for many complex tasks. Therefore, it is very significant to decompose the complex task into repeatable execution unit. In this paper, we propose a complex task representation method based on dynamic motion primitives, and use hierarchical knowledge graph to represent the analytic results of complex tasks. To realize the execution of complex robot manipulation tasks, we decompose the semantic tasks into the minimum motion units that can be executed by the robot and combine the multi-modal information: posture, force and robot joint parameters, obtained by the sensors. We use the knowledge graph to record the end-effector required by the robot to perform different tasks and make appropriate selection of end-effector according to different needs. Finally, Taking the long sequence complex task of service scene as an example, we use UR5 robot to verify the effectiveness and feasibility of this design.
更多查看译文
关键词
Robot manipulation, Knowledge expression, Knowledge graph, Motion primitives
AI 理解论文
溯源树
样例
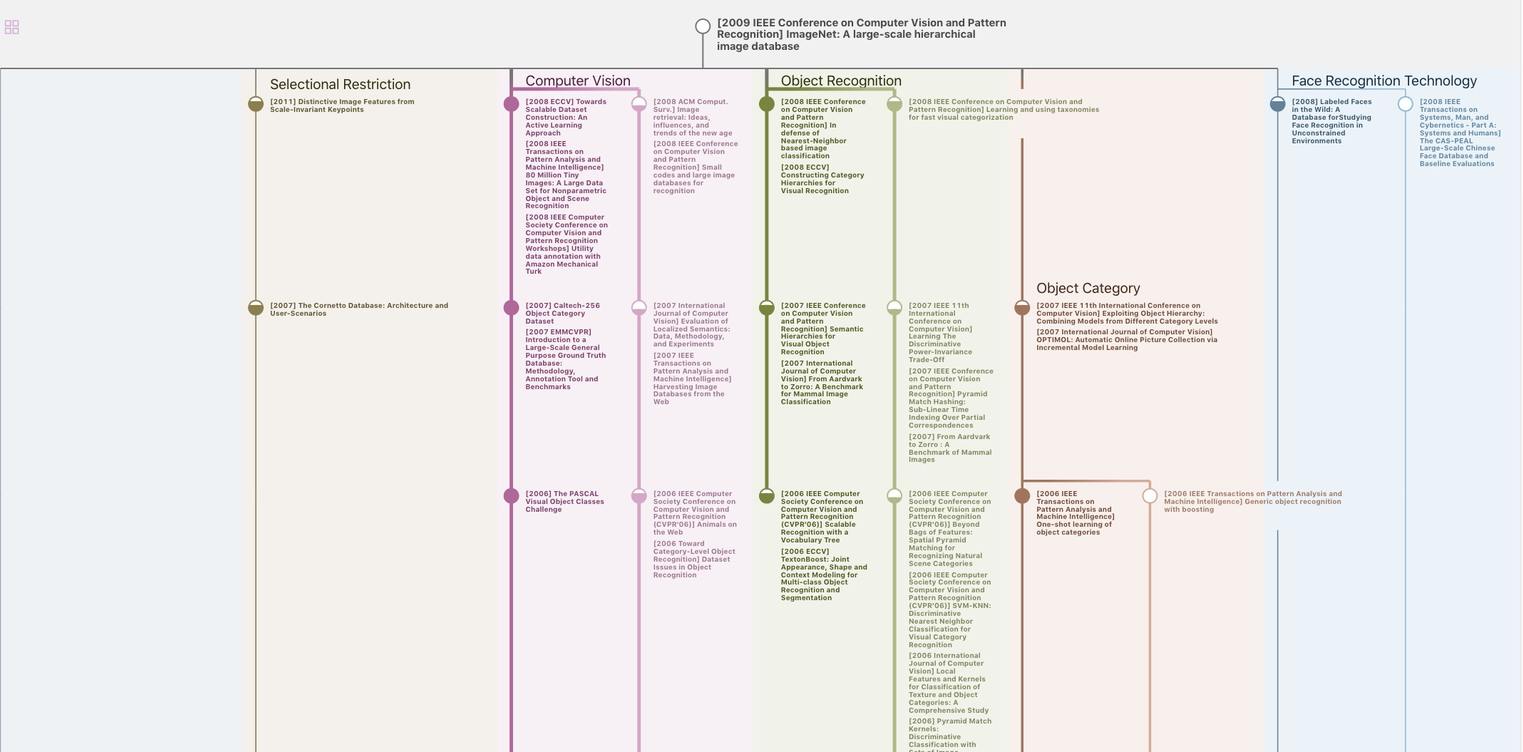
生成溯源树,研究论文发展脉络
Chat Paper
正在生成论文摘要