Real-time plume tracking using transfer learning approach.
Comput. Chem. Eng.(2023)
摘要
Deep learning has been used to track the real-time flammable plume of natural gas. However, a large volume of high-fidelity data is required to train the deep learning model for sufficient accuracy in congested industrial environments, which can be computationally prohibitive. This study proposes a transfer learning-based variablefidelity approach for real-time plume tracking. A Gaussian dispersion model was applied to efficiently generate a large volume of low-fidelity data, which is then used to pre-train the deep learning model. A limited number of high-fidelity simulations were conducted by solving the Navier-Stokes equation to fine-tune the pre-trained model. A case study demonstrated our proposed approach could reduce high-fidelity computations by 72% while ensuring prediction accuracy with R2=0.96 for released plume area estimation in congested chemical facilities. Optimal number of frozen layers, learning rate and the number of high-fidelity simulations required were determined to ensure adequate efficiency for this approach. This study provides an efficient alternative to improve the generalization of deep learning for real-time plume area estimation for large-scale congested chemical plants.
更多查看译文
关键词
Natural gas release,Flammable area prediction,Variable -fidelity modeling,Transfer learning,Deep learning,Digital twin for emergency management
AI 理解论文
溯源树
样例
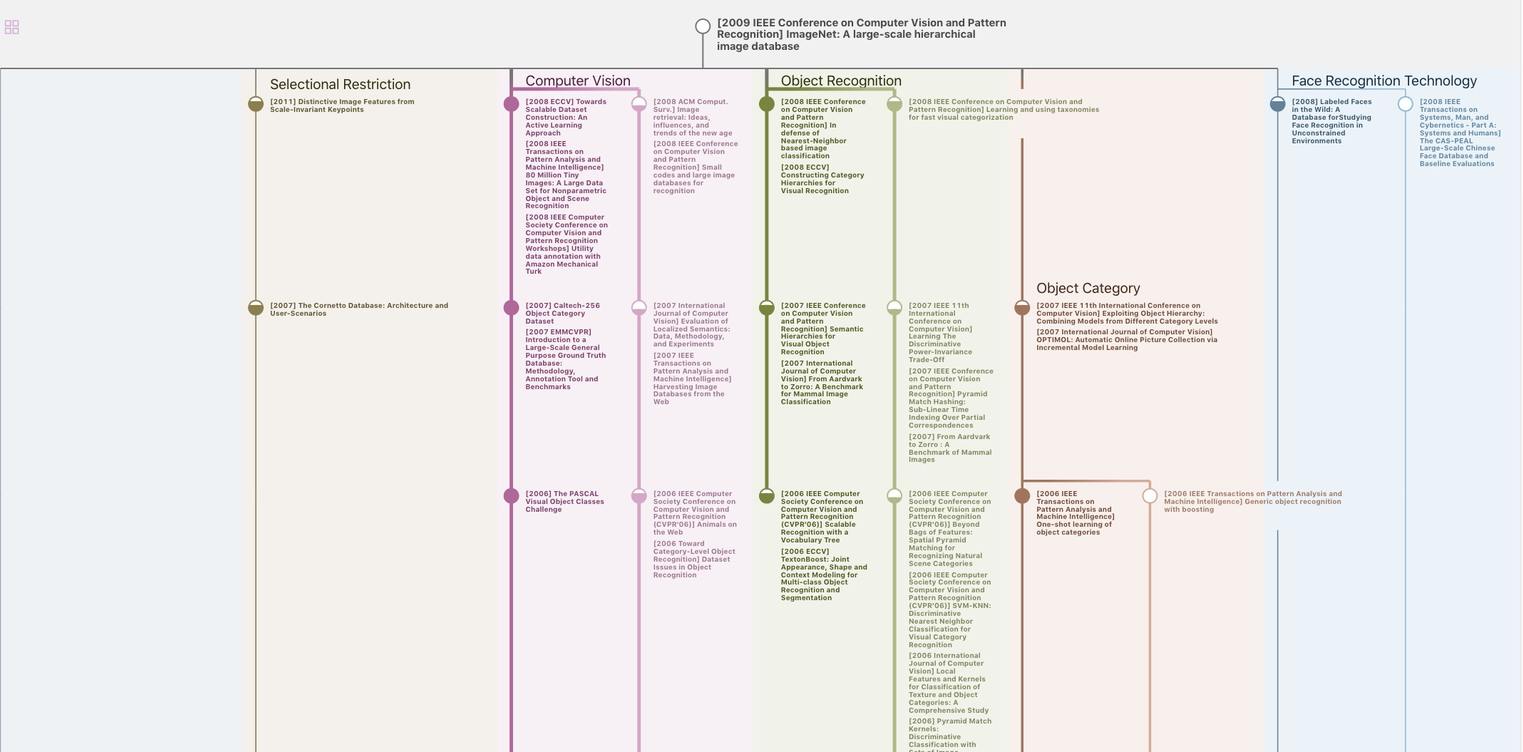
生成溯源树,研究论文发展脉络
Chat Paper
正在生成论文摘要