A Cross-patient SEEG Epileptic Signal Detection Method Based on Adaptive Feature Fusion of Brain Network Features and Single-Channel Features
ICBBE(2022)
摘要
Epilepsy is a common neurological disorder caused by the abnormal discharge of brain neurons. The automatic detection of epileptic signals (ES) is of great significance for the clinical diagnosis of epilepsy. However, there are still problems such as imperfect feature extraction, ignoring channel correlation, and unbalanced data distribution. Therefore, in this paper, we propose an ES detection method based on adaptive feature fusion (AFF) of brain network features (BNF) and single-channel features (SCF). First, two types of features, BNF and SCF, are extracted. The in-degree of each channel calculated from brain functional connectivity based on Pearson correlation is used as BNF. A multi-temporal resolution convolutional neural network (MTRCNN) extracts low-frequency and high-frequency features from single-channel stereo electroencephalography (SEEG) data as SCF. In the feature extraction process, cross-norm and self-norm (CNSN) reduce individual differences. Second, AFF automatically adjusts the fused features, aiming to combine BNF with SCF more effectively. Finally, the weighted loss function considers both the proportion and discriminability of positive and negative samples to reduce imbalanced data distribution. In this paper, we investigated interictal SEEG data from 7 patients with refractory focal hippocampal sclerosing epilepsy and performed cross-patient cross-validation. The results show that our method outperforms the state-of-the-art in all 5 metrics. Our ES automatic detection method provides an objective reference for epilepsy diagnosis, thereby reducing the workload of neurologists.
更多查看译文
关键词
Epilepsy, Epileptic signal detection, Brain network, SEEG, Feature fusion
AI 理解论文
溯源树
样例
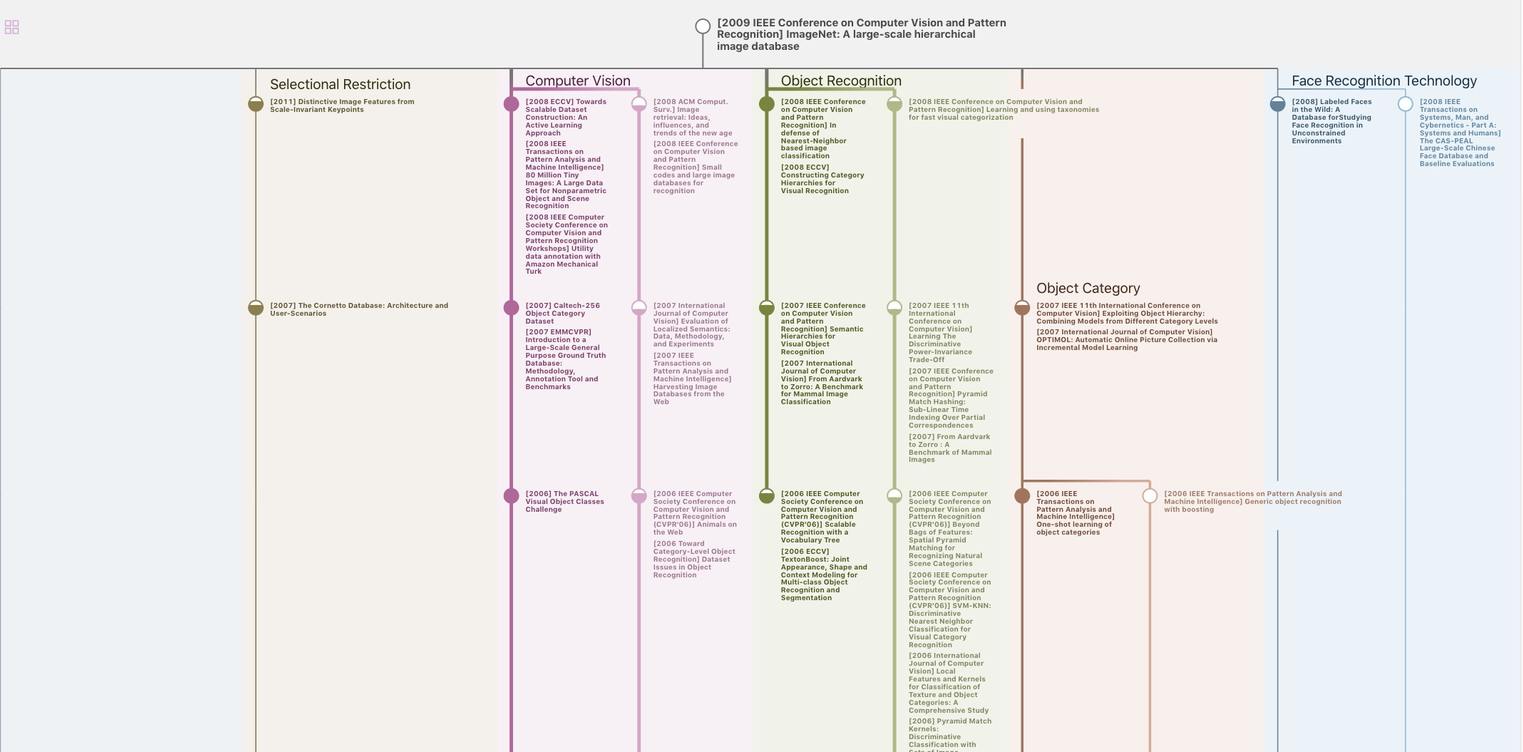
生成溯源树,研究论文发展脉络
Chat Paper
正在生成论文摘要