A Comparison of Different U-Net Models for Segmentation of Overlapping Organoids
ICBBE(2022)
摘要
The interest in automatically analyzing biomedical images increased in the past years, as an accurate localization and segmentation of organoids can help with the early detection of malignancies and predict diseases, such as cancer. The morphometric appearances of these images and the high level of overlapping in the organoids make the segmentation task challenging. This paper studies a simple U-Net and also proposes a double U-Net model with a shared encoder and two decoders, one for binary segmentation of the mask and one for the multi-class segmentation of overlaps. A significant addition to the U-Net is the residual-atrous skip connections, which reduce the semantic gap between the encoder and the decoder. The issue of high imbalance between the classes is addressed using a combination between Focal Loss and Focal Tversky Loss, which significantly improved the performance of the model. Ten networks were trained on more than 20,000 crop images with overlapping and non-overlapping organoids and obtained promising results. When tested on 88 new images, the final models achieved an F1 score of 0.83 for the mask channel and 0.43 for the overlapping channel. The Jaccard Index was 0.72 for the mask and 0.34 for the overlap. To the authors' knowledge, there exists no work in the literature that explores the detection and segmentation of overlapping objects in biomedical images, especially segmenting overlapping organoids, which also shows the novelty of this work.
更多查看译文
关键词
Deep learning, Segmentation, Imbalanced classes, Organoids
AI 理解论文
溯源树
样例
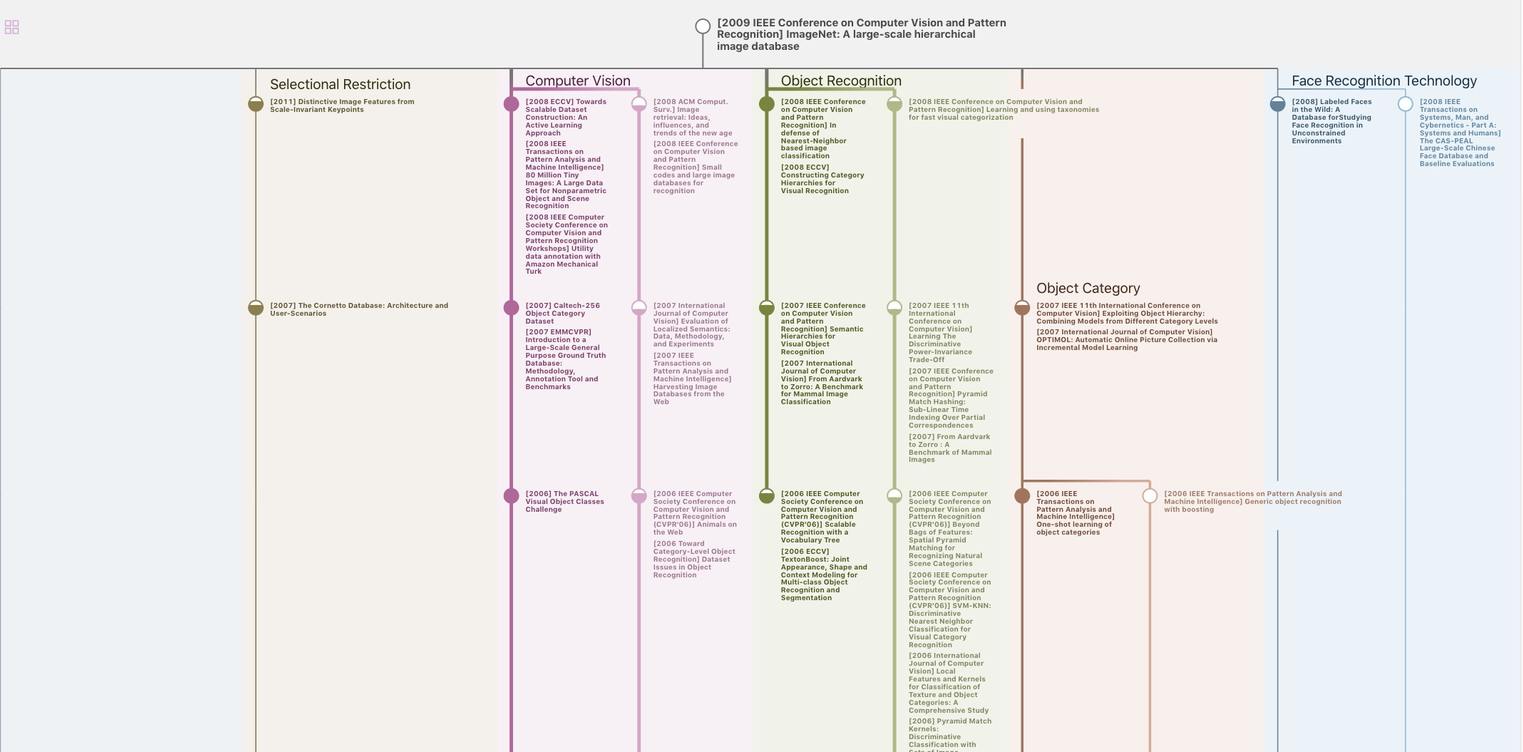
生成溯源树,研究论文发展脉络
Chat Paper
正在生成论文摘要