An unobtrusive upper-limb activity recognition system based on deep neural network fusion for stroke survivors
ICBBE(2022)
摘要
Stroke is a cerebrovascular disease that may cause long-term paralysis. Stroke survivors can recover more quickly with personalized treatment, which often requires the identification and evaluation of daily activities. Most of the existing methods for stroke activity recognition use wearable devices to collect motion and/or electrophysiology signals. However, as most survivors are elderly, the wearing process and operation methods are inevitably inconvenient for them. In this paper, we proposed an unobtrusive upper-limb movement recognition system for stroke survivors based on model fusion via combining three deep neural networks. Specifically, we recruited 16 stroke survivors with different impairment levels. Considering fine and dexterous movements of the upper limbs and hands take an important part in our daily life, fine-grained hand activities are more difficult to recognize. We conducted seventeen hand gesture recognition using video data collected by an Azure Kinect sensor. We compared the performance of three state-of-the-art deep neural networks, namely TSN, I3D, and Slowfast. Moreover, we fused the three models using soft voting. The top1 mean accuracy of our fusion model is 93.45% on our dataset. With our method, it is expected to assist rehabilitation physicians, to formulate the corresponding treatment plan, and make better-personalized treatment for stroke survivors.
更多查看译文
关键词
Stroke, Deep neural network, Hand gesture recognition
AI 理解论文
溯源树
样例
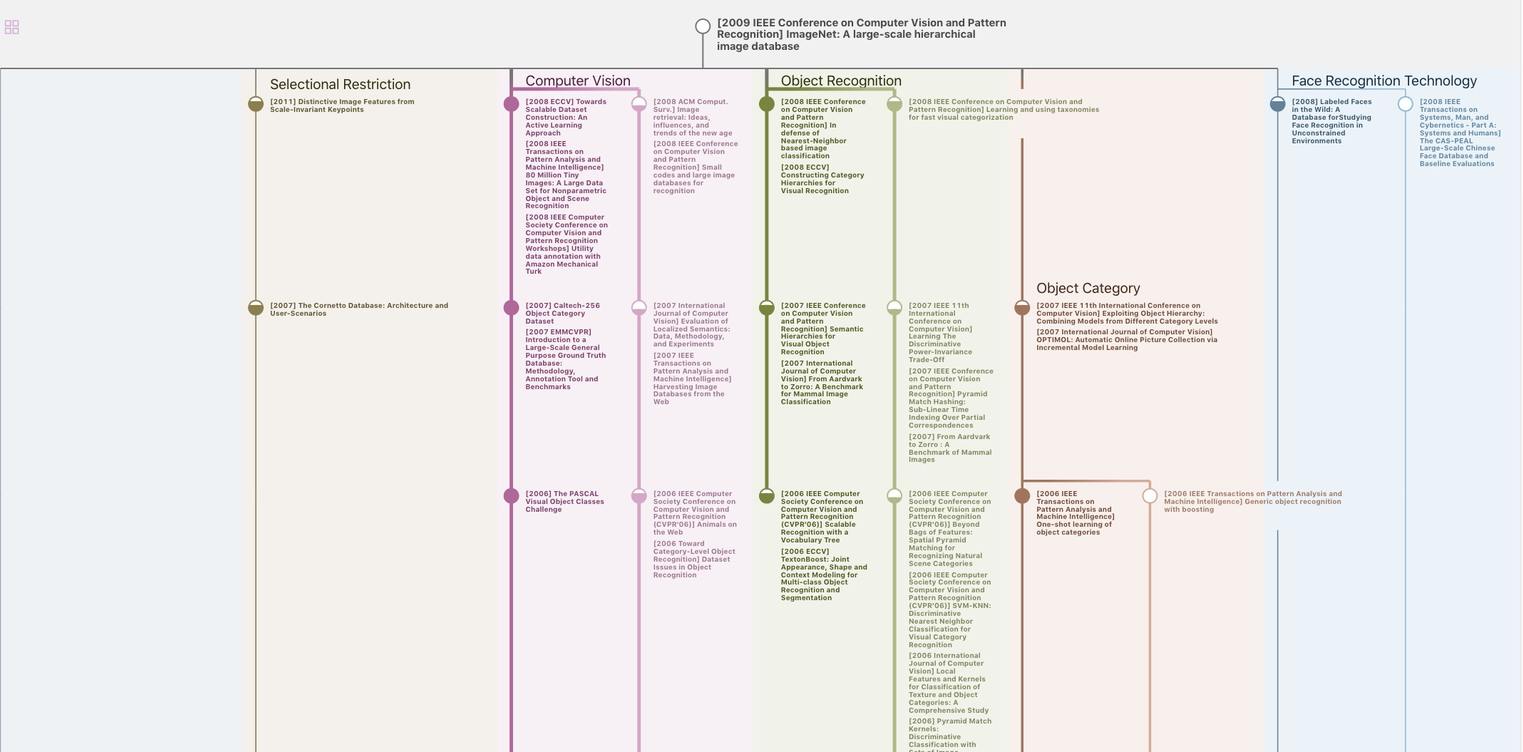
生成溯源树,研究论文发展脉络
Chat Paper
正在生成论文摘要